Flexible-Modal Face Anti-Spoofing: A Benchmark
arxiv(2023)
摘要
Face anti-spoofing (FAS) plays a vital role in securing face recognition systems from presentation attacks. Benefitted from the maturing camera sensors, single-modal (RGB) and multi-modal (e.g., RGB+Depth) FAS has been applied in various scenarios with different configurations of sensors/modalities. Existing single- and multi-modal FAS methods usually separately train and deploy models for each possible modality scenario, which might be redundant and inefficient. Can we train a unified model, and flexibly deploy it under various modality scenarios? In this paper, we establish the first flexible-modal FAS benchmark with the principle ‘train one for all’. To be specific, with trained multi-modal (RGB+Depth+IR) FAS models, both intra- and cross-dataset testings are conducted on four flexible-modal sub-protocols (RGB, RGB+Depth, RGB+IR, and RGB+Depth+IR). We also investigate prevalent deep models and feature fusion strategies for flexible-modal FAS. We hope this new benchmark will facilitate the future research of the multi-modal FAS. The protocols and codes are available at https://github.com/ZitongYu/Flex-Modal-FAS.
更多查看译文
关键词
deploy models,face recognition systems,flexible-modal face anti-spoofing,flexible-modal FAS benchmark,flexible-modal sub-protocols,maturing camera sensors,modality scenarios,multimodal FAS methods,possible modality scenario,prevalent deep models,principle train,RGB+Depth+IR,RGB+IR,trained multimodal FAS models,unified model
AI 理解论文
溯源树
样例
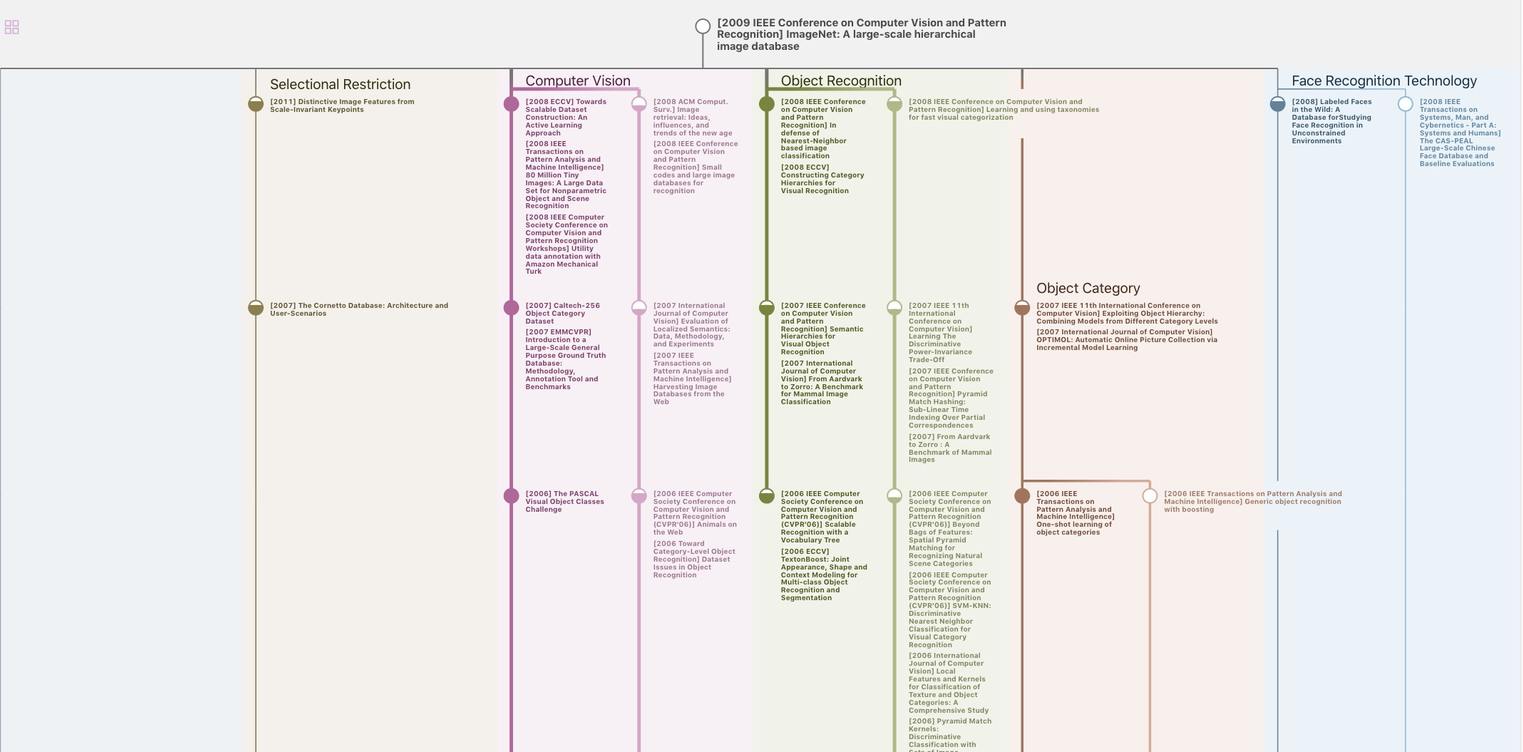
生成溯源树,研究论文发展脉络
Chat Paper
正在生成论文摘要