Dual Contrastive Universal Adaptation Network.
ICME(2021)
摘要
We study Universal Domain Adaptation (UniDA) problem, which is recently proposed. Different from existing domain adaptation (DA) methods, e.g., Closed set, Open set and Partial DA, UniDA does not need any prior knowledge about the overlap across the source and target label sets. We have two challenges in UniDA problem: i) Domain shift. ii) Category shift. Towards tackling above challenges, we formulate a universal adaptation network called Dual Contrastive Network (DCN), where a contrastive module and a transferability rule are included. The experimental results reveal that DCN can work stably on different UniDA settings and exceeds the state-of-the-art performance across five benchmarks against existing DA methods.
更多查看译文
关键词
Universal Domain Adaptation,image classification,contrastive learning
AI 理解论文
溯源树
样例
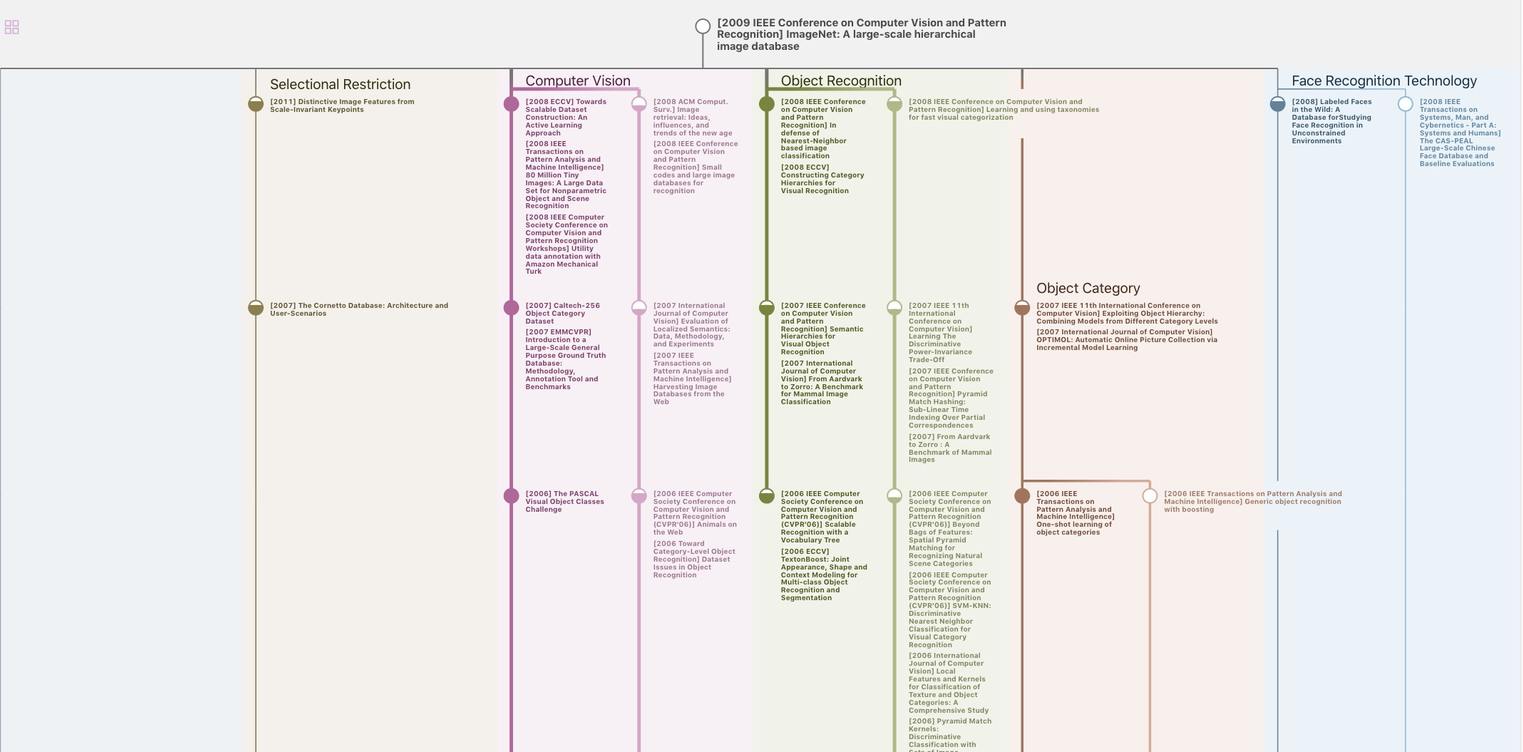
生成溯源树,研究论文发展脉络
Chat Paper
正在生成论文摘要