Switch-GPT: An Effective Method for Constrained Text Generation under Few-Shot Settings (Student Abstract).
AAAI Conference on Artificial Intelligence(2022)
摘要
In real-world applications of natural language generation, target sentences are often required to satisfy some lexical constraints. However, the success of most neural-based models relies heavily on data, which is infeasible for data-scarce new domains. In this work, we present FewShotAmazon, the first benchmark for the task of Constrained Text Generation under few-shot settings on multiple domains. Further, we propose the Switch-GPT model, in which we utilize the strong language modeling capacity of GPT-2 to generate fluent and well-formulated sentences, while using a light attention module to decide which constraint to attend to at each step. Experiments show that the proposed Switch-GPT model is effective and remarkably outperforms the baselines. Codes will be available at https://github.com/chang-github-00/Switch-GPT.
更多查看译文
关键词
Few-shot Learning,GPT,NLP,Pre-trained Language Model,Text Generation
AI 理解论文
溯源树
样例
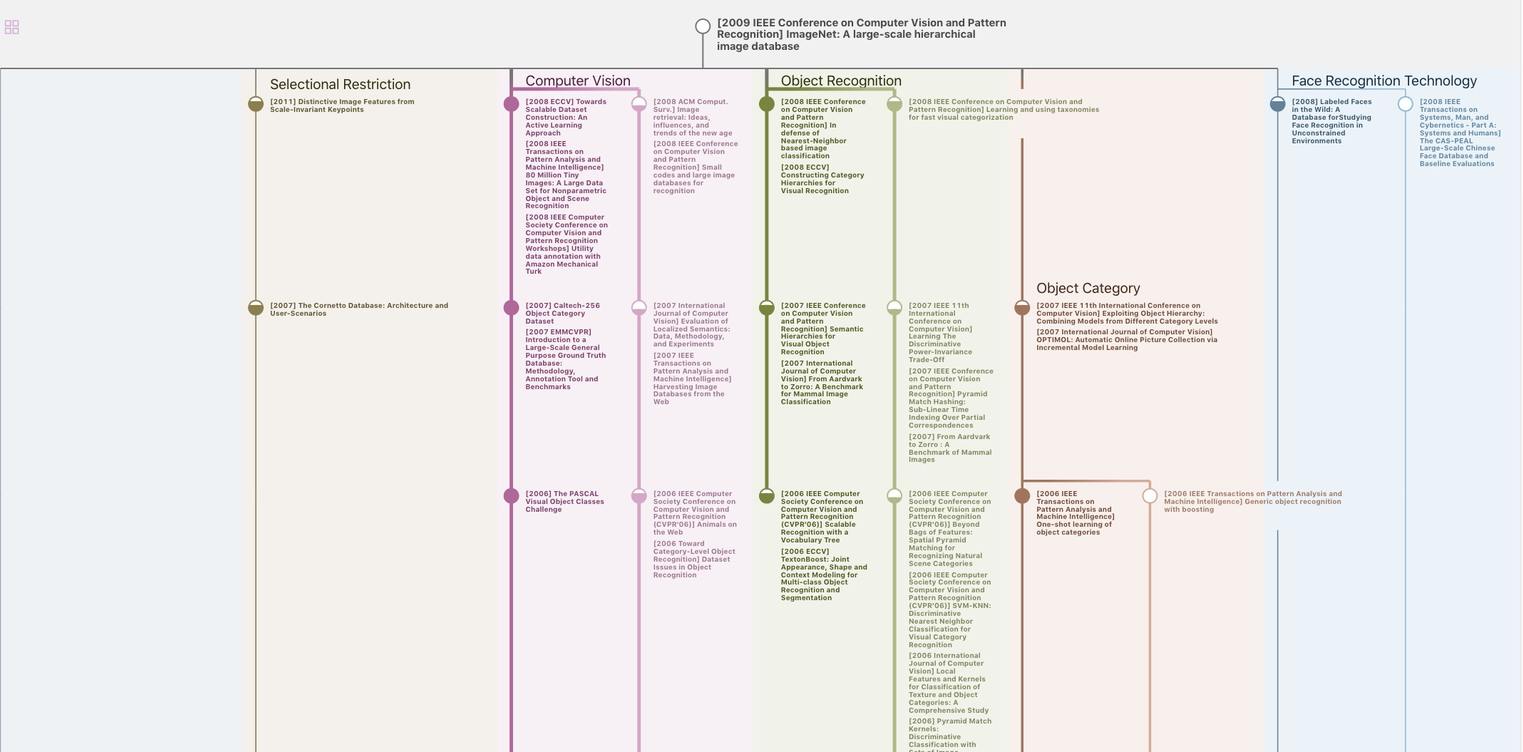
生成溯源树,研究论文发展脉络
Chat Paper
正在生成论文摘要