Oil Leakage Detection in Automobile Shock Absorber using Machine Learning Classifiers.
ICRCA(2021)
摘要
In this paper, we describe a lightweight and accurate fault diagnosis method that detects oil leakage in automobile shock absorbers. Our approach includes a machine learning classifier as its base. These classifiers are quick and maintain a low computational load that are suitable for the automotive environment where only low-performance Electronic Control Units (ECUs) are available. However, these classifiers do not produce sufficiently accurate results when raw sensor data is used as the input. To solve this issue, we have developed an approach that firstly includes sensor data selection, where only sensors that have a strong impact on accuracy are used as input. And secondly, this reduced input dataset undergoes preprocessing using Fast Fourier Transform (FFT) to further improve accuracy. Thus, our methodology produces an oil leakage detection methodology for automobile shock absorbers that addresses the limitations of fault detection in an automotive system by being both lightweight and accurate.
更多查看译文
AI 理解论文
溯源树
样例
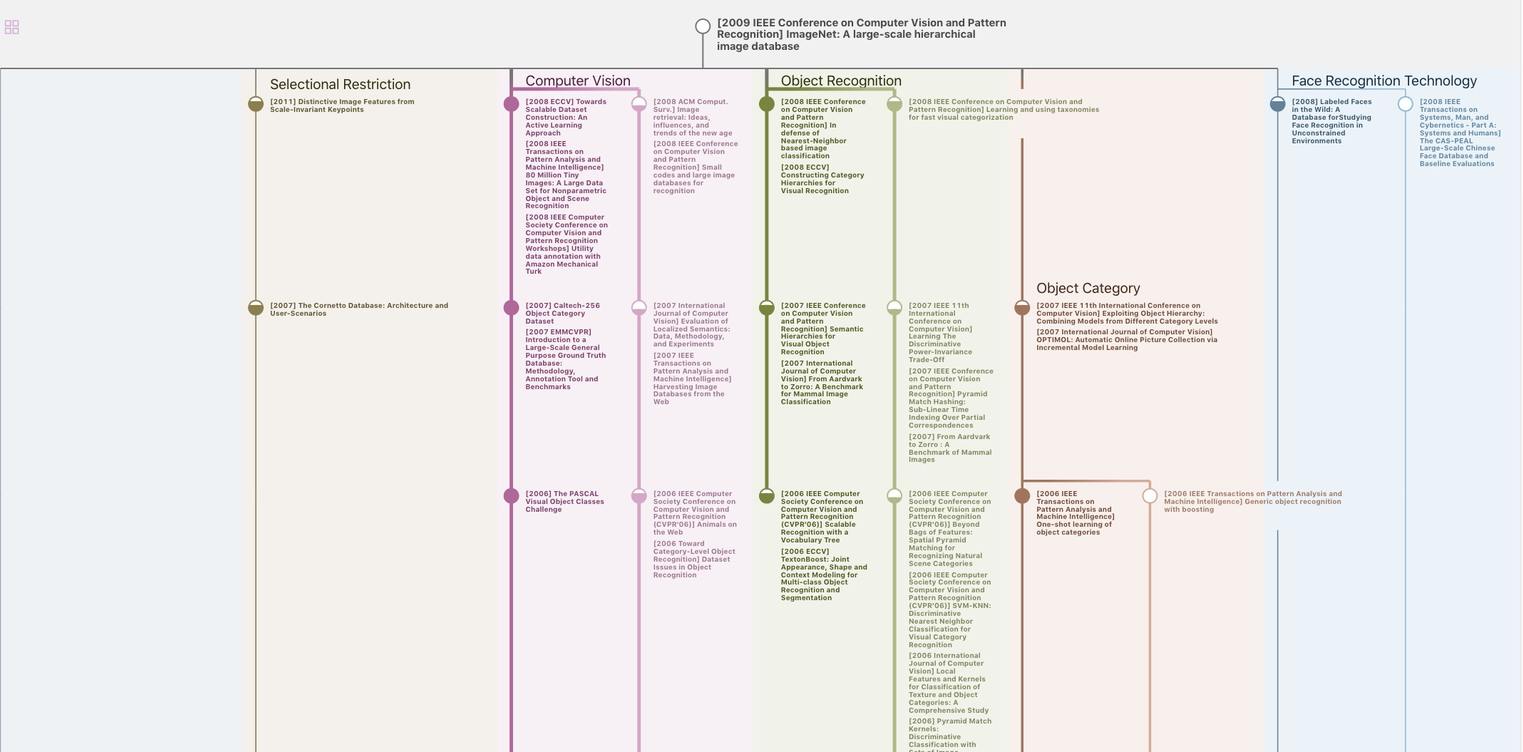
生成溯源树,研究论文发展脉络
Chat Paper
正在生成论文摘要