Health Change Detection Using Temporal Transductive Learning
MACHINE LEARNING, OPTIMIZATION, AND DATA SCIENCE (LOD 2021), PT II(2022)
摘要
Industrial equipment, devices and patients typically undergo change from a healthy state to an unhealthy state. We develop a novel approach to detect unhealthy entities and also discover the time of change to enable deeper investigation into the cause for change. In the absence of an engineering or medical intervention, health degradation only happens in one direction - healthy to unhealthy. Our transductive learning framework, known as max-margin temporal transduction (MMTT), leverages this chronology of observations for learning a superior model with minimal supervision. Temporal Transduction is achieved by incorporating chronological constraints in the conventional max-margin classifier - Support Vector Machines (SVM). We utilize stochastic gradient descent to solve the resulting optimization problem. We prove that with high probability, an epsilon-accurate solution for the proposed model can be achieved in O (1/lambda epsilon) iterations. The runtime is O (1/lambda epsilon) for the linear kernel and O (n/lambda epsilon) for a non-linear Mercer kernel, where n is the number of observations from all entities-labeled and unlabeled. Our experiments on publicly available benchmark datasets demonstrate the effectiveness of our approach in accurately detecting unhealthy entities with less supervision as compared to other strong baselines - conventional and transductive SVM.
更多查看译文
关键词
health,learning,change
AI 理解论文
溯源树
样例
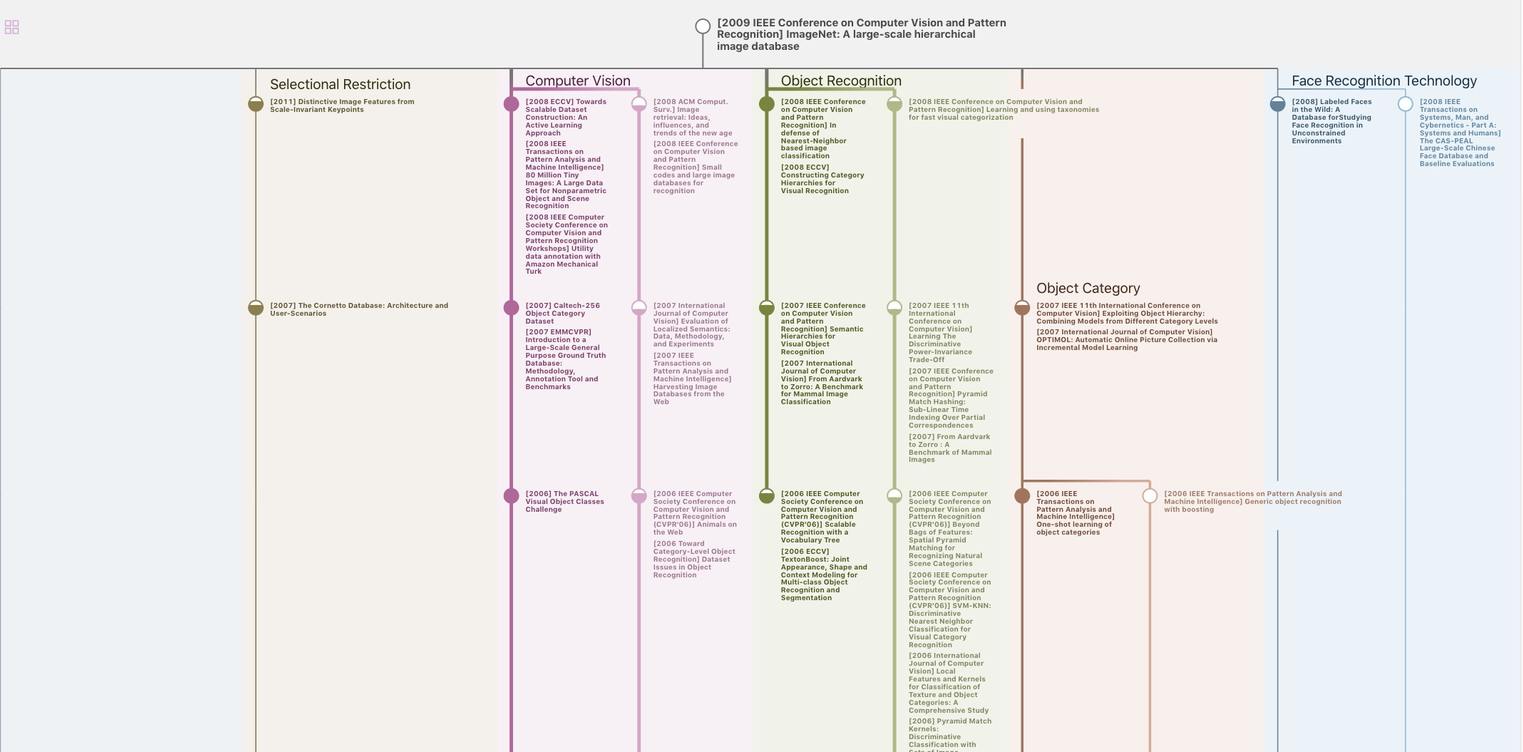
生成溯源树,研究论文发展脉络
Chat Paper
正在生成论文摘要