A Behavior Regularized Implicit Policy for Offline Reinforcement Learning
arxiv(2022)
摘要
Offline reinforcement learning enables learning from a fixed dataset, without further interactions with the environment. The lack of environmental interactions makes the policy training vulnerable to state-action pairs far from the training dataset and prone to missing rewarding actions. For training more effective agents, we propose a framework that supports learning a flexible yet well-regularized fully-implicit policy. We further propose a simple modification to the classical policy-matching methods for regularizing with respect to the dual form of the Jensen--Shannon divergence and the integral probability metrics. We theoretically show the correctness of the policy-matching approach, and the correctness and a good finite-sample property of our modification. An effective instantiation of our framework through the GAN structure is provided, together with techniques to explicitly smooth the state-action mapping for robust generalization beyond the static dataset. Extensive experiments and ablation study on the D4RL benchmark validate our framework and the effectiveness of our algorithmic designs.
更多查看译文
关键词
offline reinforcement
AI 理解论文
溯源树
样例
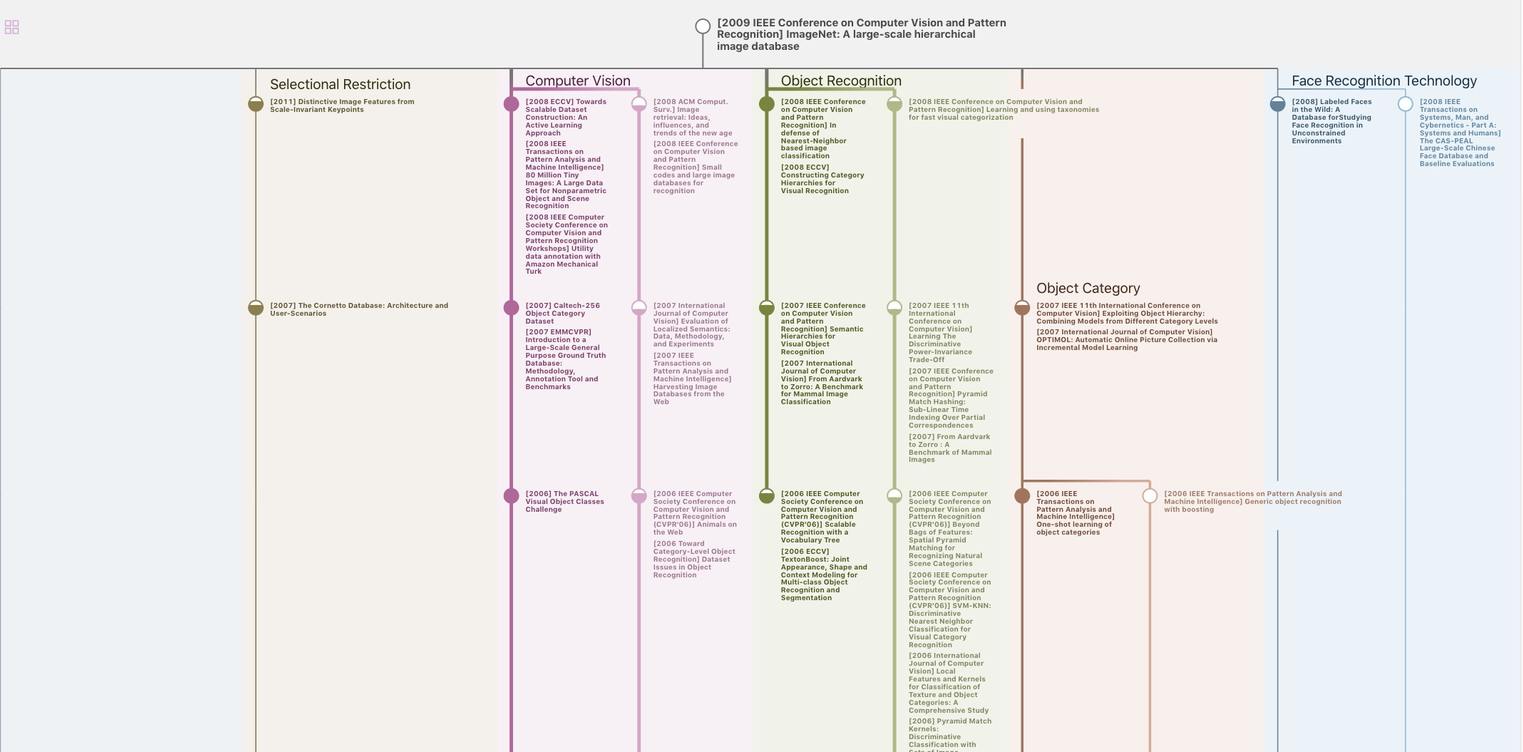
生成溯源树,研究论文发展脉络
Chat Paper
正在生成论文摘要