Accurately Quantifying under Score Variability
2021 21ST IEEE INTERNATIONAL CONFERENCE ON DATA MINING (ICDM 2021)(2021)
摘要
The quantification objective is to predict the class distribution of a data sample. Therefore, this task intrinsically involves a drift in the class distribution that causes a mismatch between the training and test sets. However, existing quantification approaches assume that the feature distribution is stationary. We analyse for the first time how score-based quantifiers are affected by concept drifts and propose a novel drift-resilient quantifier for binary classes. Our proposal does not model the different types of concept drifts. Instead, we model the changes that such changes cause in the classification scores. This observation simplifies our analysis since distribution changes can only increase, decrease or maintain the overlap of the positive and negative classes in a rank induced by the scores. Our paper has two main contributions. The first one is MoSS, a model for synthetic scores. We use this model to show that state-of-the-art quantifiers underperform in the occurrence of any concept drift that changes the score distribution. Our second contribution is a quantifier, DySyn, that uses MoSS to estimate the class distribution. We show that DySyn statistically outperforms state-of-the-art quantifiers in a comprehensive comparison with real-world and benchmark datasets in the presence of concept drifts.
更多查看译文
关键词
Counting, concept drift, machine learning
AI 理解论文
溯源树
样例
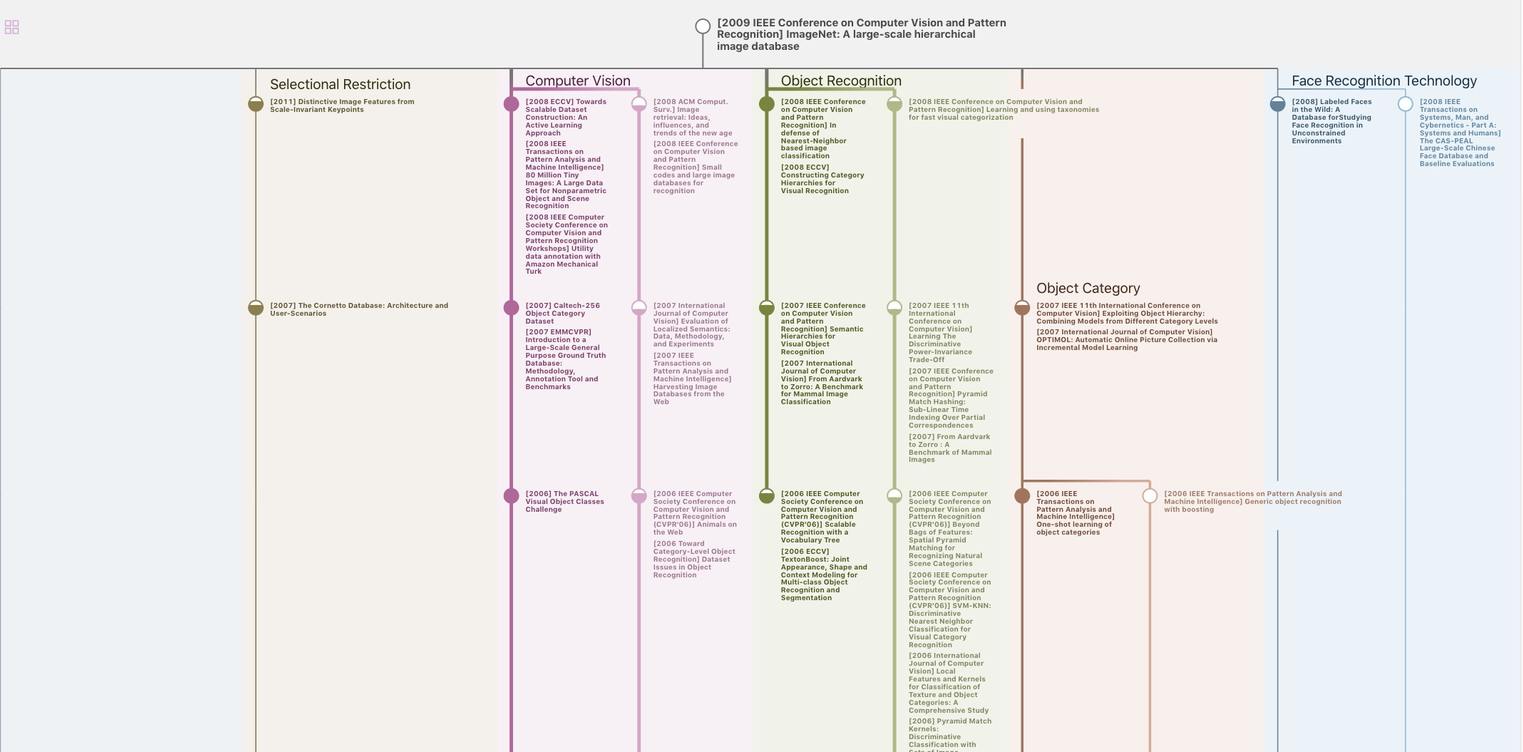
生成溯源树,研究论文发展脉络
Chat Paper
正在生成论文摘要