Rate-Distortion Optimized Hierarchical Deep Feature Compression.
ICME(2021)
摘要
Deep learning has proved to be a vital tool for many computer vision applications. In a distributed environment, an efficient way to analyze the sensed visual information is to extract features for deep learning and then transmit the features to powerful processing hubs for further analysis. This demands for efficient compression of the feature maps before transmission. This paper proposes a hierarchical framework for the compression of deep features from the key frames of a video. First, a feature selection strategy is introduced to reduce the redundancy among different features. The selected features are then quantized, repacked as videos, and further compressed using a standardized video encoder. Furthermore, a rate-distortion optimization module is integrated in the proposed framework to enhance the quality of deep-learning-based computer vision tasks under rate constraints.
更多查看译文
关键词
Compression,deep learning,computer vision,edge computing,rate-distortion optimization
AI 理解论文
溯源树
样例
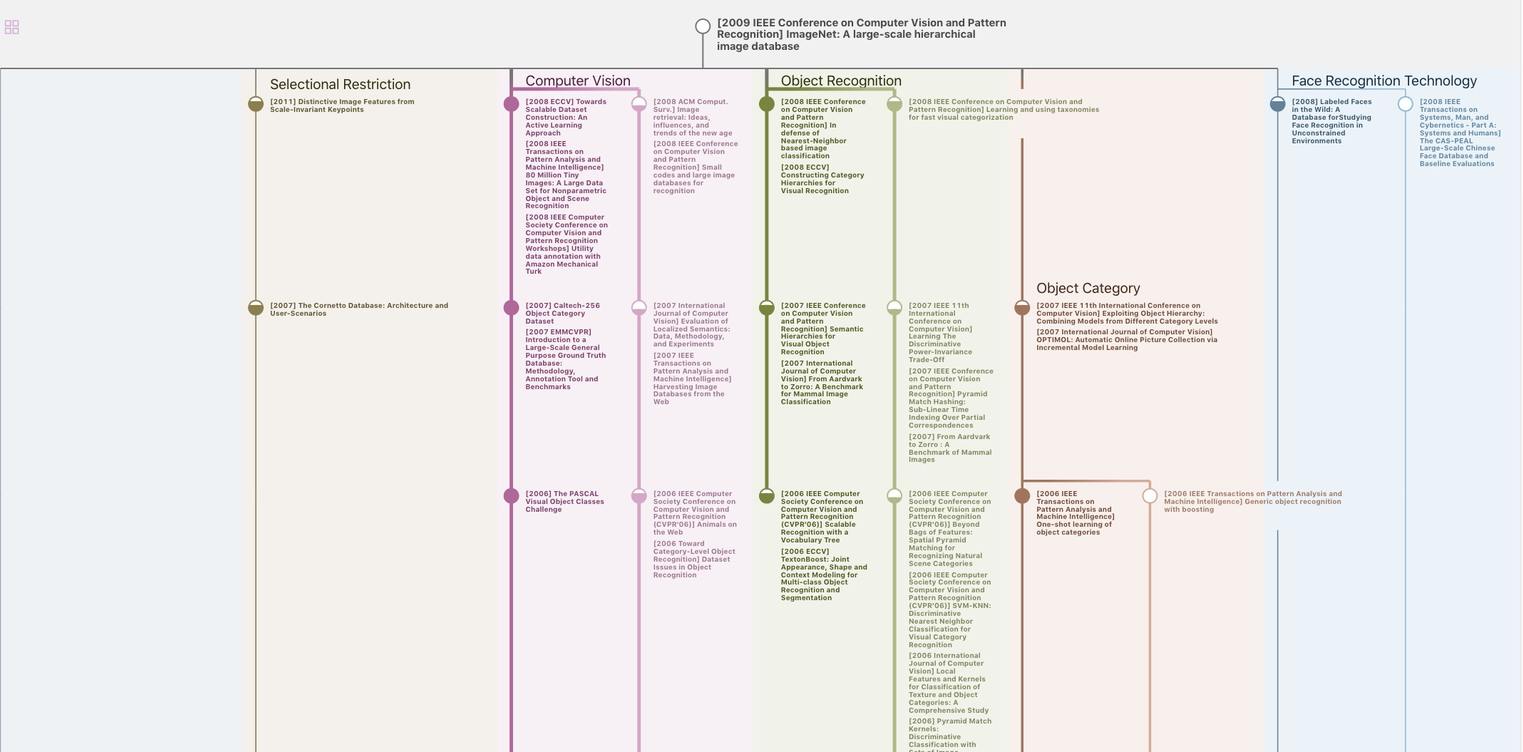
生成溯源树,研究论文发展脉络
Chat Paper
正在生成论文摘要