Bi-Level Attention Graph Neural Networks
2021 21ST IEEE INTERNATIONAL CONFERENCE ON DATA MINING (ICDM 2021)(2021)
摘要
Recent graph neural networks (GNNs) with the attention mechanism have historically been limited to small-scale homogeneous graphs (HoGs). However, GNNs handling heterogeneous graphs (HeGs), which contain several entity and relation types, all have shortcomings in handling attention. Most GNNs that learn graph attention for HeGs learn either node-level or relation-level attention, but not both, limiting their ability to predict both important entities and relations in the HeG. Even the best existing method that learns both levels of attention has the limitation of assuming graph relations are independent and that its learned attention disregards this dependency association. To effectively model both multi-relational and multi-entity large-scale HeGs, we present Bi-Level Attention Graph Neural Networks (BA-GNN), scalable neural networks (NNs) that use a novel bi-level graph attention mechanism. BA-GNN models both node-node and relation-relation interactions in a personalized way, by hierarchically attending to both types of information from local neighborhood contexts instead of the global graph context. Rigorous experiments on seven real-world HeGs show BA-GNN consistently outperforms all baselines, and demonstrate quality and transferability of its learned relation-level attention to improve performance of other GNNs.
更多查看译文
关键词
graph neural networks, representation learning
AI 理解论文
溯源树
样例
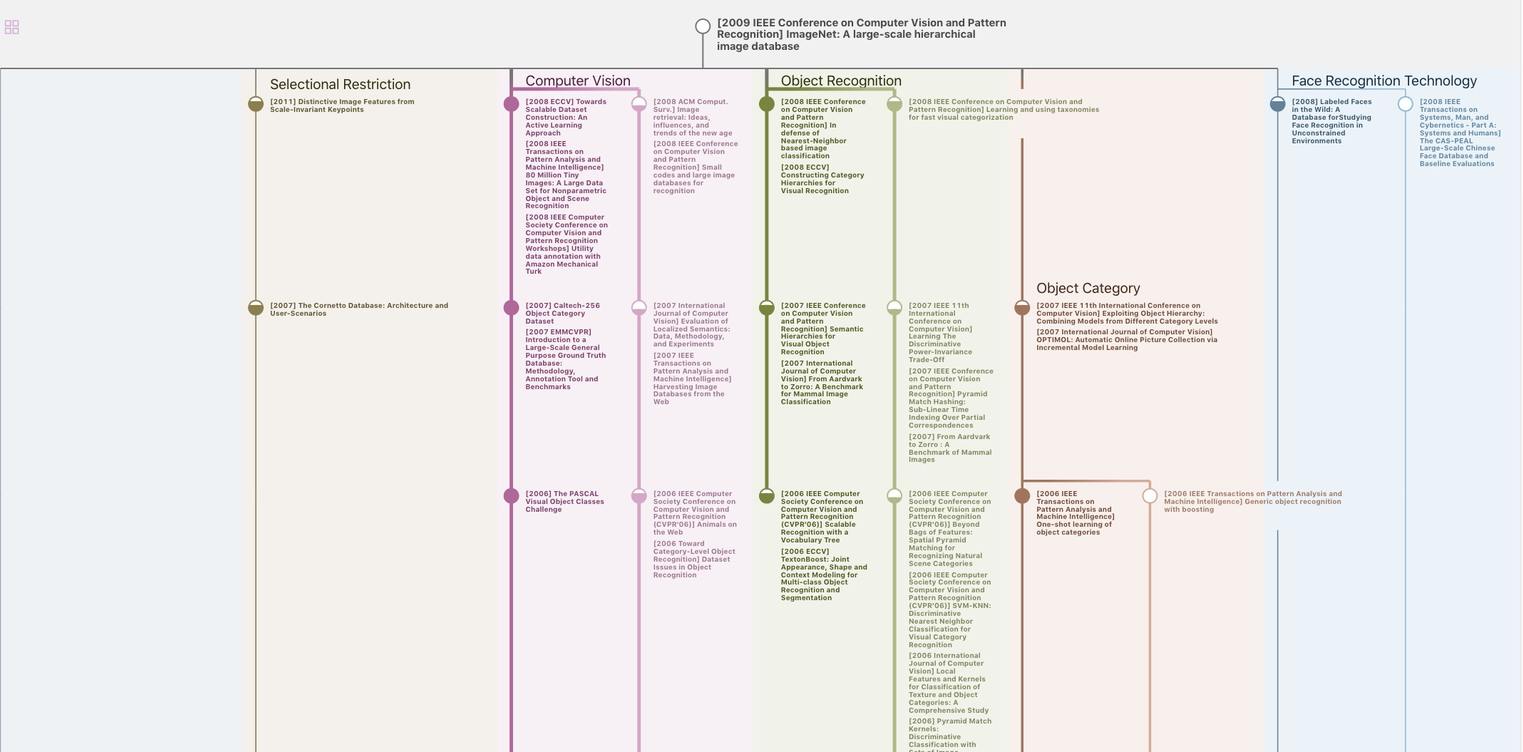
生成溯源树,研究论文发展脉络
Chat Paper
正在生成论文摘要