Construction of Deep ReLU Nets for Spatially Sparse Learning
IEEE transactions on neural networks and learning systems(2023)
摘要
Training an interpretable deep net to embody its theoretical advantages is difficult but extremely important in the community of machine learning. In this article, noticing the importance of spatial sparseness in signal and image processing, we develop a constructive approach to generate a deep net to capture the spatial sparseness feature. We conduct both theoretical analysis and numerical verifications to show the power of the constructive approach. Theoretically, we prove that the constructive approach can yield a deep net estimate that achieves the optimal generalization error bounds in the framework of learning theory. Numerically, we show that the constructive approach is essentially better than shallow learning in the sense that it provides better prediction accuracy with less training time.
更多查看译文
关键词
Deep learning,Neural networks,Training,Spatial resolution,Partitioning algorithms,Signal resolution,Optimization,Constructive deep net (CDN),deep learning,learning theory,spatial sparseness
AI 理解论文
溯源树
样例
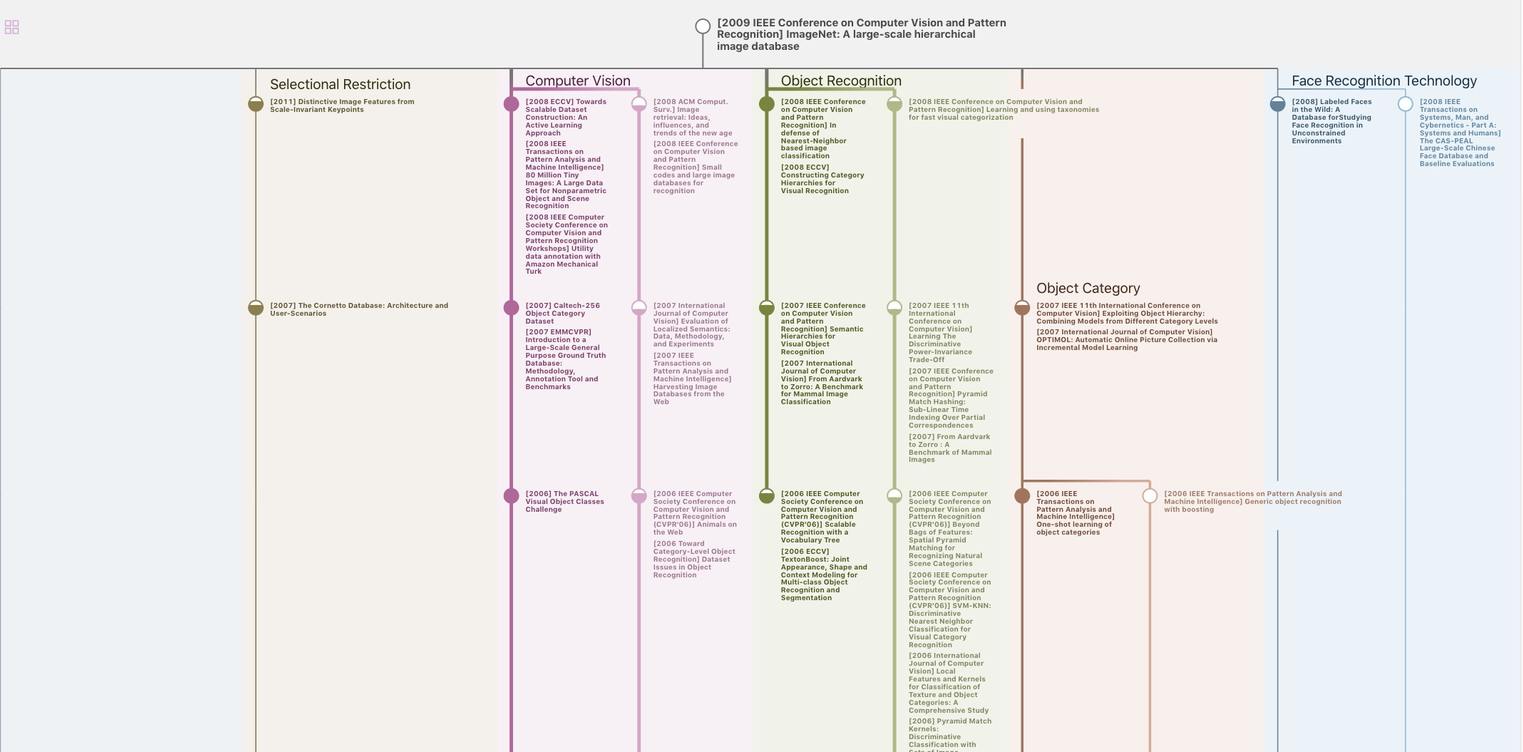
生成溯源树,研究论文发展脉络
Chat Paper
正在生成论文摘要