Improving Convolutional Networks with Boosting Attention Convolutions
ICME(2021)
摘要
Convolutional neural networks (CNNs) have been widely used in a range of tasks because of its robust convolutional feature transformation ability. In this paper, we propose a novel type of convolution called Boosting Attention Convolution (BAC) to improve the basic convolutional feature transformation process of CNNs. The proposed method is designed based on two principles, boosting and attention mechanism. Specifically, we design a set of simple yet effective Boosting Attention Modules (BAM) within grouped convolution, which progressively recalibrate distribution of feature map and enable the future filters nested in a convolution layer to focus more on the feature regions that are unactivated by previous filters. Thus, it can help CNNs generate more discriminative representations by explicitly incorporating richer information. The experimental results on various datasets verify that BAC outperforms state-of-the-art methods. More importantly, the proposed BAC is a general convolution that can be deployed to various modern networks without introducing much parameters and computational complexity.
更多查看译文
关键词
CNNs,boosting,attention mechanism,convolutional filter
AI 理解论文
溯源树
样例
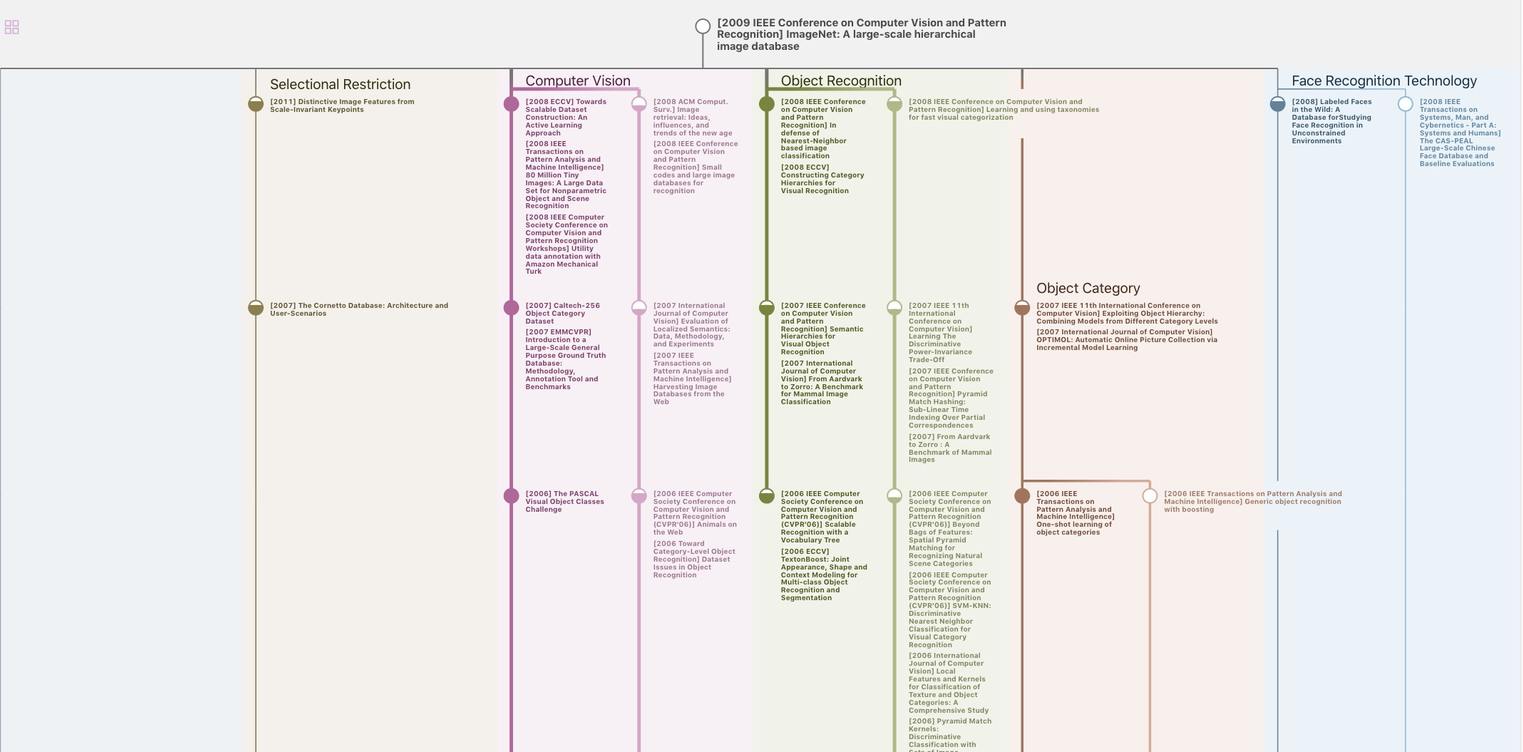
生成溯源树,研究论文发展脉络
Chat Paper
正在生成论文摘要