Getting Personal with Epigenetics: Towards Machine-Learning-Assisted Precision Epigenomics
biorxiv(2022)
摘要
Epigenetic modifications are dynamic control mechanisms involved in the regulation of gene expression. Unlike the DNA sequence itself, they vary not only between individuals but also between different cell types of the same individual. Exposure to environmental factors, somatic mutations, and ageing contribute to epigenomic changes over time, which may constitute early hallmarks or causal factors of disease. Epigenetic changes are reversible and, therefore, promising therapeutic targets. However, mapping efforts to determine an individual's cell-type-specific epigenome are constrained by experimental costs. We developed eDICE, an attention-based deep learning model, to impute epigenomic tracks. eDICE achieves improved overall performance compared to previous models on the reference Roadmap epigenomes. Furthermore, we present a proof of concept for the imputation of personalised epigenomic measurements on the ENTEx dataset, where eDICE correctly predicts individual- and cell-type-specific epigenetic patterns. This case study constitutes an important step towards robustly employing machine-learning-based approaches for personalised epigenomics.
### Competing Interest Statement
The authors have declared no competing interest.
更多查看译文
关键词
epigenomics,epigenetics,machine-learning-assisted machine-learning-assisted
AI 理解论文
溯源树
样例
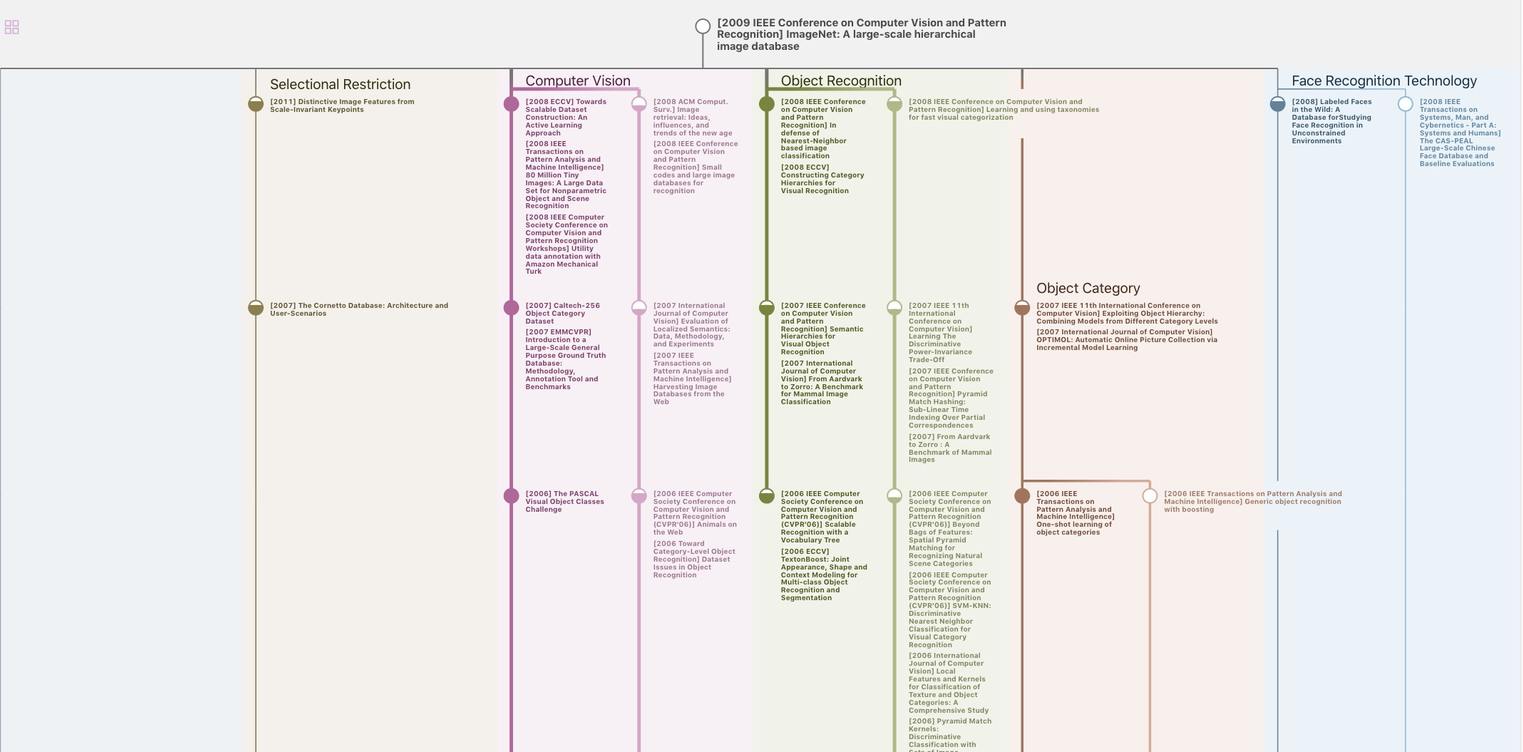
生成溯源树,研究论文发展脉络
Chat Paper
正在生成论文摘要