Learning Space-Time Crop Yield Patterns with Zigzag Persistence-Based LSTM: Toward More Reliable Digital Agriculture Insurance.
AAAI Conference on Artificial Intelligence(2022)
摘要
More than US$ 27 billion is estimated to have been paid-out in farm support in USA alone since 1991 in response to climate change impacts on agriculture, with costs likely continuing to rise. With the wider adoption of precision agriculture - an agriculture management strategy that involves gathering, processing and analyzing temporal, spatial and individual data - in both developed and developing countries, there is an increasing opportunity to harness accumulating, shareable, big data using artificial intelligence (AI) methods, collected from weather stations, field sensor networks, Internet-of-Things devices, unmanned aerial vehicles, and earth observational satellites. This requires smart algorithms tailored to agricultural data types, integrated into digital solutions that are viable, flexible, and scalable for wide deployment for a wide variety of agricultural users and decision-makers. We discuss a novel AI approach that addresses the real-world problem of developing a viable solution for reliably, timely, and cost-effectively forecasting crop status across large agricultural regions using Earth observational information in near-real-time. Our approach is based on extracting time-conditioned topological features which characterize complex spatio-temporal dependencies between crop production regions and integrating such topological signatures into Long Short Term Memory (LSTM). We discuss utility and limitations of the resulting zigzag persistence-based LSTM (ZZTop-LSTM) as a new tool for developing more informed crop insurance rate-making and accurate tracking of changing risk exposures and vulnerabilities within insurance risk areas.
更多查看译文
关键词
Digital Agricultural Insurance,LSTM,Time-aware Topological Descriptors,Zigzag Persistence,Space-time Modeling
AI 理解论文
溯源树
样例
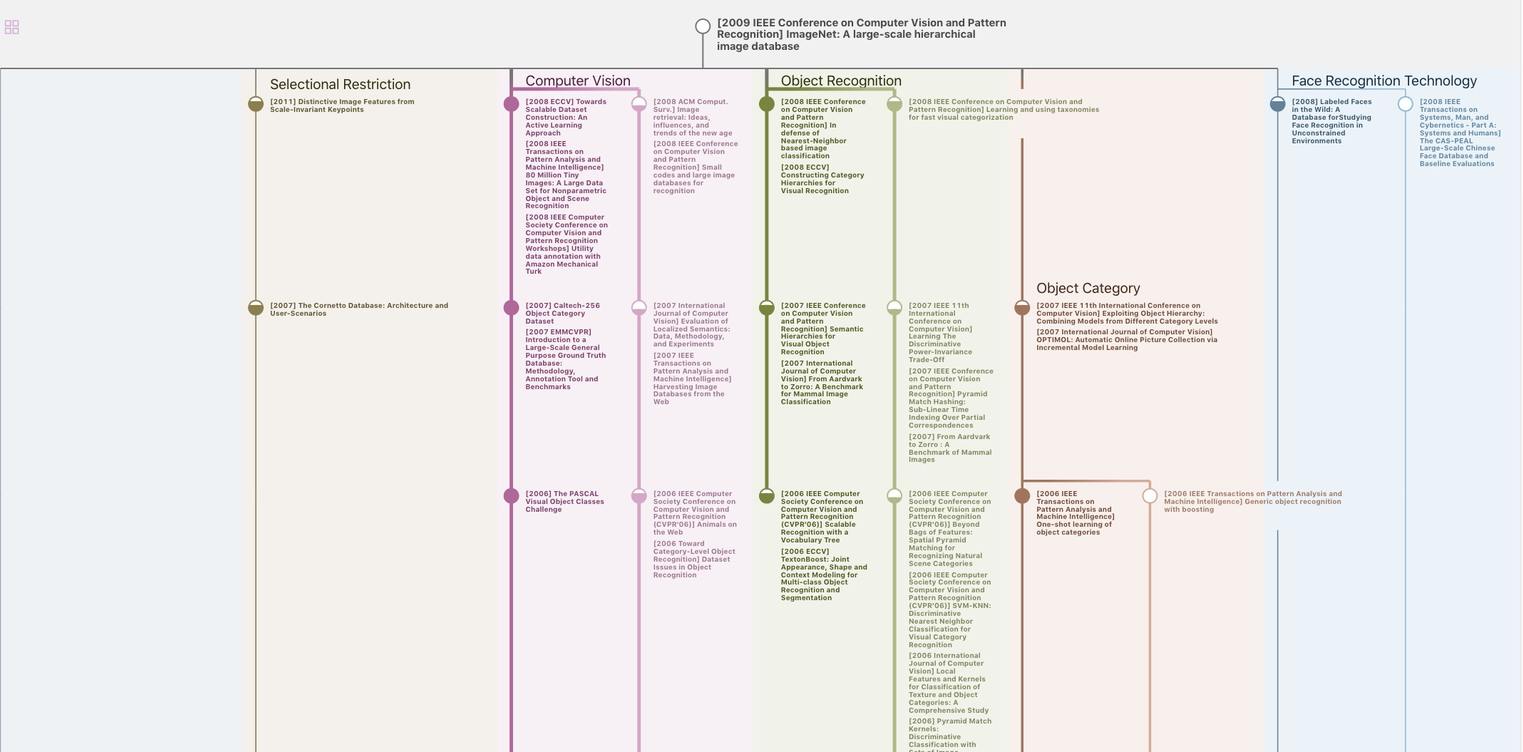
生成溯源树,研究论文发展脉络
Chat Paper
正在生成论文摘要