A fast supervised density-based discretization algorithm for classification tasks in the medical domain
HEALTH INFORMATICS JOURNAL(2022)
摘要
Discretization is a preprocessing technique used for converting continuous features into categorical. This step is essential for processing algorithms that cannot handle continuous data as input. In addition, in the big data era, it is important for a discretizer to be able to efficiently discretize data. In this paper, a new supervised density-based discretization (DBAD) algorithm is proposed, which satisfies these requirements. For the evaluation of the algorithm, 11 datasets that cover a wide range of datasets in the medical domain were used. The proposed algorithm was tested against three state-of-the art discretizers using three classifiers with different characteristics. A parallel version of the algorithm was evaluated using two synthetic big datasets. In the majority of the performed tests, the algorithm was found performing statistically similar or better than the other three discretization algorithms it was compared to. Additionally, the algorithm was faster than the other discretizers in all of the performed tests. Finally, the parallel version of DBAD shows almost linear speedup for a Message Passing Interface (MPI) implementation (9.64x for 10 nodes), while a hybrid MPI/OpenMP implementation improves execution time by 35.3x for 10 nodes and 6 threads per node.
更多查看译文
关键词
big data, classification, density-based discretization, density estimation, supervised discretization
AI 理解论文
溯源树
样例
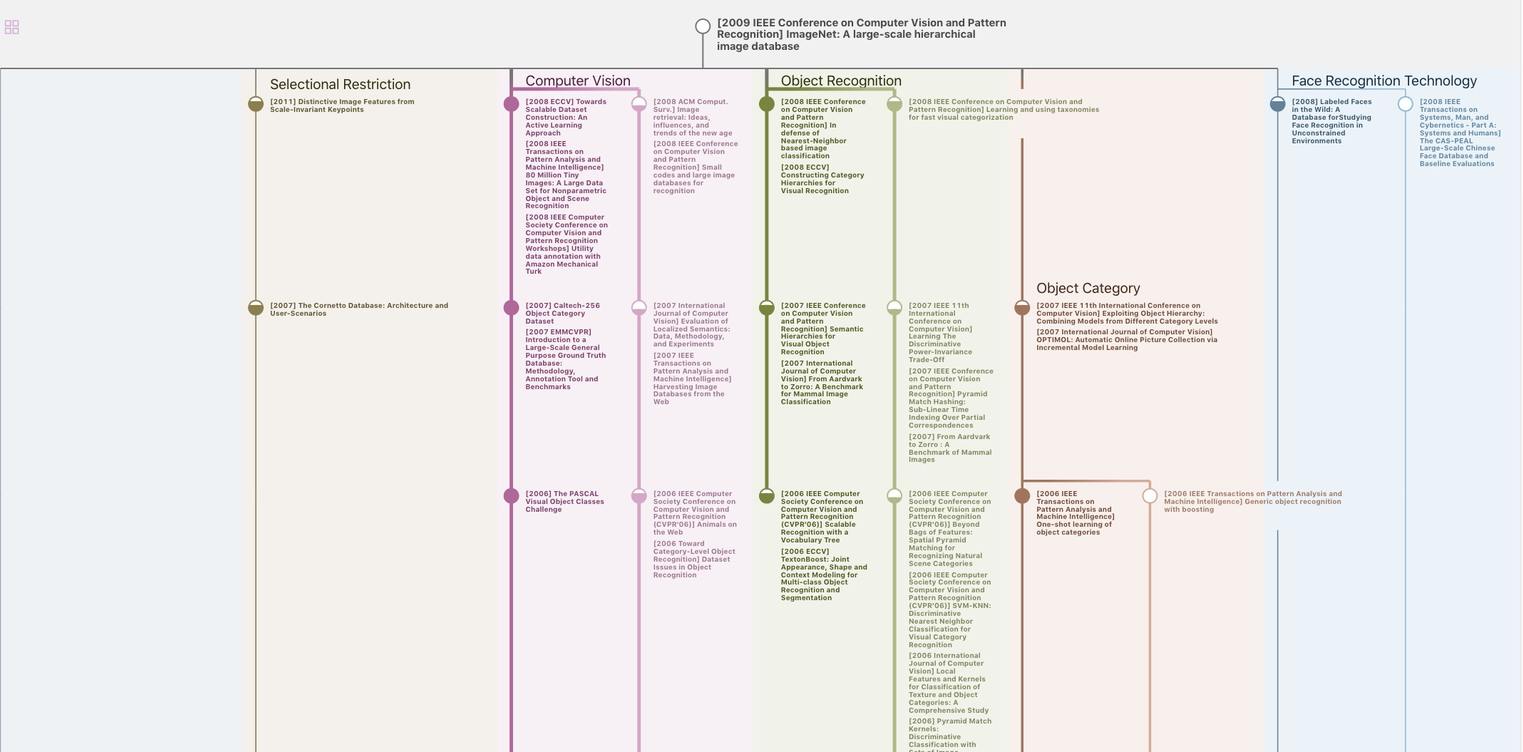
生成溯源树,研究论文发展脉络
Chat Paper
正在生成论文摘要