A federated learning based semi-supervised credit prediction approach enhanced by multi-layer label mean
WEB INTELLIGENCE(2021)
摘要
Learning based credit prediction has attracted great interest from academia and industry. Different institutions hold a certain amount of credit data with limited users to build model. An institution has the requirement to obtain data from other institutions for improving model performance. However, due to the privacy protection and subject to legal restrictions, they encounter difficulties in data exchange. This affects the performance of credit prediction. In order to solve the above problem, this paper proposes a federated learning based semi-supervised credit prediction approach enhanced by multi-layer label mean, which can aggregate parameters of each institution via joint training while protecting the data privacy of each institution. Moreover, in actual production and life, there are usually more unlabeled credit data than labeled ones, and the distribution of their feature space presents multiple data-dense divisions. To deal with these, local meanNet model is proposed with a multi-layer label mean based semi-supervised deep learning network. In addition, this paper introduces a cost-sensitive loss function in the supervised part of the local mean model. Conducted on two public credit datasets, experimental results show that our proposed federated learning based approach has achieved promising credit prediction performance in terms of Accuracy and F1 measures. At the same time, the framework design mode that splits data aggregation and keys uniformly can improve the security of data privacy and enhance the flexibility of model training.
更多查看译文
关键词
Federated learning, credit prediction, label mean, semi-supervised deep learning
AI 理解论文
溯源树
样例
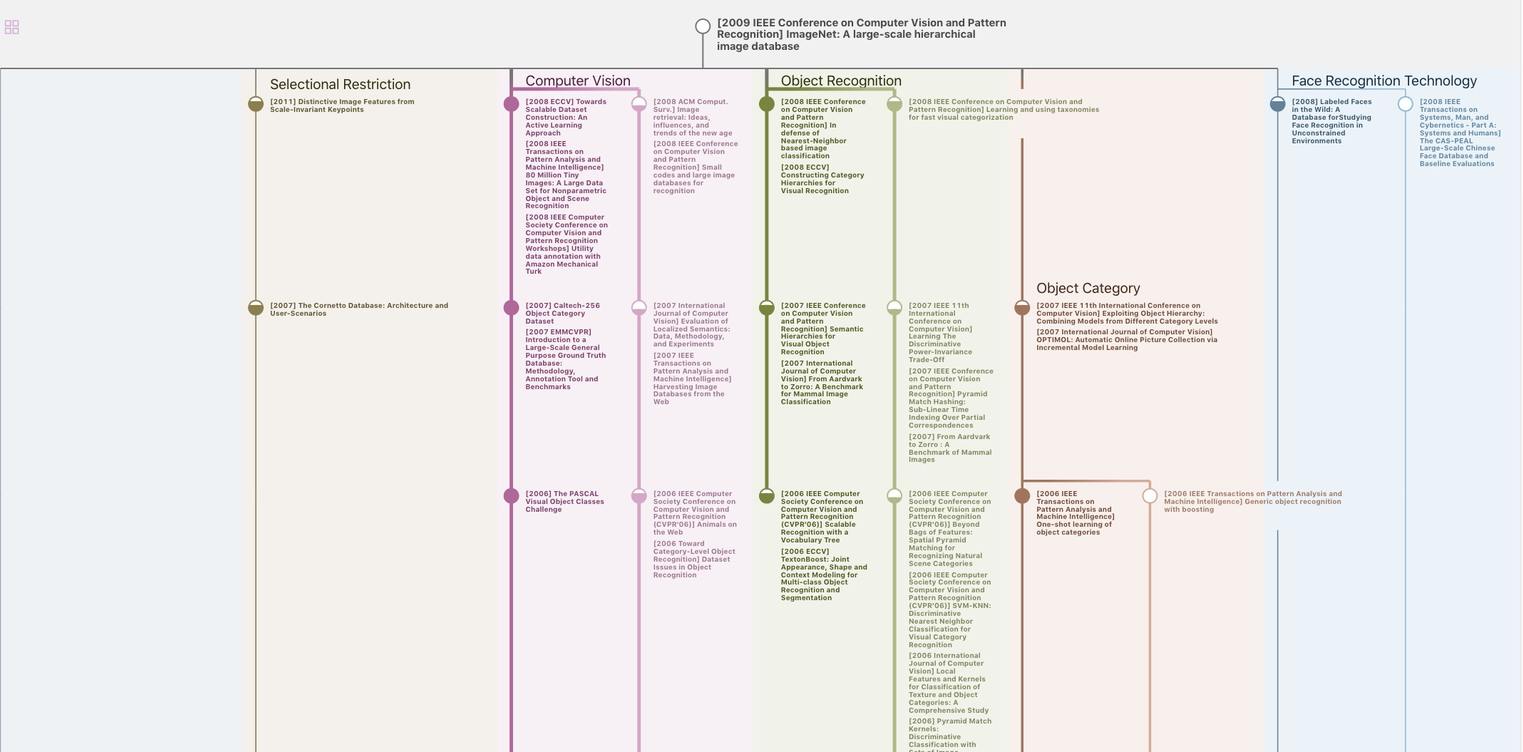
生成溯源树,研究论文发展脉络
Chat Paper
正在生成论文摘要