Spider: Deep Learning-driven Sparse Mobile Traffic Measurement Collection and Reconstruction
2021 IEEE GLOBAL COMMUNICATIONS CONFERENCE (GLOBECOM)(2021)
摘要
Data-driven mobile network management hinges on accurate traffic measurements, which routinely require expensive specialized equipment and substantial local storage capabilities, and bear high data transfer overheads. To overcome these challenges, in this paper we propose Spider, a deep-learning-driven mobile traffic measurement collection and reconstruction framework, which reduces the cost of data collection while retaining state-of-the-art accuracy in inferring mobile traffic consumption with fine geographic granularity. Spider harnesses Reinforcement Learning and tackles large action spaces to train a policy network that selectively samples a minimal number of cells where data should be collected. We further introduce a fast and accurate neural model that extracts spatiotemporal correlations from historical data to reconstruct network-wide traffic consumption based on sparse measurements. Experiments we conduct with a real-world mobile traffic dataset demonstrate that Spider samples 48% fewer cells as compared to several benchmarks considered, and yields up to 67% lower reconstruction errors than state-of-the-art interpolation methods. Moreover, our framework can adapt to previously unseen traffic patterns.
更多查看译文
关键词
deep Learning-driven sparse mobile traffic measurement collection,accurate traffic measurements,expensive specialized equipment,substantial local storage capabilities,high data transfer overheads,data collection,mobile traffic consumption,reinforcement learning,policy network,accurate neural model,historical data,network-wide traffic consumption,sparse measurements,real-world mobile traffic dataset,Spider samples,lower reconstruction errors,unseen traffic patterns,deep learning-driven sparse mobile traffic measurement reconstruction,data-driven mobile network management,fine geographic granularity,interpolation methods
AI 理解论文
溯源树
样例
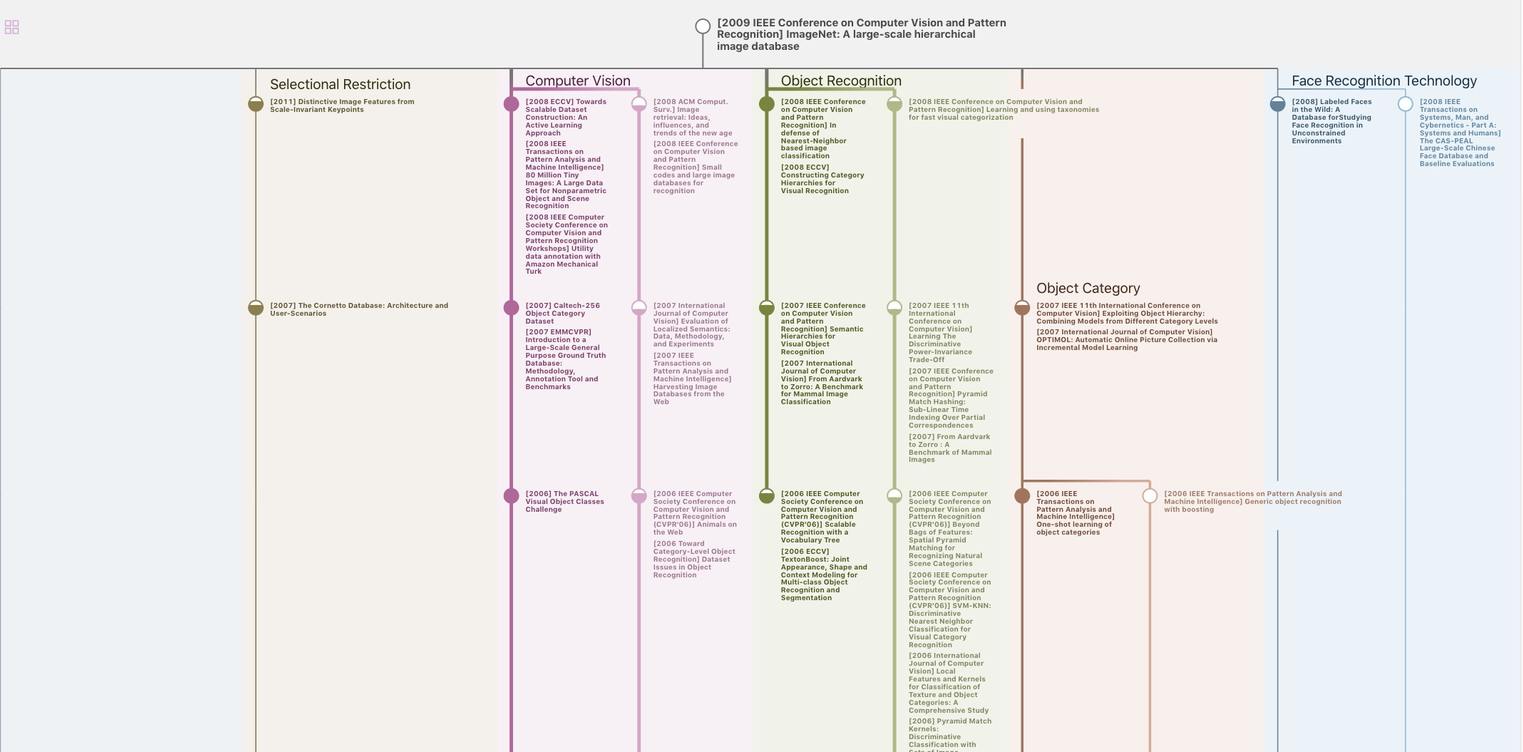
生成溯源树,研究论文发展脉络
Chat Paper
正在生成论文摘要