Historically understanding the spatial distributions of particle surface area concentrations over China estimated using a non-parametric machine learning method
Science of The Total Environment(2022)
摘要
A non-parametric ensemble model was proposed to estimate the long-term (2015–2019) particle surface area concentrations (SA) over China for the first time on basis of a vilification dataset of measured particle number size distribution. This ensemble model showed excellent cross-validation R2 value (CV R2 = 0.83) as well as a relatively low root-mean-square error (RMSE = 195.0 μm2/cm3). No matter in which year, considerable spatial heterogeneity of SA was found over China with higher SA in Beijing-Tianjin-Hebei (BTH), Yangtze River Delta (YRD), and Middle Lower Reaches of Yangtze River (MLYR). From 2015 to 2019, SA significantly decreased in representative city clusters. The reduction rates were 140.1 μm2·cm−3·a−1 in BTH, 110.7 μm2·cm−3·a−1 in Pearl River Delta (PRD), 105.2 μm2·cm−3·a−1 in YRD, and 92.4 μm2·cm−3·a−1 in Sichuan Basin (SCB), respectively. Even though such quick reduction, high SA (ranged from ~800 μm2/cm3 to ~1750 μm2/cm3) during the heavy pollution period (PM2.5 > 75 μg/m3) still existed in the above-mentioned city clusters and may provide rich reaction vessels for multiphase chemistry. A dichotomy of enhanced annual 4th maximum daily 8-h average O3 concentrations (4MDA8 O3) and decreased SA during summertime was found in Shanghai, a representative city of YRD. In Chengdu (SCB), increased 4MDA8 O3 concentration was associated with a synchronous increase of SA from 2017 to 2019. Differently, 4MDA8 O3 concentrations enhanced in Beijing (BTH) and Guangzhou (PRD), while not significant for SA before 2018. This work will greatly deepen our understanding of the historical variation and spatial distributions of SA over China.
更多查看译文
关键词
Particle surface area concentration,Machine learning,Spatiotemporal distribution
AI 理解论文
溯源树
样例
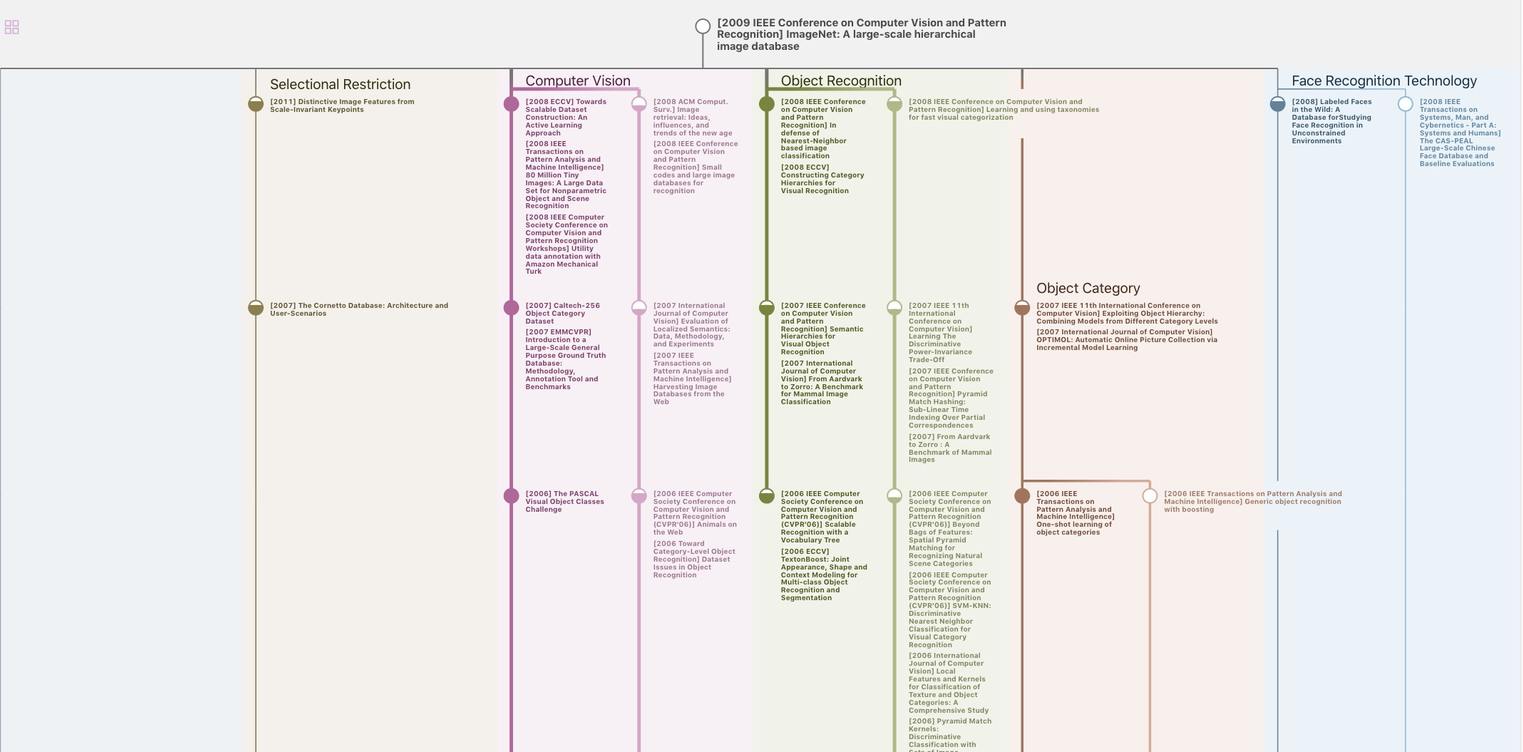
生成溯源树,研究论文发展脉络
Chat Paper
正在生成论文摘要