The C-SHIFT Algorithm for Normalizing Covariances
IEEE/ACM Transactions on Computational Biology and Bioinformatics(2023)
摘要
Omics technologies are powerful tools for analyzing patterns in gene expression data for thousands of genes. Due to a number of systematic variations in experiments, the raw gene expression data is often obfuscated by undesirable technical noises. Various normalization techniques were designed in an attempt to remove these non-biological errors prior to any statistical analysis. One of the reasons for normalizing data is the need for recovering the covariance matrix used in gene network analysis. In this paper, we introduce a novel normalization technique, called the covariance shift (C-SHIFT) method. This normalization algorithm uses optimization techniques together with the blessing of dimensionality philosophy and energy minimization hypothesis for covariance matrix recovery under additive noise (in biology, known as the bias). Thus, it is perfectly suited for the analysis of logarithmic gene expression data. Numerical experiments on synthetic data demonstrate the method’s advantage over the classical normalization techniques. Namely, the comparison is made with Rank, Quantile, cyclic LOESS (locally estimated scatterplot smoothing), and MAD (median absolute deviation) normalization methods. We also evaluate the performance of C-SHIFT algorithm on real biological data.
更多查看译文
关键词
Normalization methods,C-SHIFT algorithm,gene co-expression networks
AI 理解论文
溯源树
样例
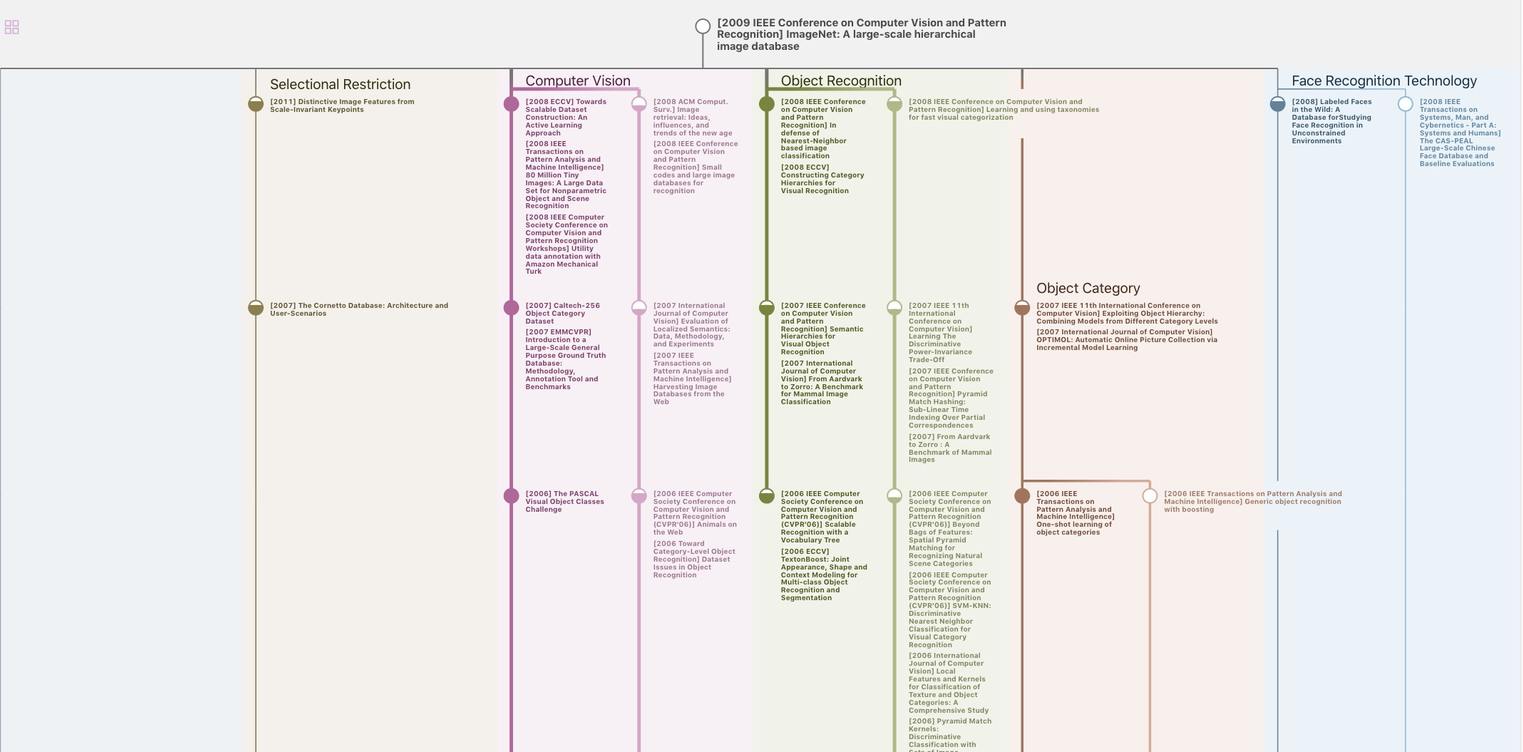
生成溯源树,研究论文发展脉络
Chat Paper
正在生成论文摘要