Gradient Estimation with Discrete Stein Operators
NeurIPS 2022(2022)
摘要
Gradient estimation -- approximating the gradient of an expectation with respect to the parameters of a distribution -- is central to the solution of many machine learning problems. However, when the distribution is discrete, most common gradient estimators suffer from excessive variance. To improve the quality of gradient estimation, we introduce a variance reduction technique based on Stein operators for discrete distributions. We then use this technique to build flexible control variates for the REINFORCE leave-one-out estimator. Our control variates can be adapted online to minimize the variance and do not require extra evaluations of the target function. In benchmark generative modeling tasks such as training binary variational autoencoders, our gradient estimator achieves substantially lower variance than state-of-the-art estimators with the same number of function evaluations.
更多查看译文
关键词
Gradient estimation,Stein's method,Markov chain,score function,REINFORCE,discrete latent variables,VAE,control variates,variance reduction
AI 理解论文
溯源树
样例
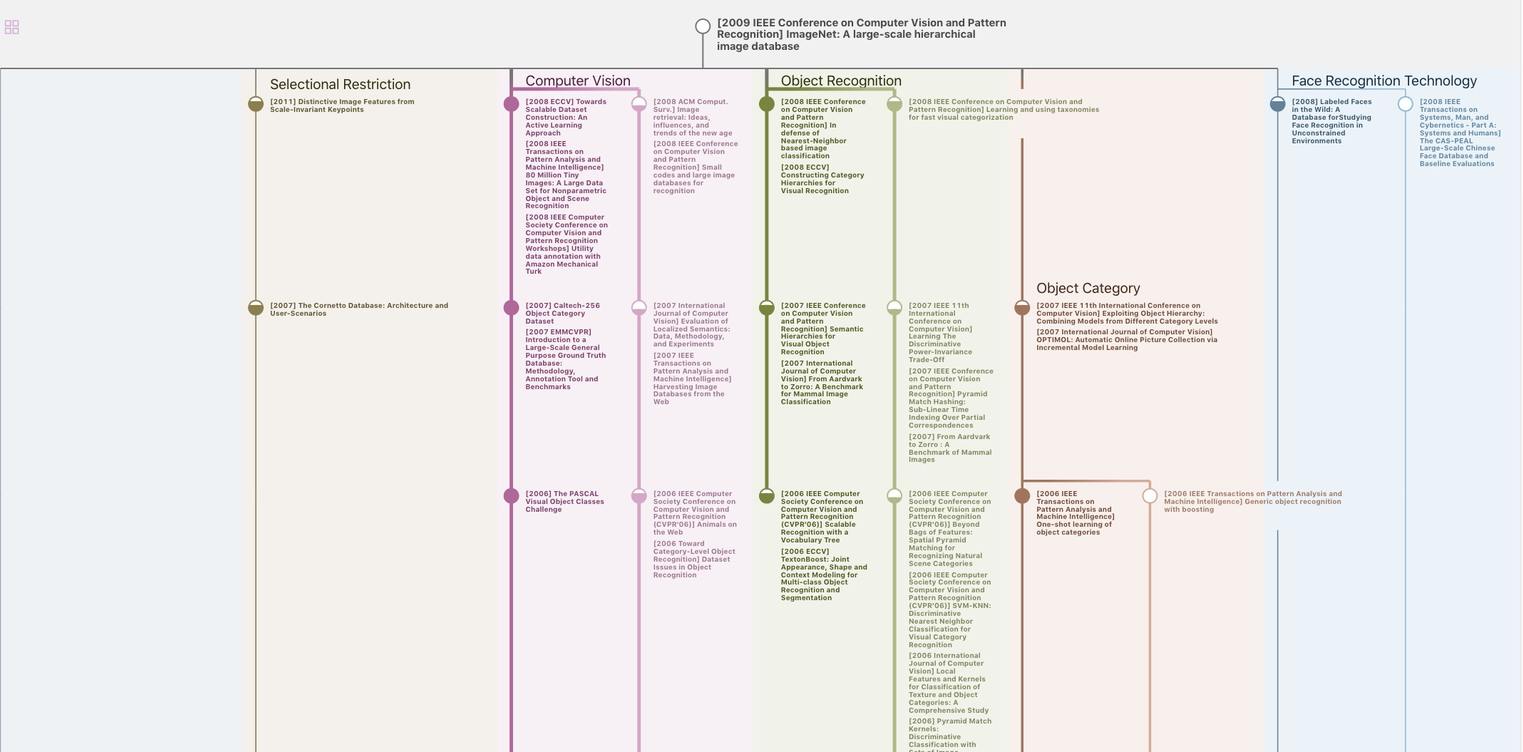
生成溯源树,研究论文发展脉络
Chat Paper
正在生成论文摘要