Augmenting training data with syntactic phrasal-segments in low-resource neural machine translation
MACHINE TRANSLATION(2021)
摘要
Neural machine translation (NMT) has emerged as a preferred alternative to the previous mainstream statistical machine translation (SMT) approaches largely due to its ability to produce better translations. The NMT training is often characterized as data hungry since a lot of training data, in the order of a few million parallel sentences, is generally required. This is indeed a bottleneck for the under-resourced languages that lack the availability of such resources. The researchers in machine translation (MT) have tried to solve the problem of data sparsity by augmenting the training data using different strategies. In this paper, we propose a generalized linguistically motivated data augmentation approach for NMT taking low-resource translation into consideration. The proposed method operates by generating source—target phrasal segments from an authentic parallel corpus, whose target counterparts are linguistic phrases extracted from the syntactic parse trees of the target-side sentences. We augment the authentic training corpus with the parser generated phrasal-segments, and investigate the efficacy of our proposed strategy in low-resource scenarios. To this end, we carried out experiments with resource-poor language pairs, viz. Hindi-to-English, Malayalam-to-English, and Telugu-to-English, considering the three state-of-the-art NMT paradigms, viz. attention-based recurrent neural network (Bahdanau et al., 2015 ), Google Transformer (Vaswani et al. 2017 ) and convolution sequence-to-sequence (Gehring et al. 2017 ) neural network models. The MT systems built on the training data prepared with our data augmentation strategy significantly surpassed the state-of-the-art NMT systems with large margins in all three translation tasks. Further, we tested our approach along with back-translation (Sennrich et al. 2016a ), and found these to be complementary to each other. This joint approach has turned out to be the best-performing one in our low-resource experimental settings.
更多查看译文
关键词
Neural machine translation, Low-resource neural machine translation, Data augmentation, Syntactic phrase augmentation
AI 理解论文
溯源树
样例
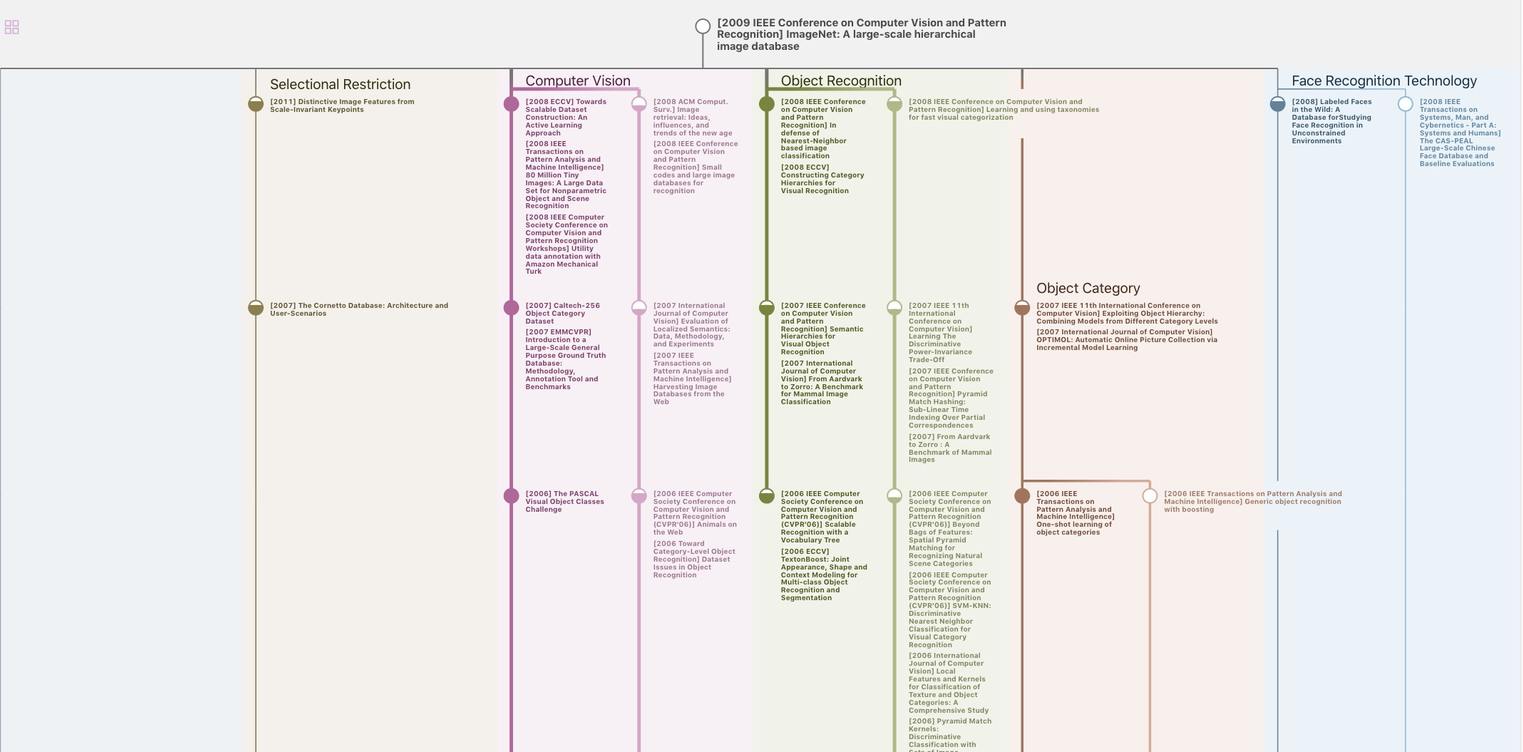
生成溯源树,研究论文发展脉络
Chat Paper
正在生成论文摘要