CMF Net: Detecting Objects in Infrared Traffic Image with Combination of Multiscale Features
2021 IEEE GLOBAL COMMUNICATIONS CONFERENCE (GLOBECOM)(2021)
摘要
Infrared image target detection has always been a hot topic of research, but there is still little research on infrared image target detection in the field of transportation. In this paper, we use the idea of transfer learning to transfer the target detection framework in the visible domain of deep learning to the infrared domain, and propose the target detection model CMF Net based on multi-scale feature fusion. CMF Net uses two multi-scale feature extraction mechanisms and features fusion, so that the final output feature map of the backbone network contains not only low-level visual features which are beneficial to target localization, but also high-level semantic features which are beneficial to target recognition, and can adapt to multi-scale features of the target. The experiment verified the advantages of CMF Net, and its mAP on the test data of the infrared image data set FLIR reached about 71%. This result is an increase of about 13% compared to Faster R-CNN, an increase of about 6% compared to YOLO3, and an increase of about 17% compared to SSD.
更多查看译文
关键词
transfer learning, multiscale features, feature fusion, deep learning, infrared image, multi-target detection
AI 理解论文
溯源树
样例
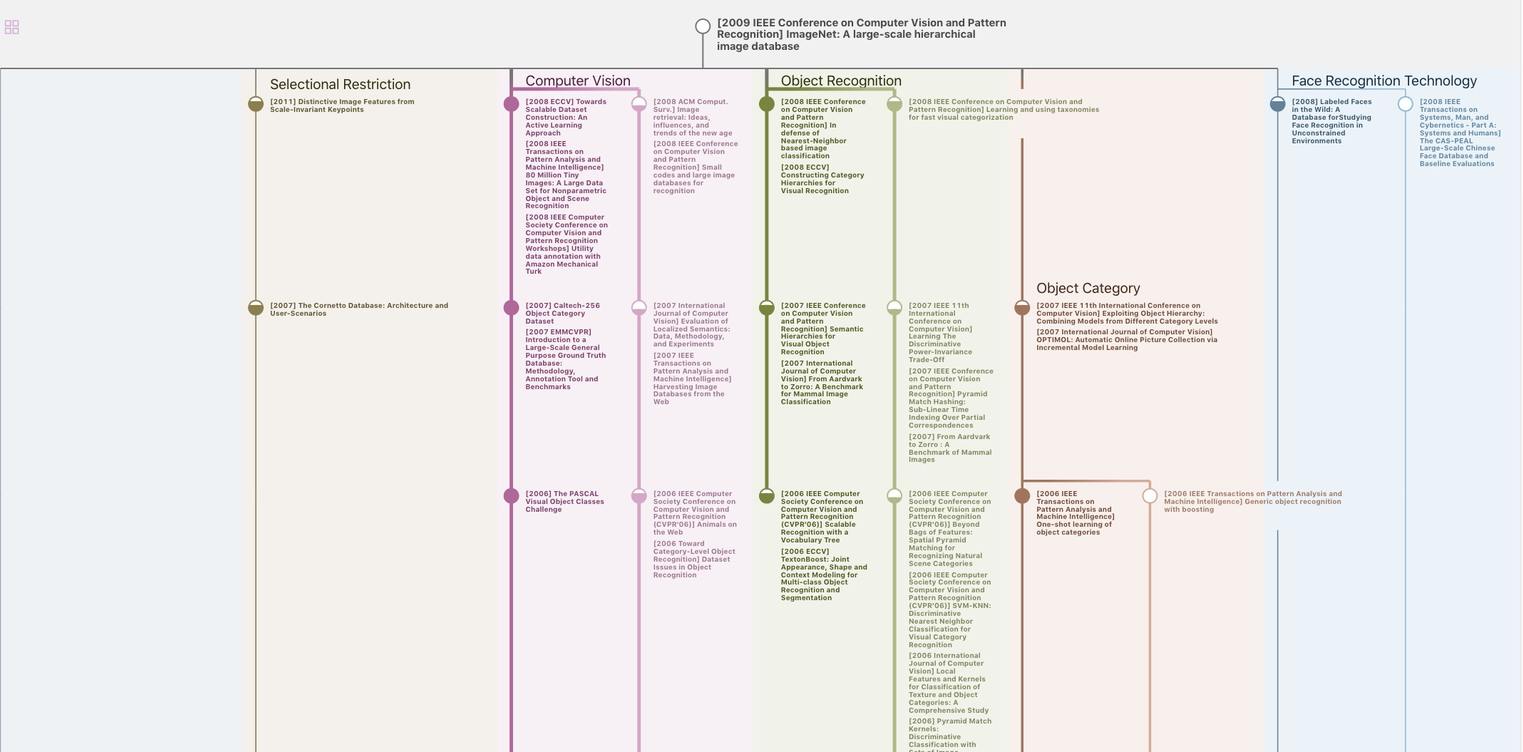
生成溯源树,研究论文发展脉络
Chat Paper
正在生成论文摘要