CurL-AutoML - Curriculum Learning-based AutoML.
ICMLA(2021)
摘要
AutoML aims to find the best Machine Learning (ML) pipeline in a complex and high-dimensional search space by evaluating multiple algorithm configurations. However, training multiple ML algorithms is time-consuming, and as AutoML tools are frequently time-constrained, the exploration of the search space may find sub-optimal results. In this work, we explore the application of curriculum learning techniques to overcome this limitation. Curriculum and anti-curriculum learning have improved model performance and accelerated the training process on previous empirical investigations using optimization-based models by ordering examples during model training based on their difficulty. We apply and compare curriculum strategies on an AutoML system to accelerate the search space exploration and find good-performing machine learning pipelines efficiently. The results indicate that AutoML can benefit from a curriculum strategy. Furthermore, in most of the evaluated scenarios, the curriculum strategies led to better classification results.
更多查看译文
关键词
Automated Machine Learning,Curriculum Learning,Hyper-Parameter Optimization
AI 理解论文
溯源树
样例
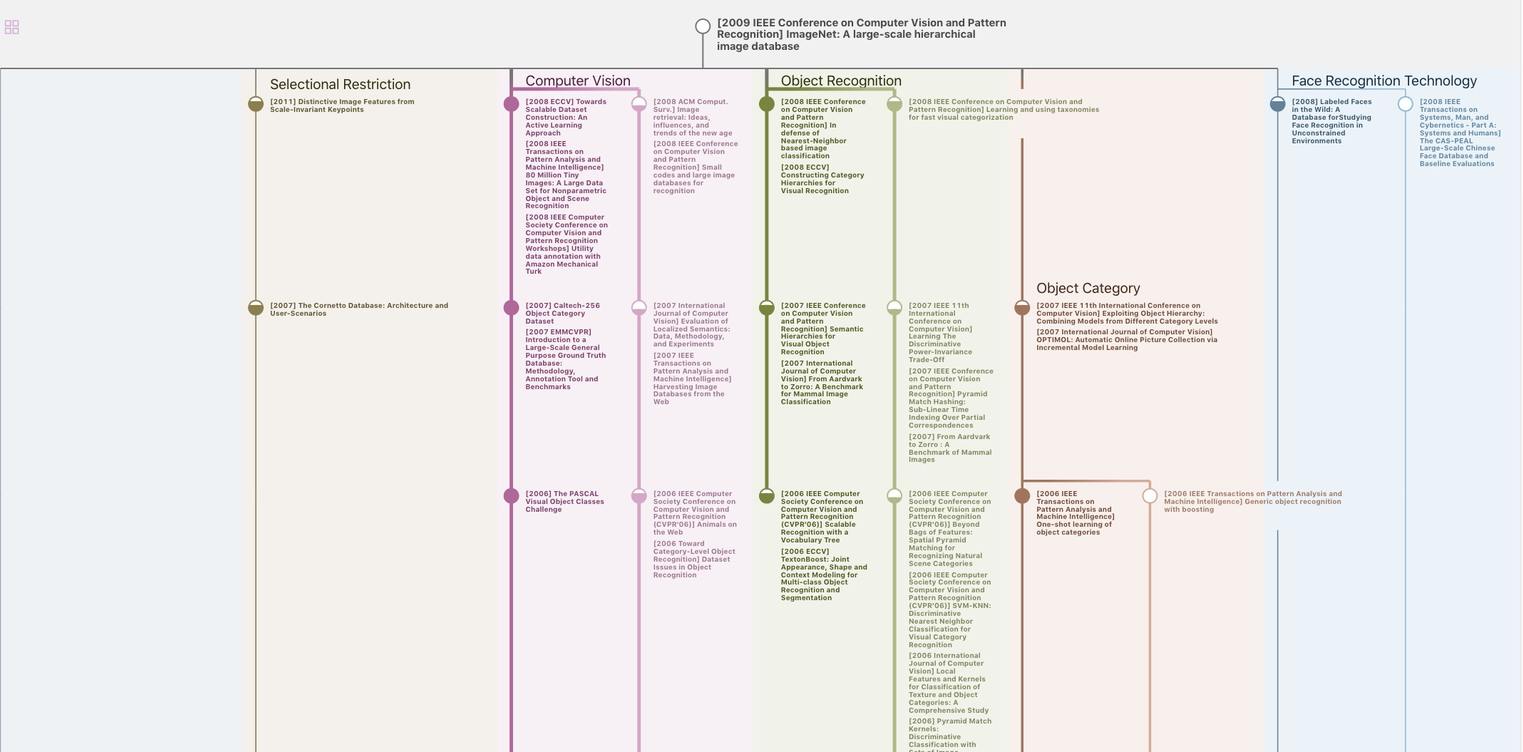
生成溯源树,研究论文发展脉络
Chat Paper
正在生成论文摘要