An L₁-Norm Based Optimization Method for Sparse Redundancy Resolution of Robotic Manipulators
IEEE Transactions on Circuits and Systems II: Express Briefs(2022)
摘要
For targeted motion control tasks of manipulators, it is frequently necessary to make use of full levels of joint actuation to guarantee successful motion planning and path tracking. Such way of motion planning and control may keep the joint actuation in a non-sparse manner during motion control process. In order to improve sparsity of joint actuation for manipulator systems, a novel motion planning scheme which can optimally and sparsely adopt joint actuation is proposed in this brief. The proposed motion planning strategy is formulated as a constrained
$L_{1}$
norm optimization problem, and an equivalent enhanced optimization solution dealing with bounded joint velocity is proposed as well. A new primal dual neural network with a new solution set division is further proposed and applied to solve such bounded optimization which can sparsely adopt joint actuation for motion control. Simulation and experiment results demonstrate the efficiency, accuracy and superiority of the proposed method for optimally and sparsely adopting joint actuation. The average sparsity (i.e., -
$\|\dot \theta \|_{p}$
where
$\theta $
denotes the joint angle) of the joint motion of the manipulator can be increased by 39.22% and 51.30% for path tracking tasks in
$X - Y$
and
$X - Z$
planes respectively, indicating that the sparsity of joint actuation can be enhanced.
更多查看译文
关键词
Redundancy resolution,dynamic neural network,kinematic control,sparsity
AI 理解论文
溯源树
样例
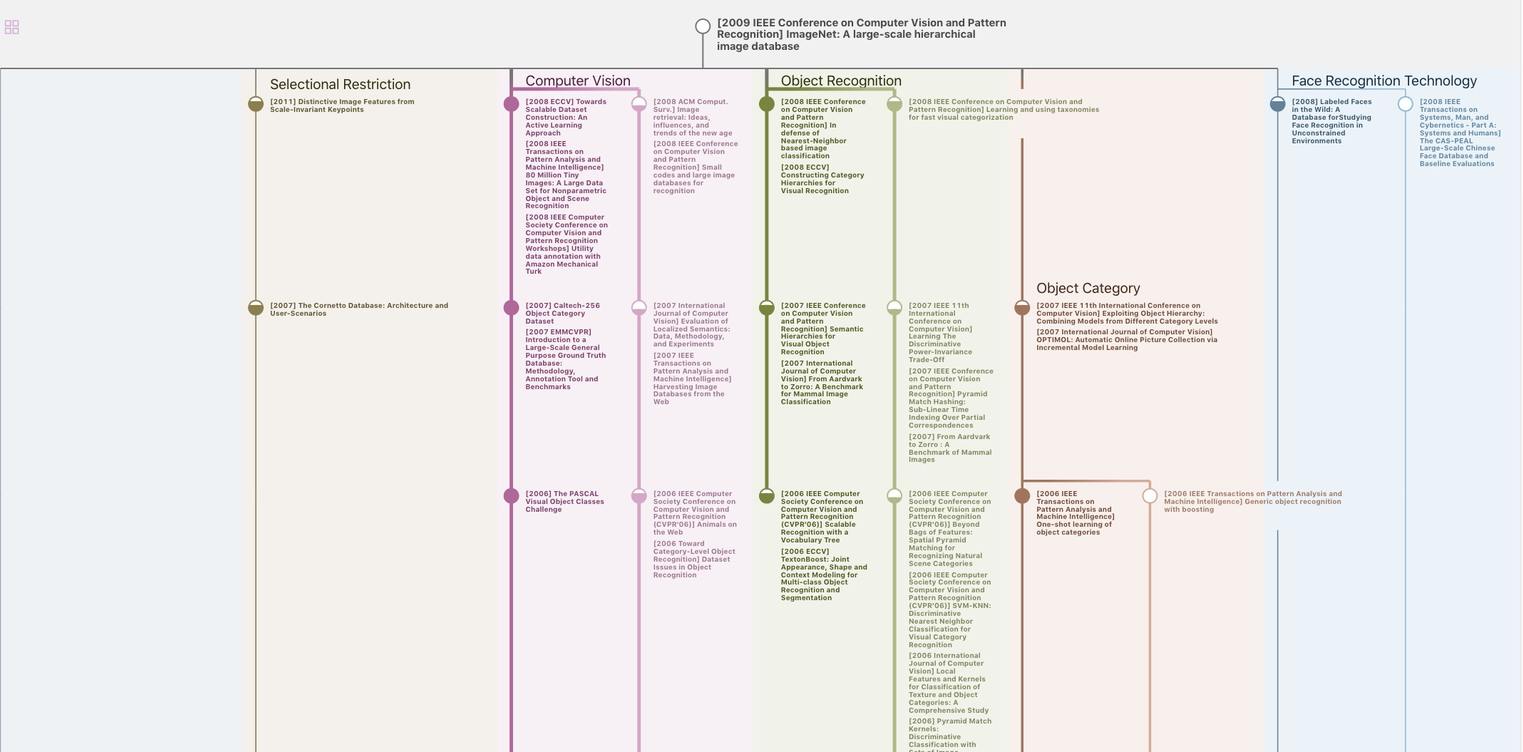
生成溯源树,研究论文发展脉络
Chat Paper
正在生成论文摘要