Predicting patients who will drop out of out-patient psychotherapy using machine learning algorithms
BRITISH JOURNAL OF PSYCHIATRY(2022)
摘要
Background About 30% of patients drop out of cognitive-behavioural therapy (CBT), which has implications for psychiatric and psychological treatment Findings concerning drop out remain heterogeneous. Aims This paper aims to compare different machine-learning algorithms using nested cross-validation, evaluate their benefit in naturalistic settings, and identify the best model as well as the most important variables. Method The data-set consisted of 2543 out-patients treated with CBT. Assessment took place before session one. Twenty-one algorithms and ensembles were compared. Two parameters (Brier score, area under the curve (AUC)) were used for evaluation. Results The best model was an ensemble that used Random Forest and nearest-neighbour modelling. During tie training process, it was significantly better than generalised linear modelling (GLM) (Brier score: d -2.93, 95% CI (-3.95, -1.90)); AUC: d = 0.59, 95% CI (0.11 to 1.06)). In the holdout sample, the ensemble was able to correctly identify 63.4% of cases of patients, wiereas the GLM only identified 46.2% correctly. The most important predictors were lower education, lower scores on the Personality Style and Disorder Inventory (PSSI) compulsive scale, younger age, higher scores on the PSSI negativistic and PSSI antisocial scale as well as on the Brief Symptom Inventory (BSI) additional scale (mean of the four additional Items) and BSI overall scale. Conclusions Machine learning improves drop-out predictions. However, not all algorithms are suited to naturalistic data-sets and binary events. Tree-based and boosted algorithms including a variable selection process seem well-suited, whereas more advanced algorithms such as neural networks do not.
更多查看译文
关键词
drop out, machine learning, algorithms, ensembles, variable selection
AI 理解论文
溯源树
样例
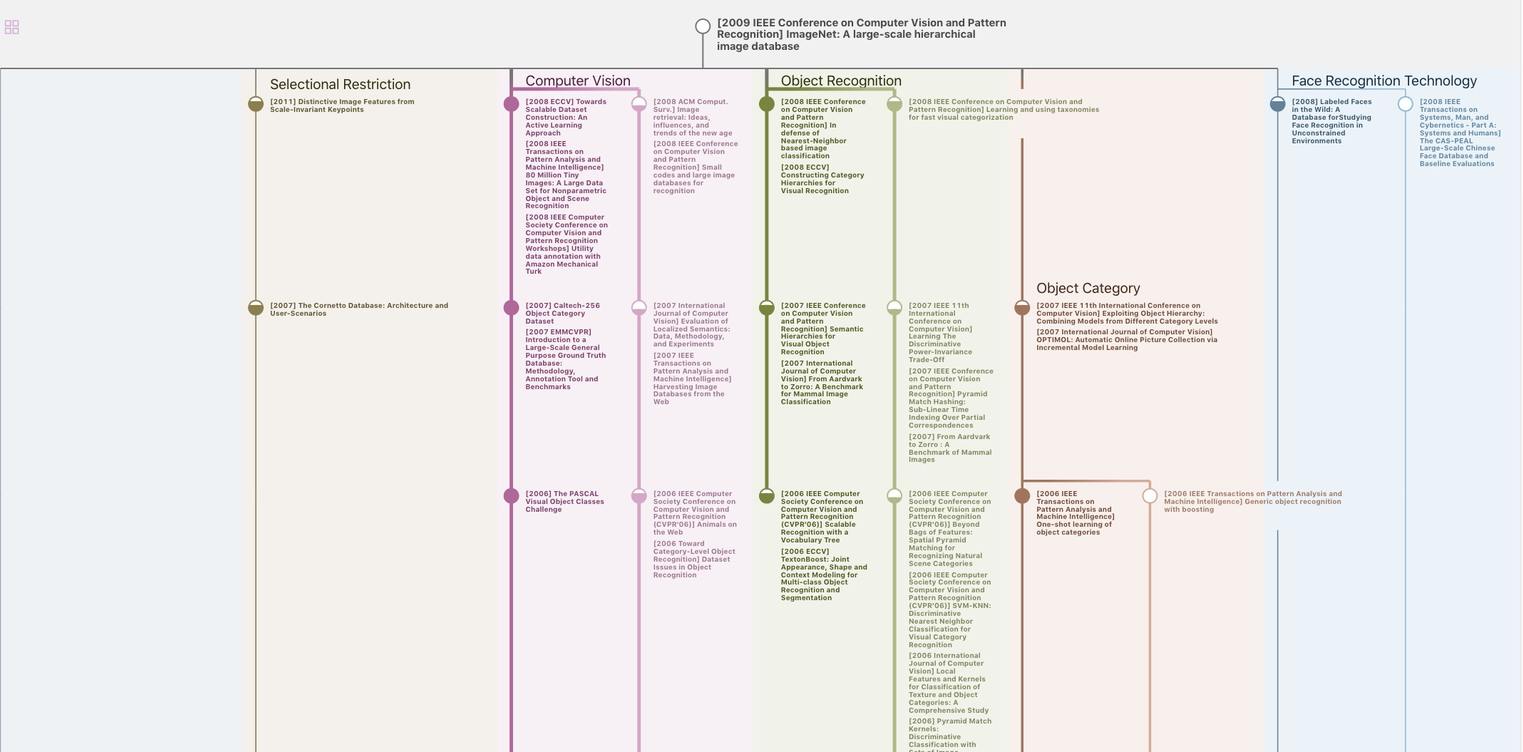
生成溯源树,研究论文发展脉络
Chat Paper
正在生成论文摘要