Data-Driven Quickest Change Detection for Securing Federated Learning for Internet-of-Vehicles
2021 IEEE GLOBAL COMMUNICATIONS CONFERENCE (GLOBECOM)(2021)
摘要
Machine Learning (ML) is on the verge of transitioning from centralized, distributed to federated learning (FL) due to the inherent privacy-preserving framework by FL. FL enables collaborative learning among participants just by exchanging updated model parameters with the FL server while keeping training data local in the end devices which is suitable for vehicular communications. However, this learning framework makes the life of the server difficult to detect the malicious behavior of participants. Malicious model updates from participants may affect the accuracy of the learning model considerably and consequently may cause severe consequences in the Internet of Vehicles (IoV) environment. To address this issue, we propose a novel approach to apply Shiryaev's quickest change detection (QCD) technique in the FL realm. QCD is applied to detect abnormal changes in statistical properties of model parameters in FL as quickly as possible. We apply QCD on the server-side in two ways. First, QCD is applied to detect a change in the statistical properties over the model parameters sent by the participating devices. Second, QCD is applied to the history of aggregated FL model parameters. The first approach facilitates identifying malicious clients which can be eliminated in future learning activities. The other approach assists the server to roll back to an earlier version of the model in case of identifying the anomaly in the aggregated FL parameters. As QCD is applied on the server-side, it does not add any computation overhead on the client-side as well as communication overheard during transmission. These two approaches are evaluated with the help of numerical results.
更多查看译文
关键词
Machine learning, Federated Learning, Internet of Vehicles, Quickest Change Detection
AI 理解论文
溯源树
样例
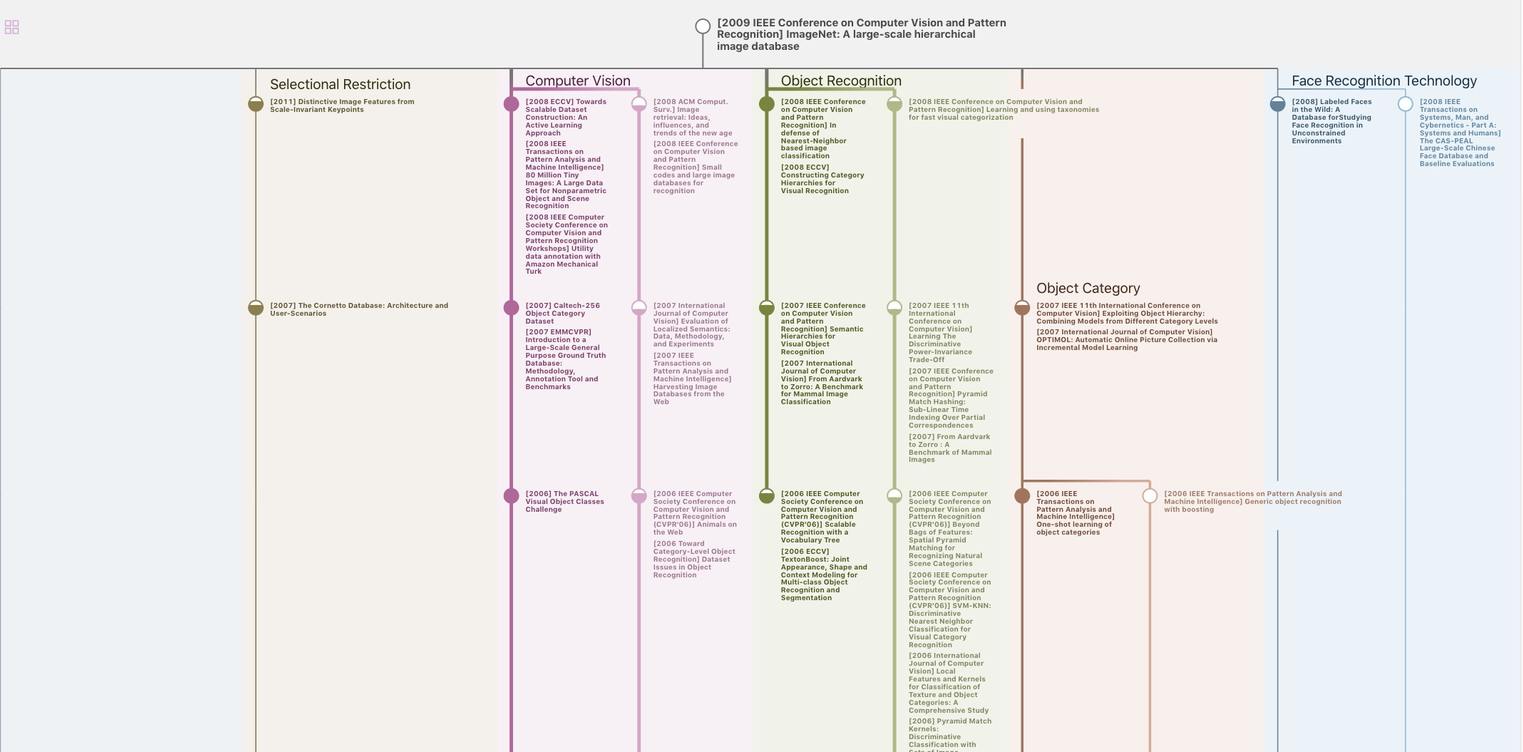
生成溯源树,研究论文发展脉络
Chat Paper
正在生成论文摘要