Kidney tumor segmentation from computed tomography images using DeepLabv3+ 2.5D model
Expert Systems with Applications(2022)
摘要
Kidney cancer is a public health problem that affects thousands of people worldwide. Accurate kidney tumor segmentation is an important task that helps doctors to reduce the time to interpret images, diagnose, and plan the treatment of the diseases. However, because of the heterogeneity of tumors, manually segmenting is a time-consuming process and subject to variability among specialists. In the last years, convolutional neural networks (CNNs) have gained popularity for automatic medical image segmentation. Three-dimensional (3D) networks have a high segmentation capacity, but they are complex and have high computational costs. Thus, two-dimensional networks are the most used owing to the relatively low memory consumption, but they do not exploit 3D features. Therefore, in the present study, a 2.5D network that balances memory consumption and model complexity is proposed to assist doctors specialized in kidney tumor diagnosis in computed tomography (CT). There are three major steps in the proposed method: (1) preprocessing of the KiTS19 dataset, (2) initial segmentation using the DeepLabv3+ 2.5D model with DPN-131 encoder, and (3) reduction of false positives using image processing. The performance of the proposed method was assessed in 210 CTs from the available KiTS19 dataset. In the best scenario, the proposed method presented accuracy of 99.71%, sensitivity of 84.24%, specificity of 99.82%, Dice coefficient of 85.17%, Jaccard index of 75.62%, Hausdorff distance of 18.39 mm, and average surface distance of 3.36 mm. Thus, the 2.5D neural network approach is capable of efficiently solving the problem of segmentation of kidney tumors in CT images, presenting results comparable to those of high-performance 3D neural networks.
更多查看译文
关键词
Computed tomography,Deep learning,Kidney cancer,Kidney tumor segmentation,Medical images
AI 理解论文
溯源树
样例
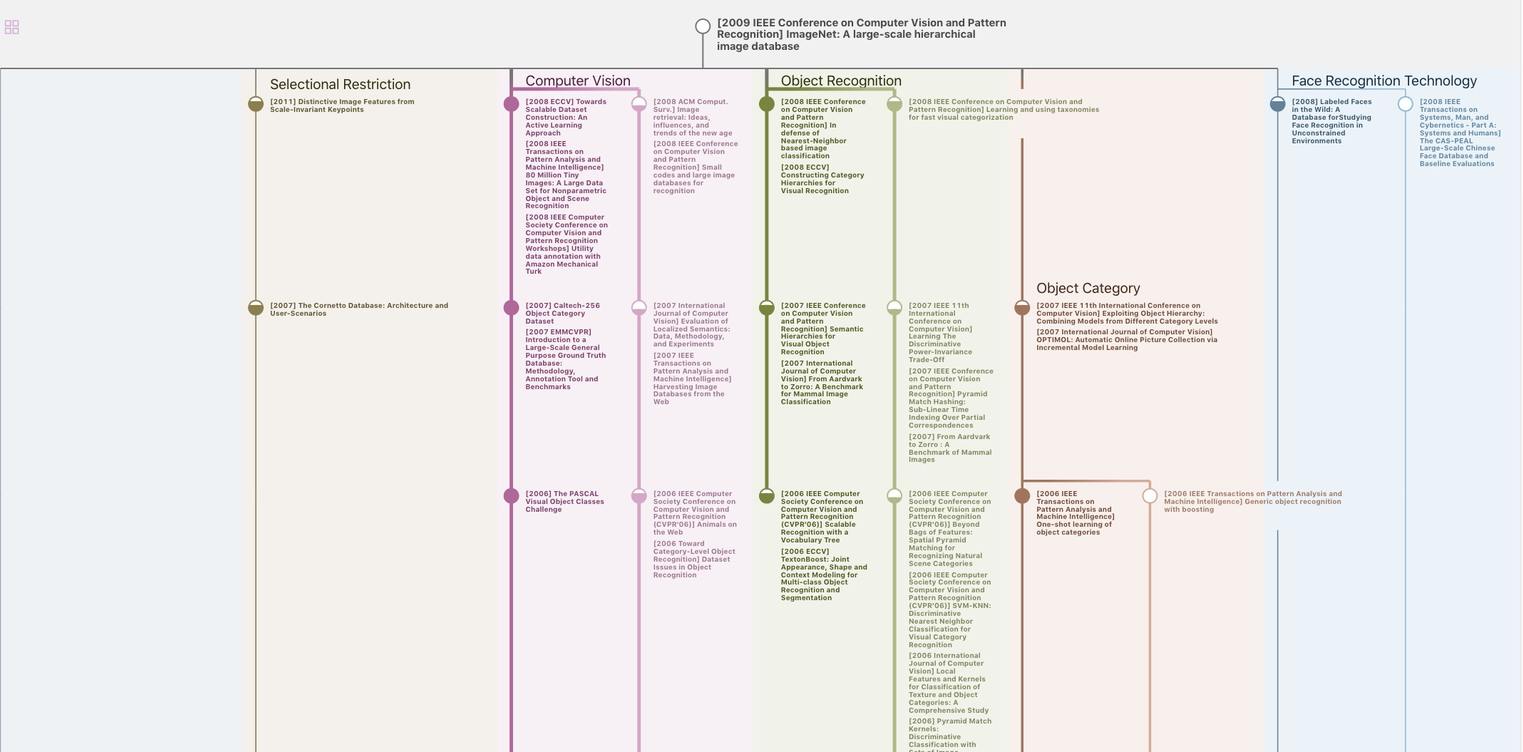
生成溯源树,研究论文发展脉络
Chat Paper
正在生成论文摘要