Optimally Weighted Ensembles for Efficient Multi-objective Optimization
MACHINE LEARNING, OPTIMIZATION, AND DATA SCIENCE (LOD 2021), PT I(2022)
摘要
The process of industrial design engineering is often involved with the simultaneous optimization of multiple expensive objectives. The surrogate assisted multi-objective S-Metric Selection - Efficient Global Optimization (SMS-EGO) algorithm is one of the most popular algorithms to solve these kind of problems. We propose an extension of the SMS-EGO algorithm with optimally weighted, linearly combined ensembles of regression models to improve its objective modelling capabilities. Multiple (different) surrogates are combined into one optimally weighted ensemble per objective using a model agnostic uncertainty quantification method to balance between exploration and exploitation. The performance of the proposed algorithm is evaluated on a diverse set of benchmark problems with a small initial sample and an additional budget of 25 evaluations of the real objective functions. The results show that the proposed Ensemble-based - S-Metric Selection - Efficient Global Optimization (E-SMS-EGO) algorithm outperforms the state-of-the-art algorithms in terms of efficiency, robustness and spread across the objective space.
更多查看译文
关键词
Multi-objective optimization, Efficient global optimization, Surrogate models, Ensemble models, Uncertainty quantification, S-metric selection, Industrial design
AI 理解论文
溯源树
样例
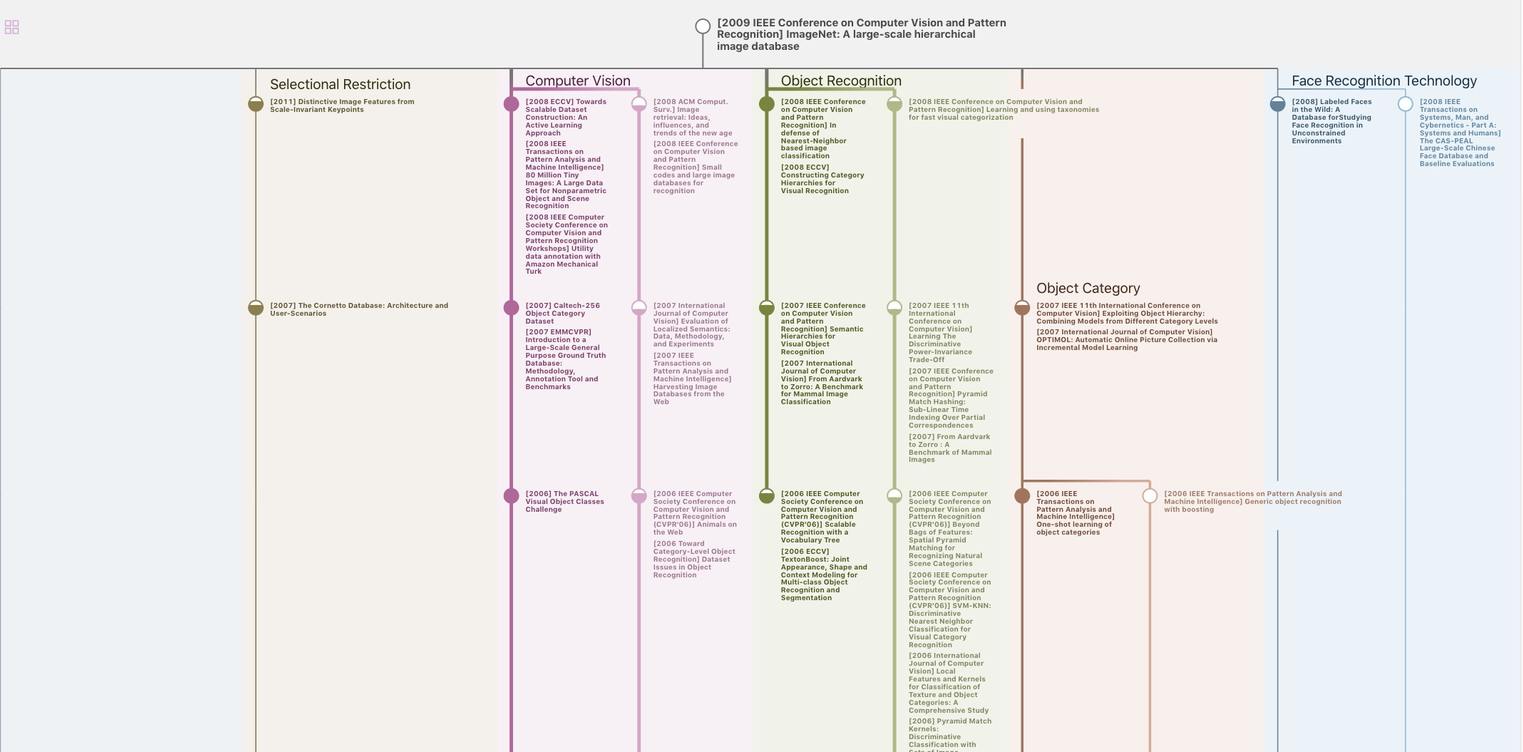
生成溯源树,研究论文发展脉络
Chat Paper
正在生成论文摘要