Incorporating Texture Information into Dimensionality Reduction for High-Dimensional Images
2022 IEEE 15th Pacific Visualization Symposium (PacificVis)(2022)
摘要
High-dimensional imaging is becoming increasingly relevant in many fields from astronomy and cultural heritage to systems biology. Visual exploration of such high-dimensional data is commonly facilitated by dimensionality reduction. However, common dimensionality reduction methods do not include spatial information present in images, such as local texture features, into the construction of low-dimensional embeddings. Consequently, exploration of such data is typically split into a step focusing on the attribute space followed by a step focusing on spatial information, or vice versa. In this paper, we present a method for incorporating spatial neighborhood information into distance-based dimensionality reduction methods, such as t-Distributed Stochastic Neighbor Embedding (t-SNE). We achieve this by modifying the distance measure between high-dimensional attribute vectors associated with each pixel such that it takes the pixel's spatial neighborhood into account. Based on a classification of different methods for comparing image patches, we explore a number of different approaches. We compare these approaches from a theoretical and experimental point of view. Finally, we illustrate the value of the proposed methods by qualitative and quantitative evaluation on synthetic data and two real-world use cases.
更多查看译文
关键词
Mathematics of computing-Dimensionality reduction,Human-centered computing-Visualization techniques,Human-centered computing-Visual analytics
AI 理解论文
溯源树
样例
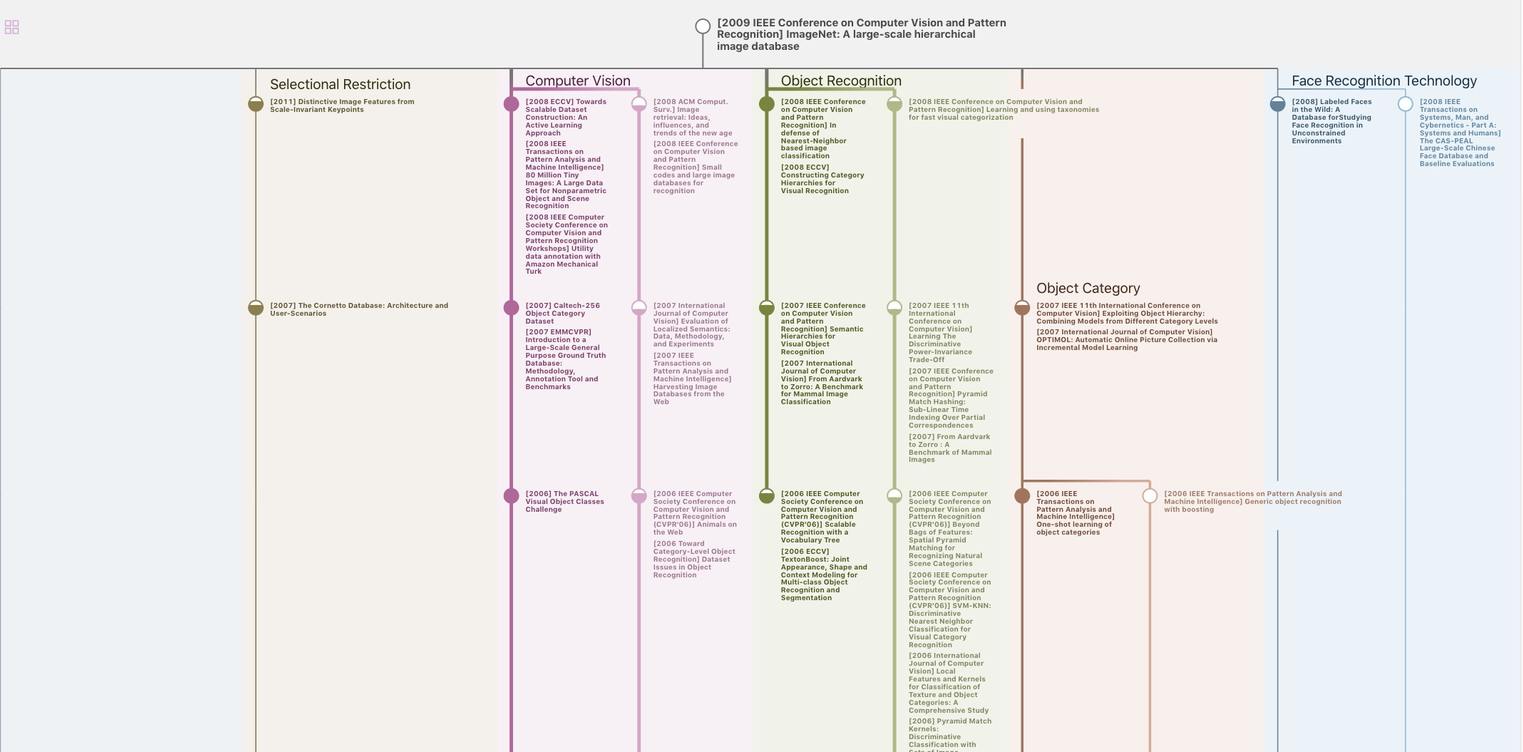
生成溯源树,研究论文发展脉络
Chat Paper
正在生成论文摘要