Unpaired Multi-Domain Stain Transfer for Kidney Histopathological Images.
AAAI Conference on Artificial Intelligence(2022)
摘要
As an essential step in the pathological diagnosis, histochemical staining can show specific tissue structure information and, consequently, assist pathologists in making accurate diagnoses. Clinical kidney histopathological analyses usually employ more than one type of staining: H&E, MAS, PAS, PASM, etc. However, due to the interference of colors among multiple stains, it is not easy to perform multiple staining simultaneously on one biological tissue. To address this problem, we propose a network based on unpaired training data to virtually generate multiple types of staining from one staining. Our method can preserve the content of input images while transferring them to multiple target styles accurately. To efficiently control the direction of stain transfer, we propose a style guided normalization (SGN). Furthermore, a multiple style encoding (MSE) is devised to represent the relationship among different staining styles dynamically. An improved one-hot label is also proposed to enhance the generalization ability and extendibility of our method. Vast experiments have demonstrated that our model can achieve superior performance on a tiny dataset. The results exhibit not only good performance but also great visualization and interpretability. Especially, our method also achieves satisfactory results over cross-tissue, cross-staining as well as cross-task. We believe that our method will significantly influence clinical stain transfer and reduce the workload greatly for pathologists. Our code and Supplementary materials are available at https://github.com/linyiyang98/UMDST.
更多查看译文
关键词
Computer Vision (CV)
AI 理解论文
溯源树
样例
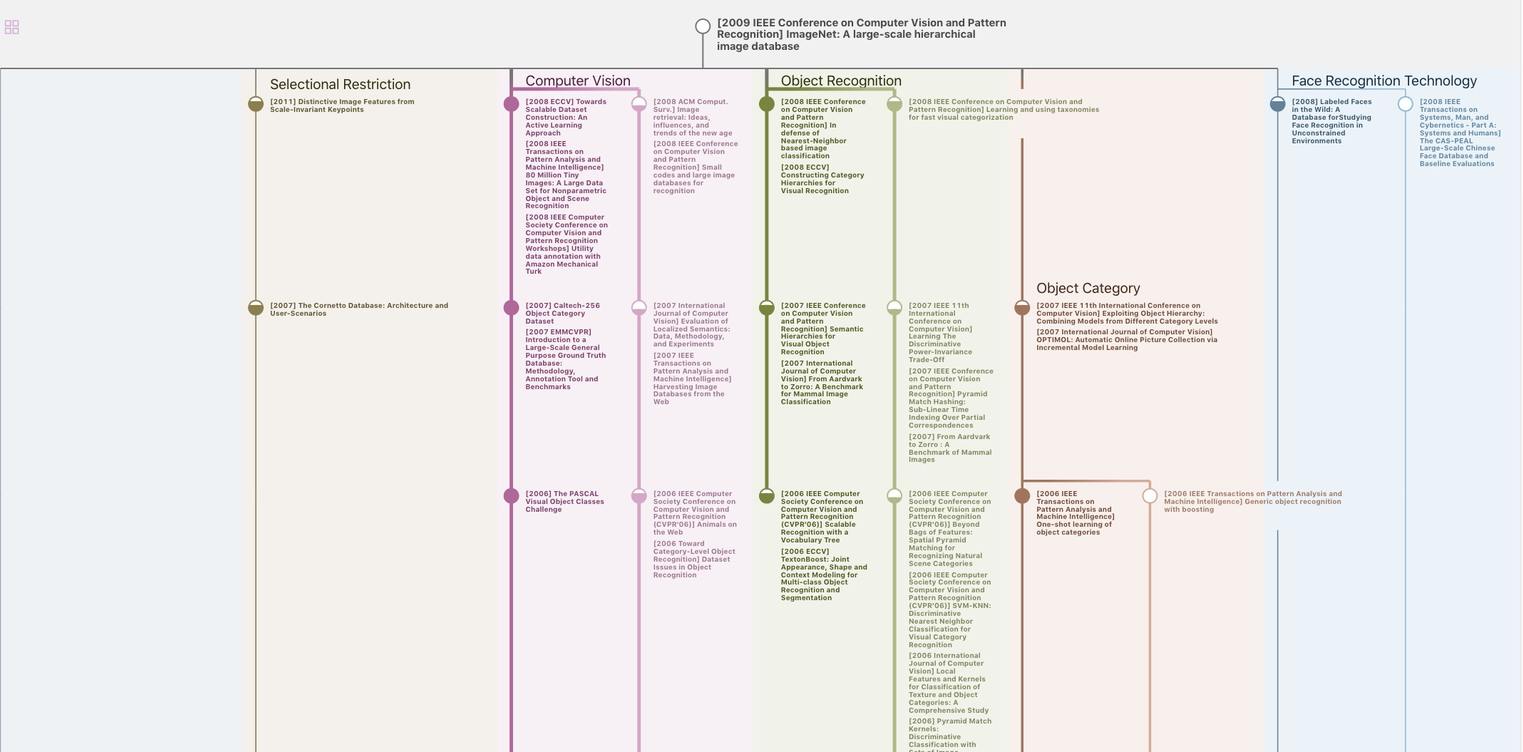
生成溯源树,研究论文发展脉络
Chat Paper
正在生成论文摘要