Enhancing transferability of adversarial examples via rotation-invariant attacks
IET COMPUTER VISION(2022)
摘要
Deep neural networks are vulnerable to adversarial examples. However, existing attacks exhibit relatively low efficacy in generating transferable adversarial examples. Improved transferability to address this issue is proposed via a rotation-invariant attack method that maximizes the loss function w.r.t the random rotated image instead of the original input at each iteration, thus mitigating the high correlation between the adversarial examples and the source models and making the adversarial examples more transferable. Extensive experiments show that the proposed method can significantly improve the transferability of the adversarial examples with almost no extra computational cost and can be integrated into various methods. In addition, when this method is easily applied through a plug-in, the average attack success rate against six robustly trained models increases by 5.4% over the state-of-the-art baseline method, demonstrating its effectiveness and efficiency. The codes used are publicly available at .
更多查看译文
关键词
computer vision,deep learning (artificial intelligence),computer crime,iterative methods
AI 理解论文
溯源树
样例
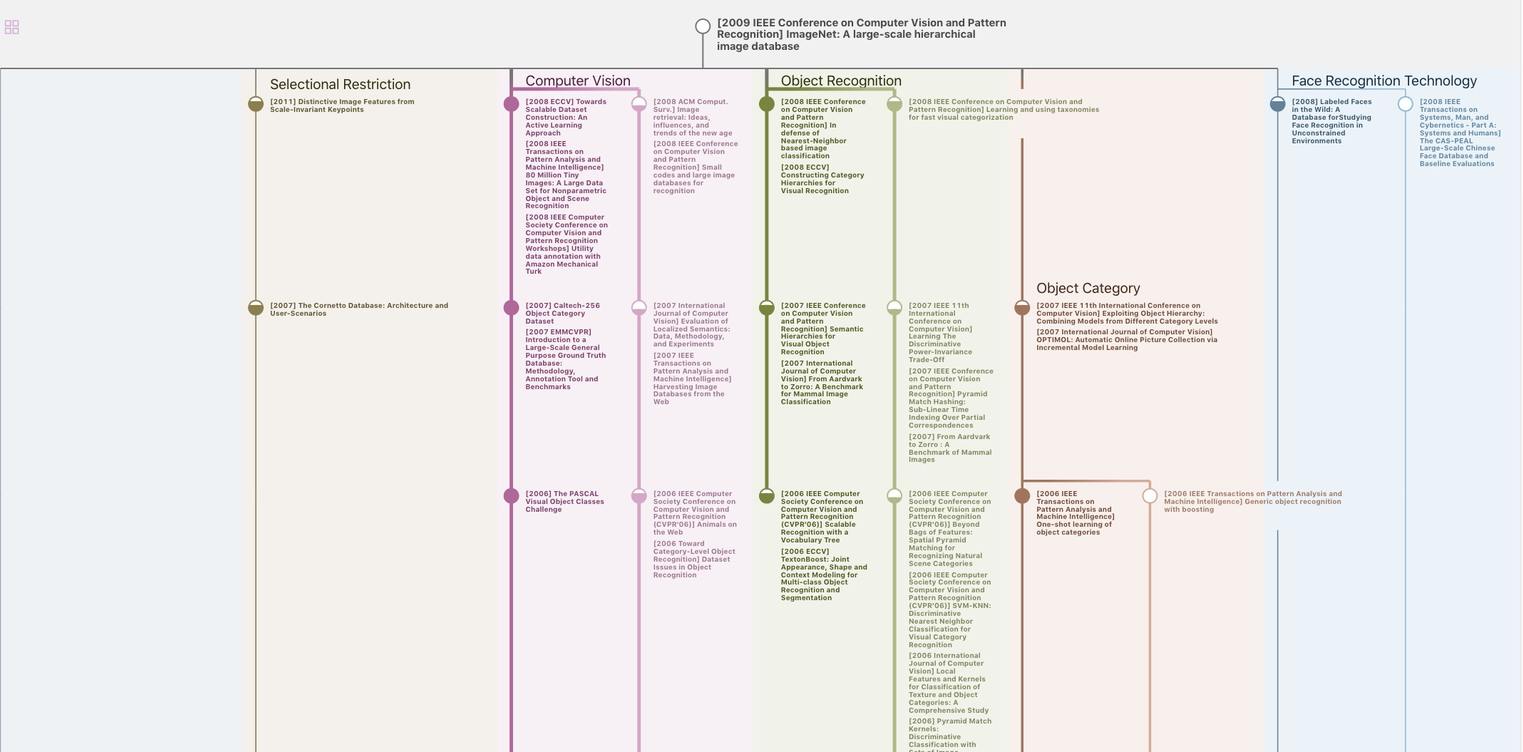
生成溯源树,研究论文发展脉络
Chat Paper
正在生成论文摘要