Robust high-dimensional screening
Environmental Modelling & Software(2022)
摘要
Global sensitivity analysis (GSA) is a valuable tool for filtering out non-influential model inputs. In combination with robustness, convergence and validation analyses, GSA can be particularly beneficial in interpreting and simplifying models with tens of thousands of independent inputs. However, there is lack of research on robust screening of such large models, where the curse of dimensionality can make existing analyses obsolete. We aim to close this gap by evaluating the computational performance of Spearman rank correlation coefficients, Sobol and delta indices, and gradient boosted trees regression. Numerical experiments are conducted for the Morris test function and a life cycle assessment model with 10'000 inputs each. Our results enable us to recommend a standardized procedure for higher-dimensional models which efficiently tests for model linearity, GSA screening, and convergence and robustness analyses of sensitivity indices, screening and rankings.
更多查看译文
关键词
Global sensitivity analysis,High-dimensional models,Robustness,Convergence,Validation,Life cycle assessment,Environmental impact assessment
AI 理解论文
溯源树
样例
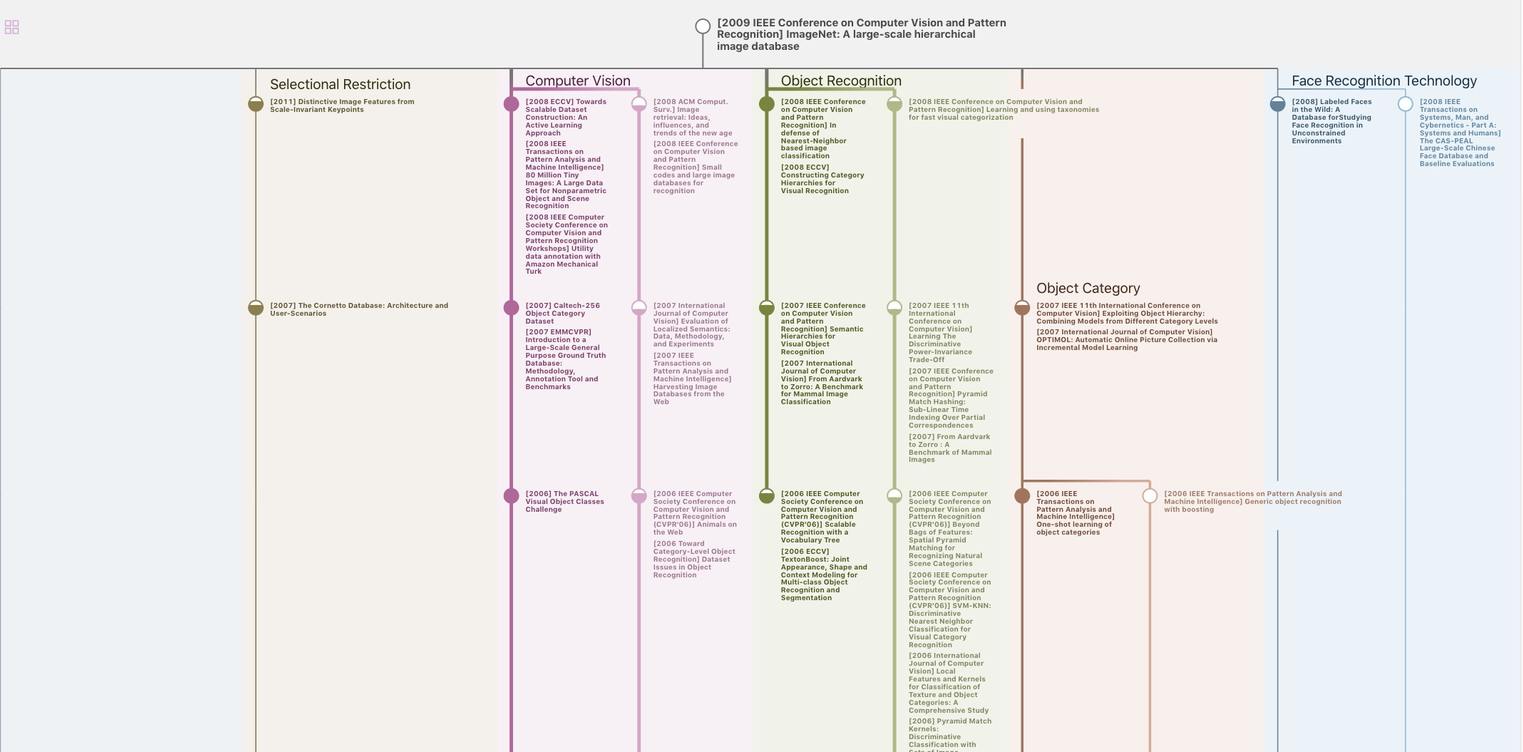
生成溯源树,研究论文发展脉络
Chat Paper
正在生成论文摘要