Evolved Extended Kalman Filter for first-order dynamical systems with unknown measurements noise covariance
APPLIED SOFT COMPUTING(2022)
摘要
The present work focuses on an open problem in the design of Extended Kalman filters: the lack of knowledge of the measurement noise covariance. A novel extension of the analytic behaviors framework, which integrates a theoretical formulation and evolutionary computing, has been introduced as a design methodology for the construction of this unknown parameter. The proposed methodology is developed and applied for the design of Evolved Extended Kalman Filters for nonlinear first-order dynamical systems. The proposed methodology applies an offline evolutionary synthesis of analytic nonlinear functions, to be used as measurement noise covariance, aiming to minimize the Kalman criterion. The virtues of the methodology are exemplified through a complex, highly nonlinear, firstorder dynamical system, for which 2649 optimized replacements of the measurement noise covariance are found. Under different scenarios, the performance of the Evolved Extended Kalman Filter with unknown measurement noise covariance is compared with that of the conventional Extended Kalman Filter where the measurement noise covariance is known. The robustness of the Evolved Extended Kalman Filter is demonstrated through numerical evaluation.
更多查看译文
关键词
Extended Kalman Filter, Analytic behaviors, Genetic programming, Nonlinear first-order dynamical systems, Logistic map system
AI 理解论文
溯源树
样例
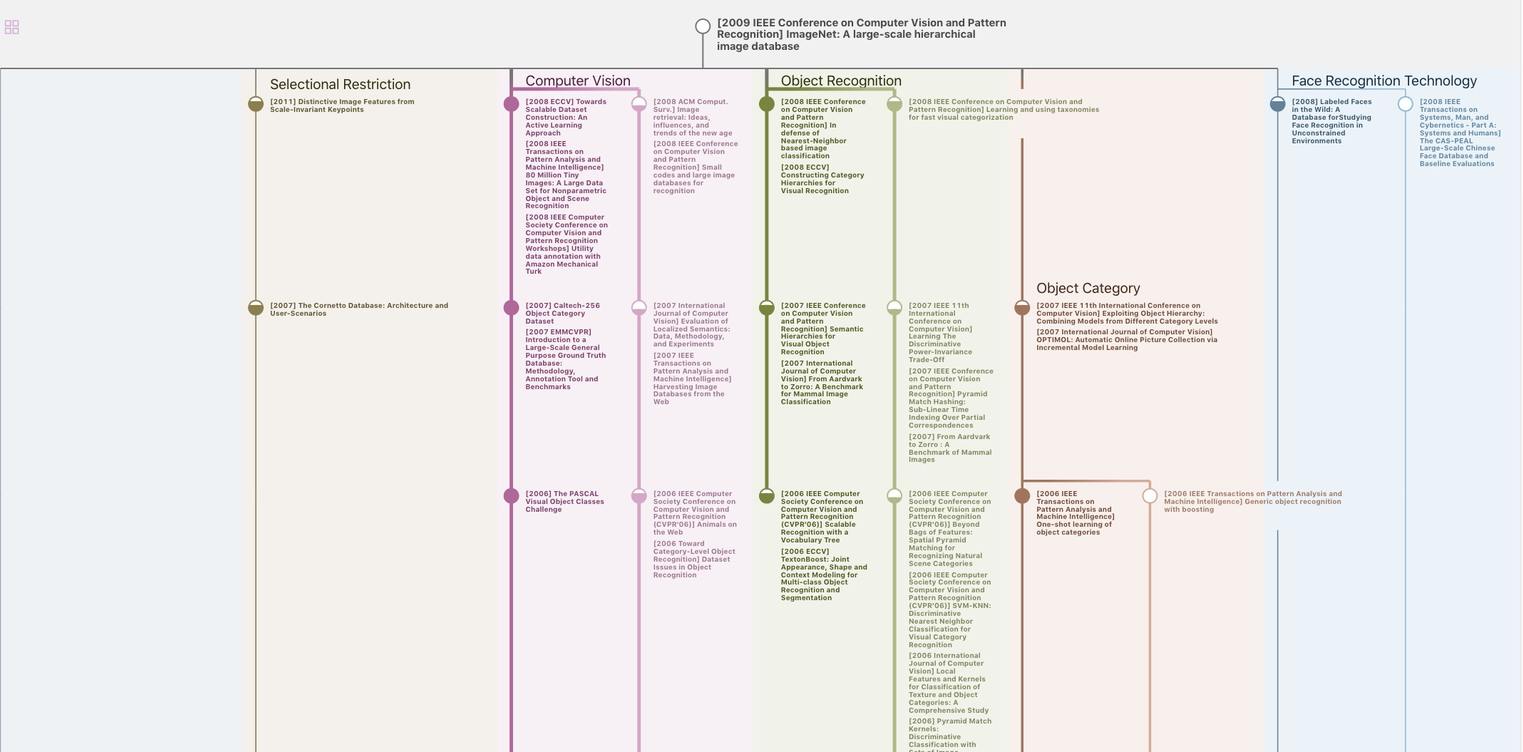
生成溯源树,研究论文发展脉络
Chat Paper
正在生成论文摘要