CVAE-AN: Atypical Attack Flow Detection Using Incremental Adversarial Learning
2021 IEEE GLOBAL COMMUNICATIONS CONFERENCE (GLOBECOM)(2021)
摘要
Network Intrusion Detection Systems (NIDS) are powerful tools for identifying and deterring cybersecurity attacks nowadays. However, while these modern IDS can detect typical attacks, recent studies show their poor performances in identifying unknown or dynamically changing atypical attacks. Another issue with the training aspect of such systems is the problem of class imbalance which impedes their performance, especially for minority attack classes. This renders IDS systems vulnerable to both adversarial as well as non-AI synthesized atypical attacks when deployed in a real network. To reduce misclassification (especially for minority classes) and detect atypical attack flows, we propose a novel adversarial incremental learning approach based on a hybrid model consisting of a Conditional Variational Autoencoder (CVAE) and a Generative Adversarial Network (GAN) namely, CVAE-Adversarial Network (CVAE-AN). The binary IDS has been trained using the CICIDS2017 dataset and evaluated using multiple atypical attacks. Simulation results demonstrate that the proposed technique significantly improves the performance of the IDS against different atypical attacks and outperforms the state-of-the-art detection models as well as class balancing methods.
更多查看译文
关键词
Artificial Intelligence (AI), Atypical Attacks, Class Imbalance, Feature Profile, Intrusion Detection System (IDS)
AI 理解论文
溯源树
样例
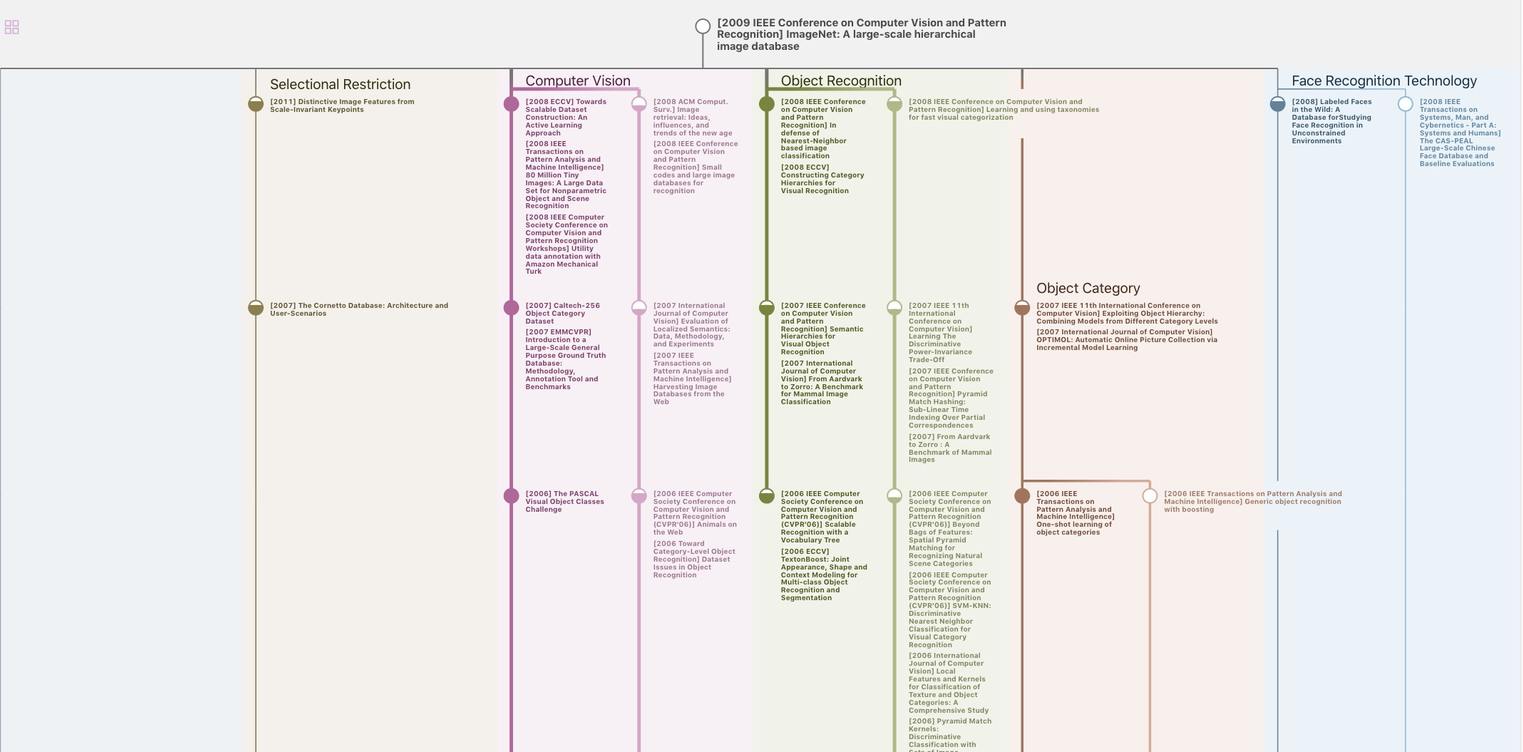
生成溯源树,研究论文发展脉络
Chat Paper
正在生成论文摘要