1-WL Expressiveness Is (Almost) All You Need.
IEEE International Joint Conference on Neural Network (IJCNN)(2022)
摘要
It has been shown that a message passing neural networks (MPNNs), a popular family of neural networks for graph-structured data, are at most as expressive as the first-order Weisfeiler-Leman (1-WL) graph isomorphism test, which has motivated the development of more expressive architectures. In this work, we analyze if the limited expressiveness is actually a limiting factor for MPNNs and other WL-based models in standard graph datasets. Interestingly, we find that the expressiveness of WL is sufficient to identify almost all graphs in most datasets. Moreover, we find that the classification accuracy upper bounds are often close to 100\%. Furthermore, we find that simple WL-based neural networks and several MPNNs can be fitted to several datasets. In sum, we conclude that the performance of WL/MPNNs is not limited by their expressiveness in practice.
更多查看译文
关键词
limiting factor,graph datasets,classification accuracy upper bounds,neural networks,1-WL expressiveness,graph-structured data,first-order Weisfeiler-Leman graph isomorphism test,message passing neural networks,MPNNs
AI 理解论文
溯源树
样例
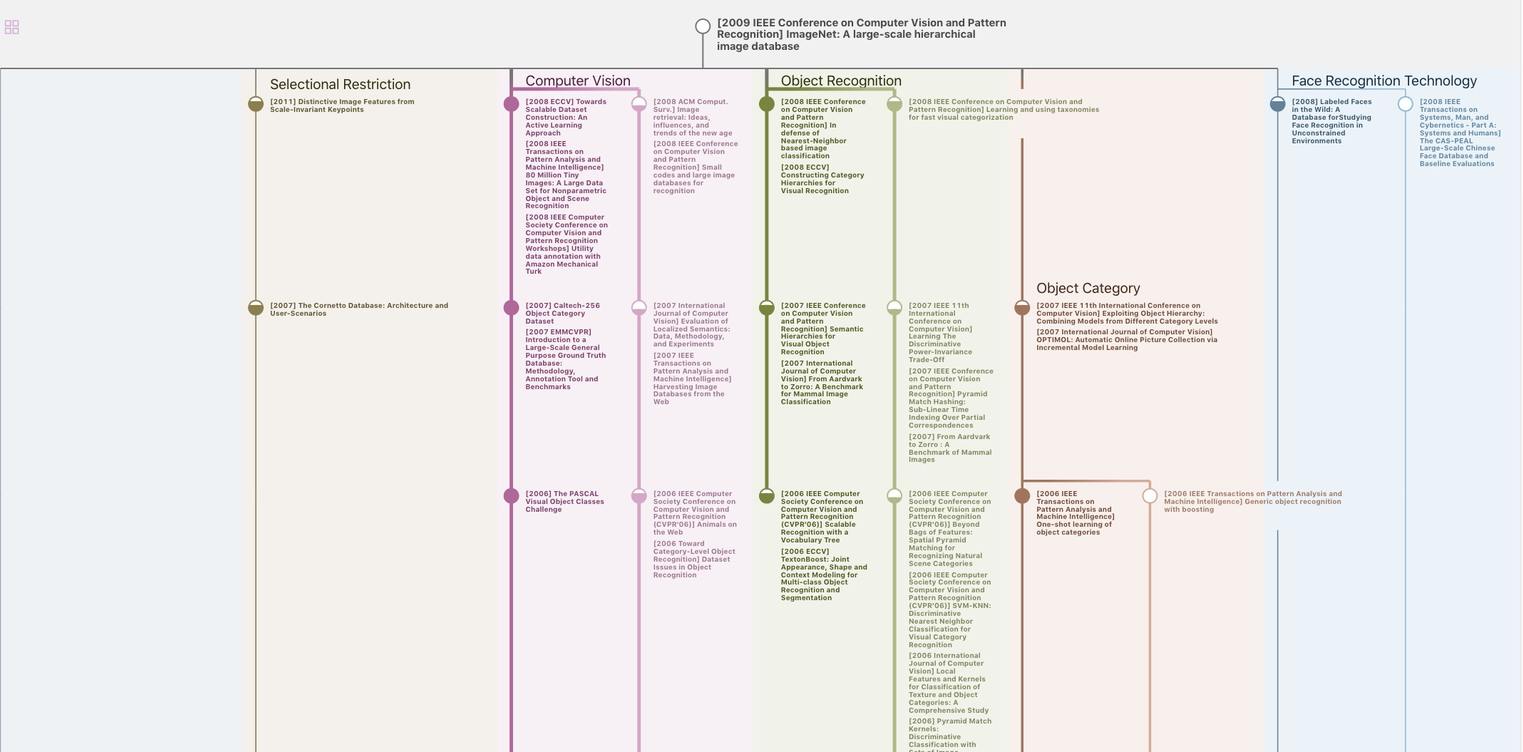
生成溯源树,研究论文发展脉络
Chat Paper
正在生成论文摘要