Dynamic survival analysis for non-Markovian epidemic models
JOURNAL OF THE ROYAL SOCIETY INTERFACE(2022)
摘要
We present a new method for analysing stochastic epidemic models under minimal assumptions. The method, dubbed dynamic survival analysis (DSA), is based on a simple yet powerful observation, namely that population-level mean-field trajectories described by a system of partial differential equations may also approximate individual-level times of infection and recovery. This idea gives rise to a certain non-Markovian agent-based model and provides an agent-level likelihood function for a random sample of infection and/or recovery times. Extensive numerical analyses on both synthetic and real epidemic data from foot-and-mouth disease in the UK (2001) and COVID-19 in India (2020) show good accuracy and confirm the method's versatility in likelihood-based parameter estimation. The accompanying software package gives prospective users a practical tool for modelling, analysing and interpreting epidemic data with the help of the DSA approach.
更多查看译文
关键词
spatial epidemic models, parameter inference, MCMC methods, survival analysis
AI 理解论文
溯源树
样例
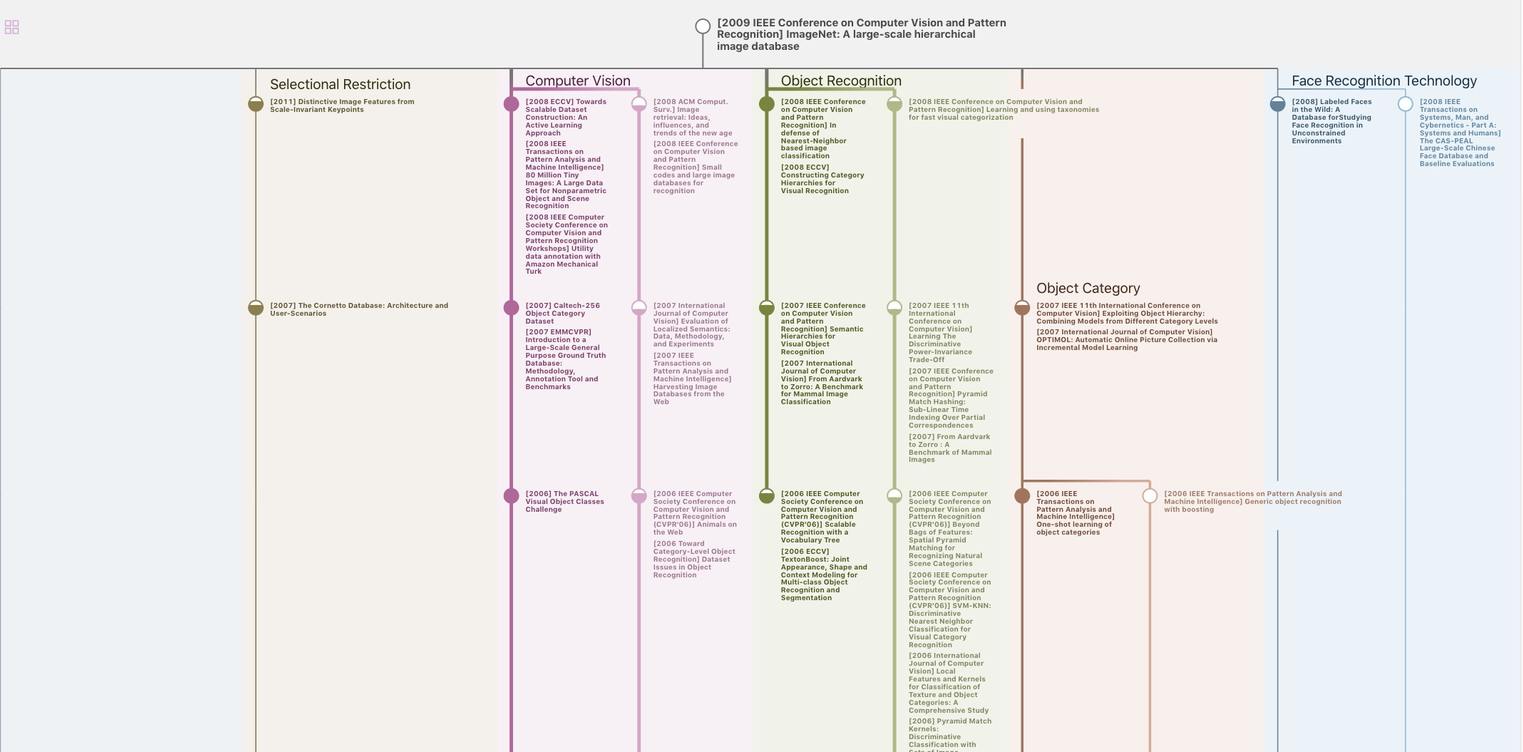
生成溯源树,研究论文发展脉络
Chat Paper
正在生成论文摘要