OA-FSUI2IT: A Novel Few-Shot Cross Domain Object Detection Framework with Object-Aware Few-Shot Unsupervised Image-to-Image Translation.
AAAI Conference on Artificial Intelligence(2022)
摘要
Unsupervised image-to-image (UI2I) translation methods aim to learn a mapping between different visual domains with well-preserved content and consistent structure. It has been proven that the generated images are quite useful for enhancing the performance of computer vision tasks like object detection in a different domain with distribution discrepancies. Current methods require large amounts of images in both source and target domains for successful translation. However, data collection and annotations in many scenarios are infeasible or even impossible. In this paper, we propose an Object-Aware Few-Shot UI2I Translation (OA-FSUI2IT) framework to address the few-shot cross domain (FSCD) object detection task with limited unlabeled images in the target domain. To this end, we first introduce a discriminator augmentation (DA) module into the OA-FSUI2IT framework for successful few-shot UI2I translation. Then, we present a patch pyramid contrastive learning (PPCL) strategy to further improve the quality of the generated images. Last, we propose a self-supervised content-consistency (SSCC) loss to enforce the content-consistency in the translation. We implement extensive experiments to demonstrate the effectiveness of our OA-FSUI2IT framework for FSCD object detection and achieve state-of-the-art performance on the benchmarks of Normal-to-Foggy, Day-to-Night, and Cross-scene adaptation. The source code of our proposed method is also available at https://github.com/emdata-ailab/FSCD-Det.
更多查看译文
关键词
Computer Vision (CV),Machine Learning (ML),Domain(s) Of Application (APP)
AI 理解论文
溯源树
样例
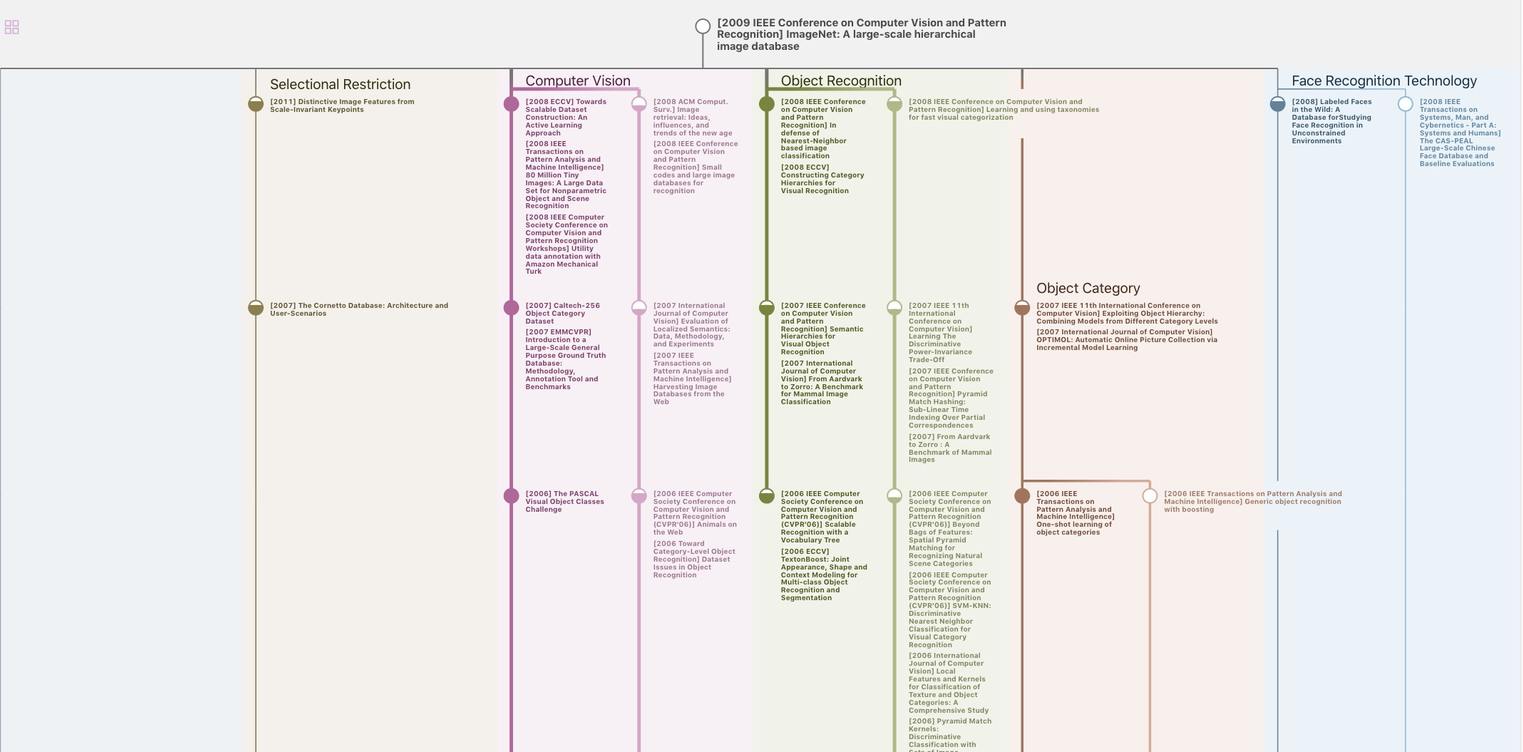
生成溯源树,研究论文发展脉络
Chat Paper
正在生成论文摘要