One Configuration to Rule Them All? Towards Hyperparameter Transfer in Topic Models using Multi-Objective Bayesian Optimization
arxiv(2022)
摘要
Topic models are statistical methods that extract underlying topics from document collections. When performing topic modeling, a user usually desires topics that are coherent, diverse between each other, and that constitute good document representations for downstream tasks (e.g. document classification). In this paper, we conduct a multi-objective hyperparameter optimization of three well-known topic models. The obtained results reveal the conflicting nature of different objectives and that the training corpus characteristics are crucial for the hyperparameter selection, suggesting that it is possible to transfer the optimal hyperparameter configurations between datasets.
更多查看译文
关键词
topic models,hyperparameter transfer,bayesian,optimization,multi-objective
AI 理解论文
溯源树
样例
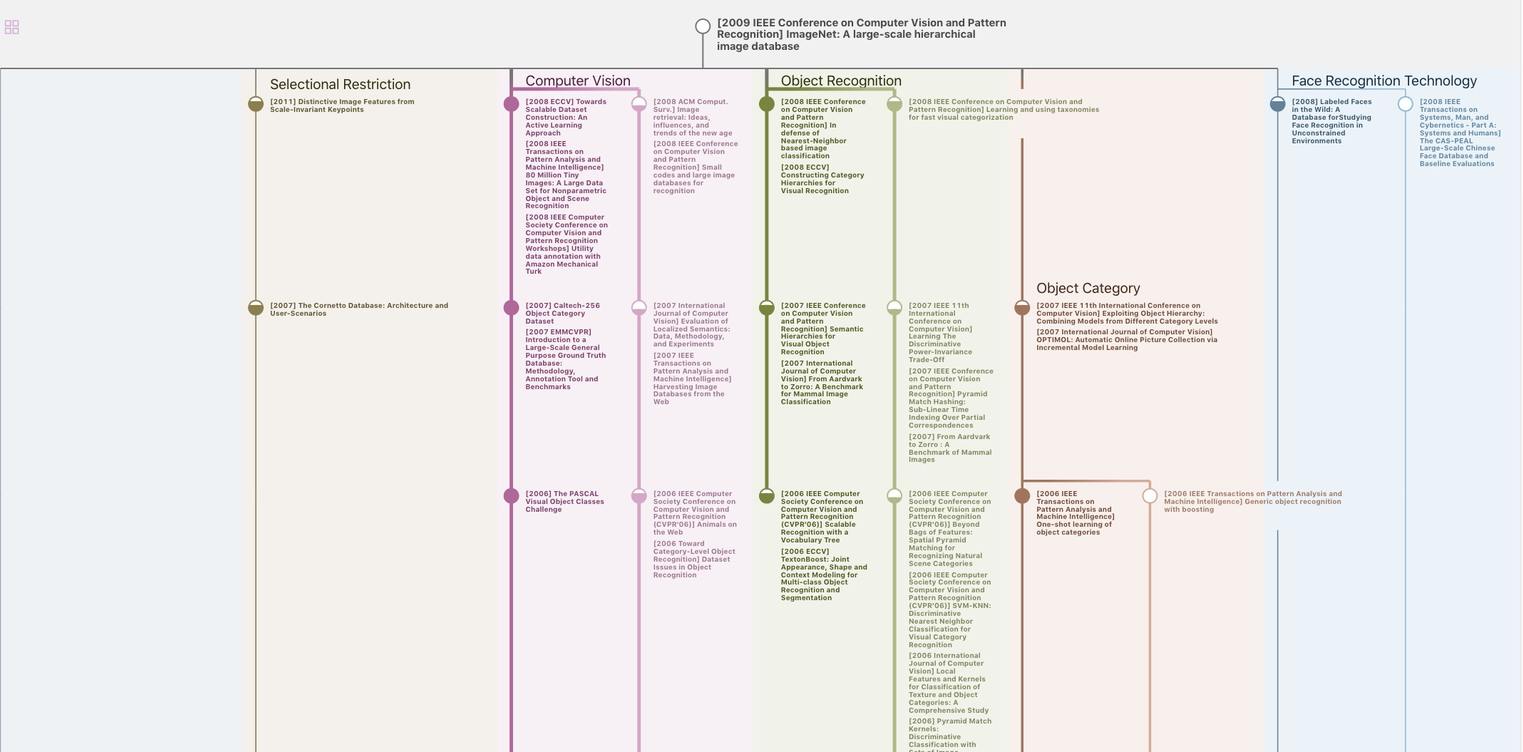
生成溯源树,研究论文发展脉络
Chat Paper
正在生成论文摘要