Localformer: a Locality-Preserving Vision Transformer
arxiv(2023)
摘要
Zigzag flattening (ZF) is commonly used in computer vision as a default option to unfold matrices, \eg in patch slicing for Vision Transformer (ViT). However, when decomposing multi-scale-object web images, ZF cannot preserve the smoothness of local information well. To address this, we draw inspiration from Space-Filling Curves (SFC) and investigate Hilbert flattening (HF) as an alternative for visual models. We provide a comprehensive theoretical discussion and practical analysis, demonstrating the superiority of HF over other SFC in locality and multi-scale robustness. We leverage HF to alleviate the problem of the lack of locality bias in the shallow layers of ViT, which formulates our Localformer. Extensive experiments demonstrate that Localformer consistently improves performance for several common visual tasks. Additionally, upon inspection, we find that Localformer enhances representation learning and length extrapolation abilities of ViT.
更多查看译文
AI 理解论文
溯源树
样例
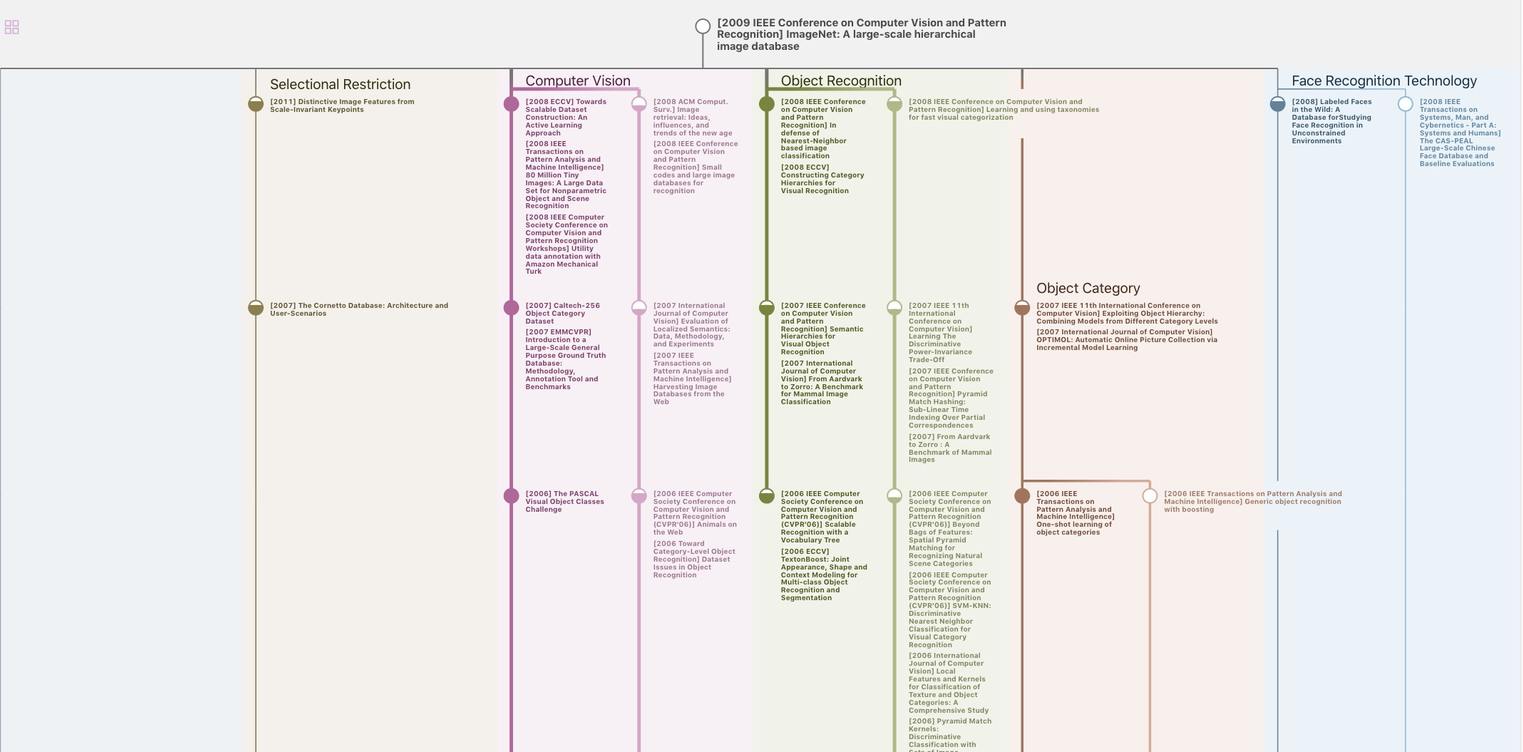
生成溯源树,研究论文发展脉络
Chat Paper
正在生成论文摘要