Aspect-based Sentiment Classification via Reinforcement Learning
2021 21ST IEEE INTERNATIONAL CONFERENCE ON DATA MINING (ICDM 2021)(2021)
摘要
Aspect-based sentiment classification aims to predict sentimental polarities of one or multiple aspects in texts. As texts always contain a large proportion of task-irrelevant words, accurate alignment between aspects and their sentimental descriptions is the most crucial and challenging step. State-of-the-art approaches are mainly based on word-level attention learned from recurrent neural network variants (e.g., LSTM) or graph neural networks. From another view, these methods essentially weight and aggregate all possible alignments. However, this mechanism heavily relies on large-scale supervision training: without enough labels, it could easily overfit with difficulty in generalization. To address this challenge, we propose SentRL, a reinforcement learning-based framework for aspect-based sentiment classification. In this framework, input texts are transformed into their dependency graphs. Then, an agent is deployed to walk on the graphs, explores paths from target aspect nodes to their potential sentimental regions, and differentiates the effectiveness of different paths. By limiting the agent's exploration budget, our method encourages the agent to skip task-irrelevant information and focus on the most effective paths for alignment purpose. Our method considerably reduces the impact of task-irrelevant words and improves generalization performance. Compared with competitive baseline methods, our approach achieves the highest performance on public benchmark datasets with up to 3.7% improvement.
更多查看译文
关键词
Natural Language Processing, Sentiment Classification, Reinforcement Learning
AI 理解论文
溯源树
样例
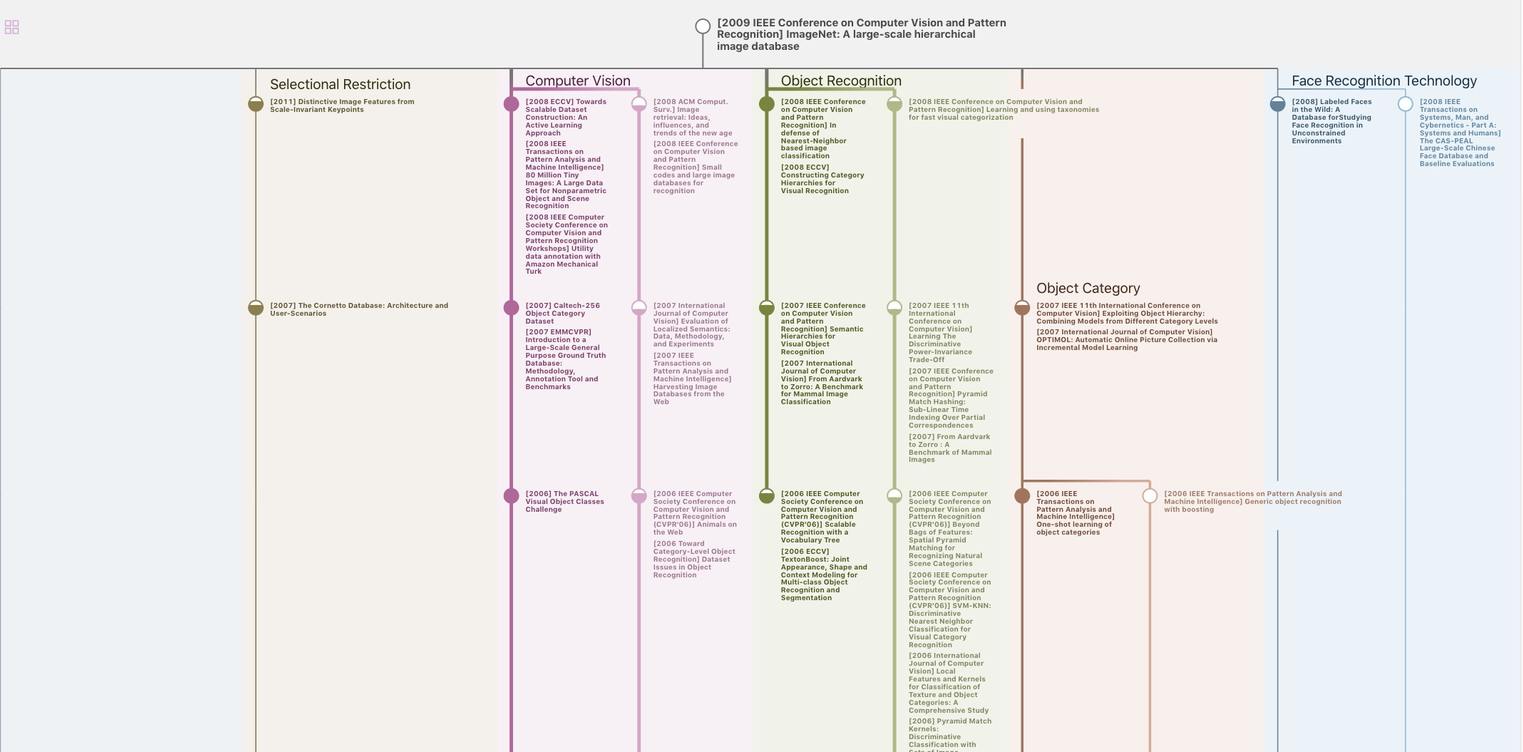
生成溯源树,研究论文发展脉络
Chat Paper
正在生成论文摘要