Span-Based Semantic Role Labeling with Argument Pruning and Second-Order Inference.
AAAI Conference on Artificial Intelligence(2022)
摘要
We study graph-based approaches to span-based semantic role labeling. This task is difficult due to the need to enumerate all possible predicate-argument pairs and the high degree of imbalance between positive and negative samples. Based on these difficulties, high-order inference that considers interactions between multiple arguments and predicates is often deemed beneficial but has rarely been used in span-based semantic role labeling. Because even for second-order inference, there are already O(n^5) parts for a sentence of length n, and exact high-order inference is intractable. In this paper, we propose a framework consisting of two networks: a predicate-agnostic argument pruning network that reduces the number of candidate arguments to O(n), and a semantic role labeling network with an optional second-order decoder that is unfolded from an approximate inference algorithm. Our experiments show that our framework achieves significant and consistent improvement over previous approaches.
更多查看译文
关键词
Speech & Natural Language Processing (SNLP)
AI 理解论文
溯源树
样例
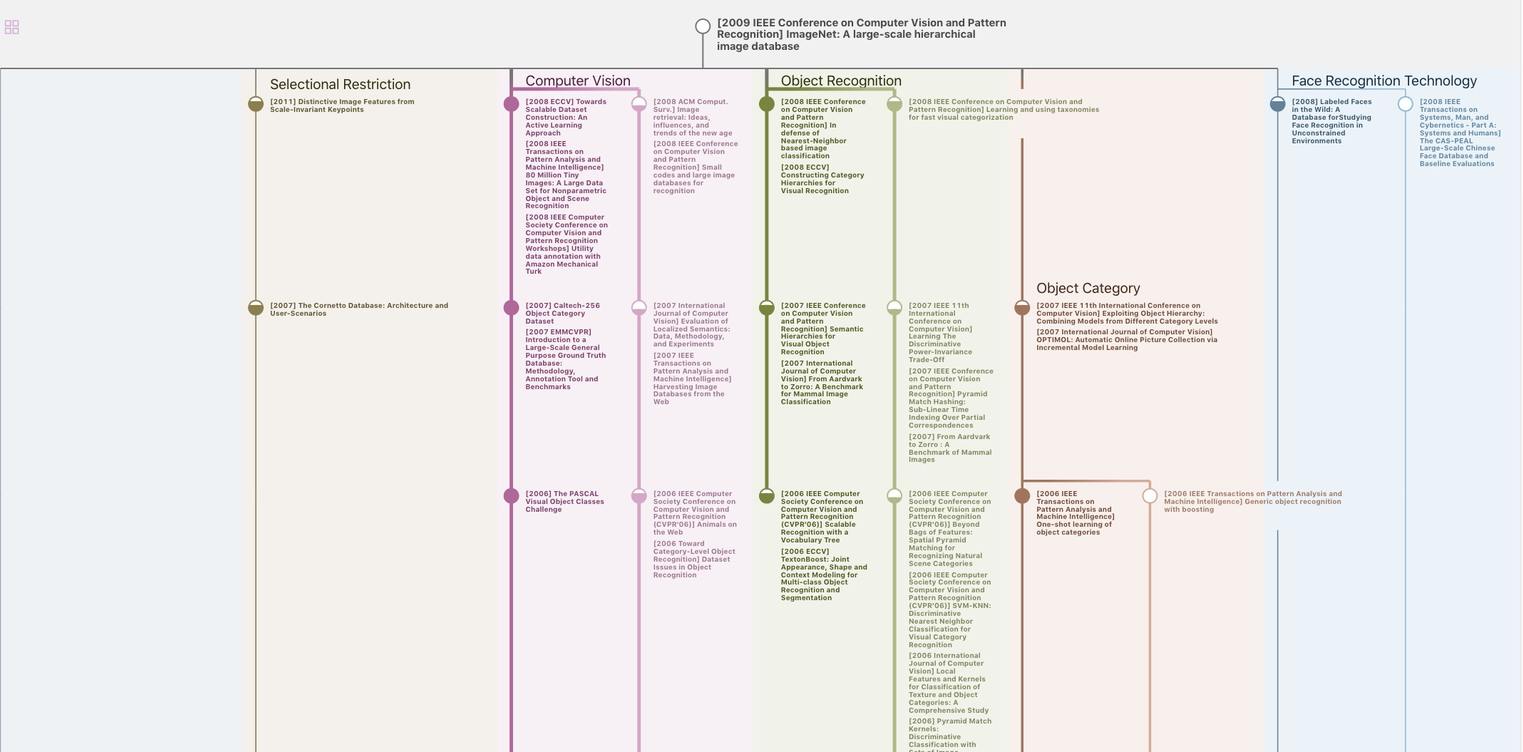
生成溯源树,研究论文发展脉络
Chat Paper
正在生成论文摘要