Multi-Asset Market Making via Multi-Task Deep Reinforcement Learning
MACHINE LEARNING, OPTIMIZATION, AND DATA SCIENCE (LOD 2021), PT II(2022)
摘要
Market making (MM) is a trading activity by an individual market participant or a member firm of an exchange that buys and sells same securities with the primary goal of profiting on the bid-ask spread, which contributes to the market liquidity. Reinforcement learning (RL) is emerging as a quite popular method for automated market making, in addition to many other financial problems. The current state of the art in MM based on RL includes two recent benchmarks which use temporal-difference learning with Tile-Codings and Deep Q Networks (DQN). These two benchmark approaches focus on single-asset modelling, limiting their applicability in realistic scenarios, where the MM agents are required to trade on a collection of assets. Moreover, the Multi-Asset trading reduces the risk associated with the returns. Therefore, we design a Multi-Asset Market Making (MAMM) model, known as MTDRLMM, based on Multi-Task Deep RL. From a Multi-Task Learning perspective, multiple assets are considered as multiple tasks of the same nature. These assets share common characteristics among them, along with their individual traits. The experimental results show that the MAMM is more profitable than Single-Asset MM, in general. Moreover, the MTDRLMM model achieves the state-of-the-art in terms of investment return in a collection of assets.
更多查看译文
关键词
Multi-Asset Market Making, Multi-Task Deep Reinforcement Learning, Tile-Codings, Deep Q Networks
AI 理解论文
溯源树
样例
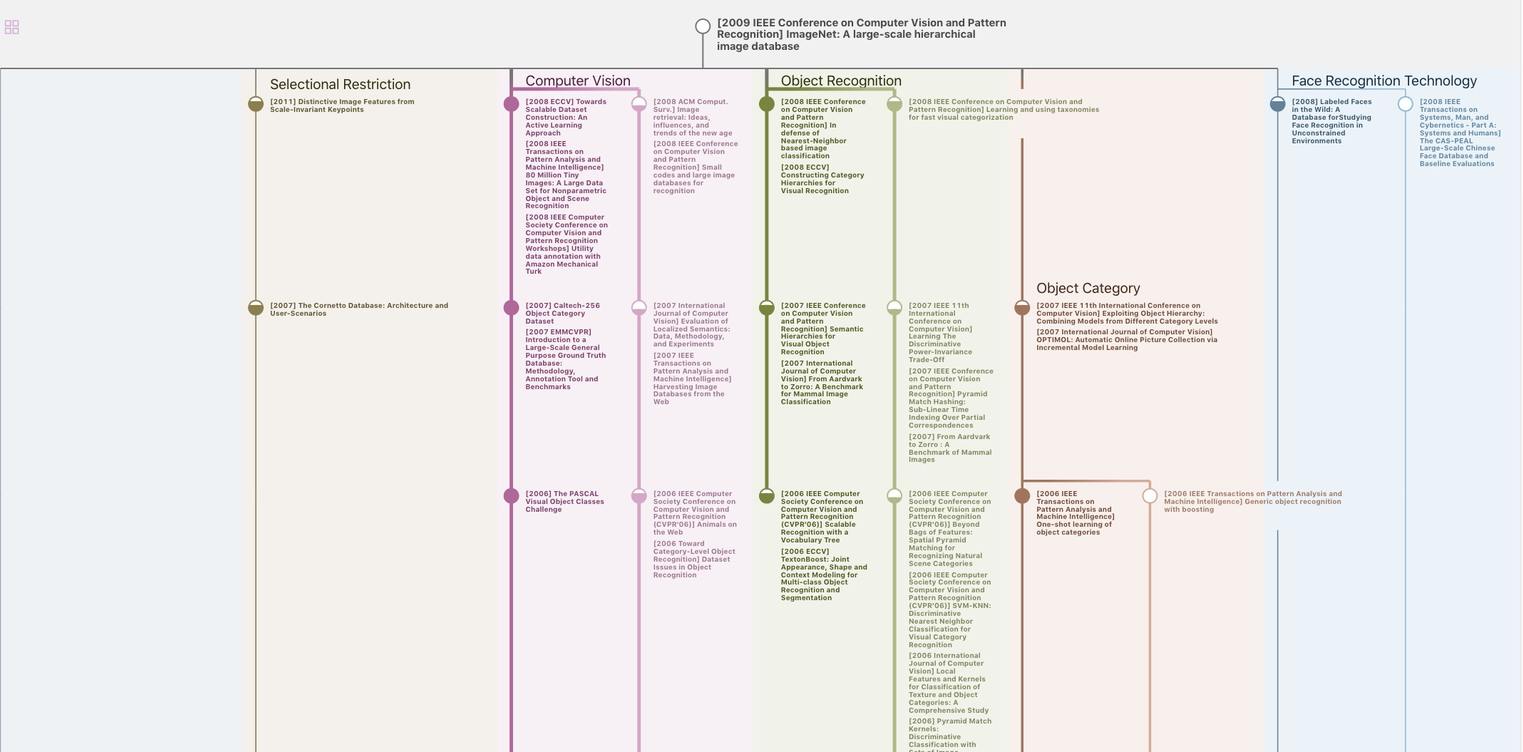
生成溯源树,研究论文发展脉络
Chat Paper
正在生成论文摘要