On the Reversibility of Adversarial Attacks.
ICIP(2021)
摘要
Adversarial attacks modify images with perturbations that change the prediction of classifiers. These modified images, known as adversarial examples, expose the vulnerabilities of deep neural network classifiers. In this paper, we investigate the predictability of the mapping between the classes predicted for original images and for their corresponding adversarial examples. This predictability relates to the possibility of retrieving the original predictions and hence reversing the induced misclassification. We refer to this property as the reversibility of an adversarial attack, and quantify reversibility as the accuracy in retrieving the original class or the true class of an adversarial example. We present an approach that reverses the effect of an adversarial attack on a classifier using a prior set of classification results. We analyse the reversibility of state-of-the-art adversarial attacks on benchmark classifiers and discuss the factors that affect the reversibility.
更多查看译文
关键词
Adversarial perturbations,Adversarial example,Deep neural network,Reversibility
AI 理解论文
溯源树
样例
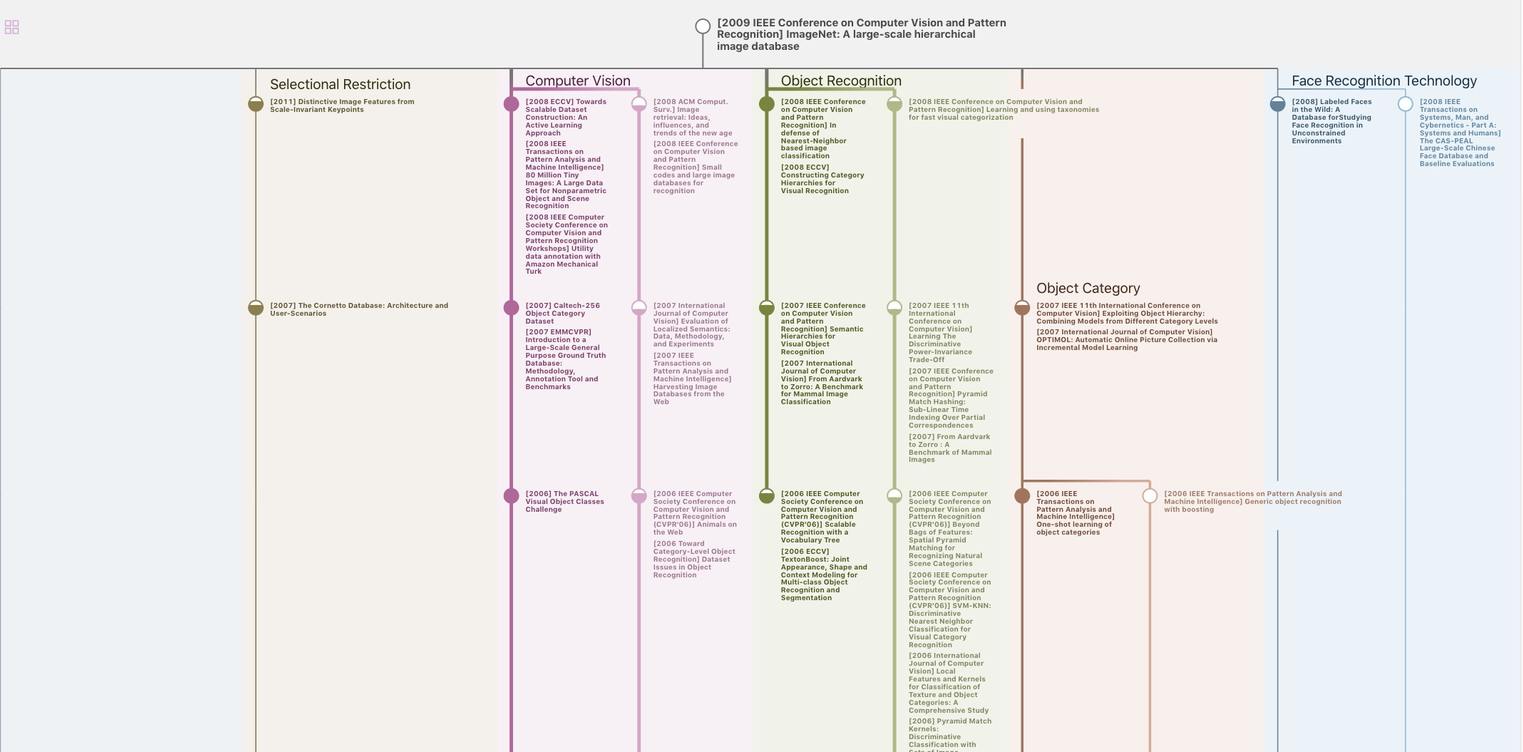
生成溯源树,研究论文发展脉络
Chat Paper
正在生成论文摘要