EBB - Progressive Optimization For Partial Domain Adaptation.
ICIP(2021)
摘要
Unsupervised domain adaptation (UDA) methods are generally proposed based on the assumption that the source domain and the target domain share an identical group of classes. However, in transfer learning tasks in reality, the target domain often has fewer data with missing classes. Partial domain adaptation (PDA) allows the source domain to have unshared categories. Anchor points are used to describe the easily identified target samples. It is observed that the shared classes tend to have more anchor points compared with the unshared classes and we introduce a novel progressive optimization method named Ebb PDA tasks. Ebb could resist the negative transfer caused by the category gap and can be applied to any domain adaptation model. Ebb picks the anchor points by analyzing the features of the base model, and it uses the class-wise distribution of anchor points to estimate the category gap. Then Ebb minimizes the errors of shared classes and corrects the error samples caused by blind alignment. To verify the effectiveness of the method, we apply Ebb to three PDA image classification tasks based on three widely used data sets, i.e, Office-Home, Office-31 and ImageCLEF-DA while using three state-of-the-art methods as the base models. The results show that Ebb brings a significant improvement in all tasks and the models optimized by Ebb have stable performance under a wide range of category gaps.
更多查看译文
关键词
Unsupervised Domain Adaptation,Partial Domain Adaptation,Progressive Optimization
AI 理解论文
溯源树
样例
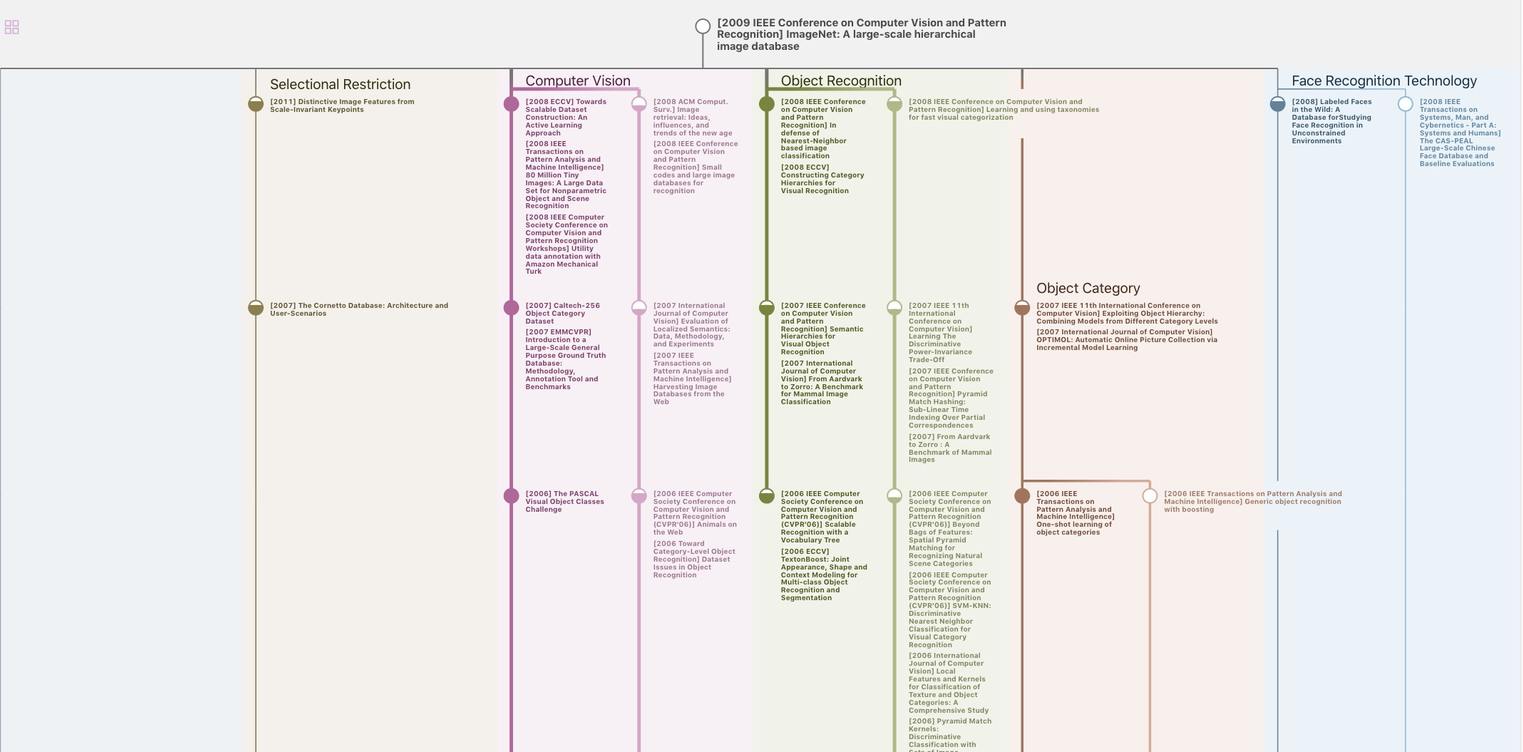
生成溯源树,研究论文发展脉络
Chat Paper
正在生成论文摘要