Adaptive Graph Convolutional Networks for Weakly Supervised Anomaly Detection in Videos.
IEEE Signal Process. Lett.(2022)
摘要
For weakly supervised anomaly detection, most existing work is limited to the problem of inadequate video representation due to the inability of modeling long-term contextual information. To solve this, we propose a novel weakly supervised adaptive graph convolutional network (WAGCN) to model the complex contextual relationship among video segments. By which, we fully consider the influence of other video segments on the current one when generating the anomaly probability score for each segment. Firstly, we combine the temporal consistency as well as feature similarity of video segments to construct a global graph, which makes full use of the association information among spatial-temporal features of anomalous events in videos. Secondly, we propose a graph learning layer in order to break the limitation of setting topology manually, which can extract graph adjacency matrix based on data adaptively and effectively. Extensive experiments on two public datasets (i.e., UCF-Crime dataset and ShanghaiTech dataset) demonstrate the effectiveness of our approach which achieves state-of-the-art performance.
更多查看译文
关键词
Anomaly detection,adaptive learning,graph convolutional networks,temporal modeling
AI 理解论文
溯源树
样例
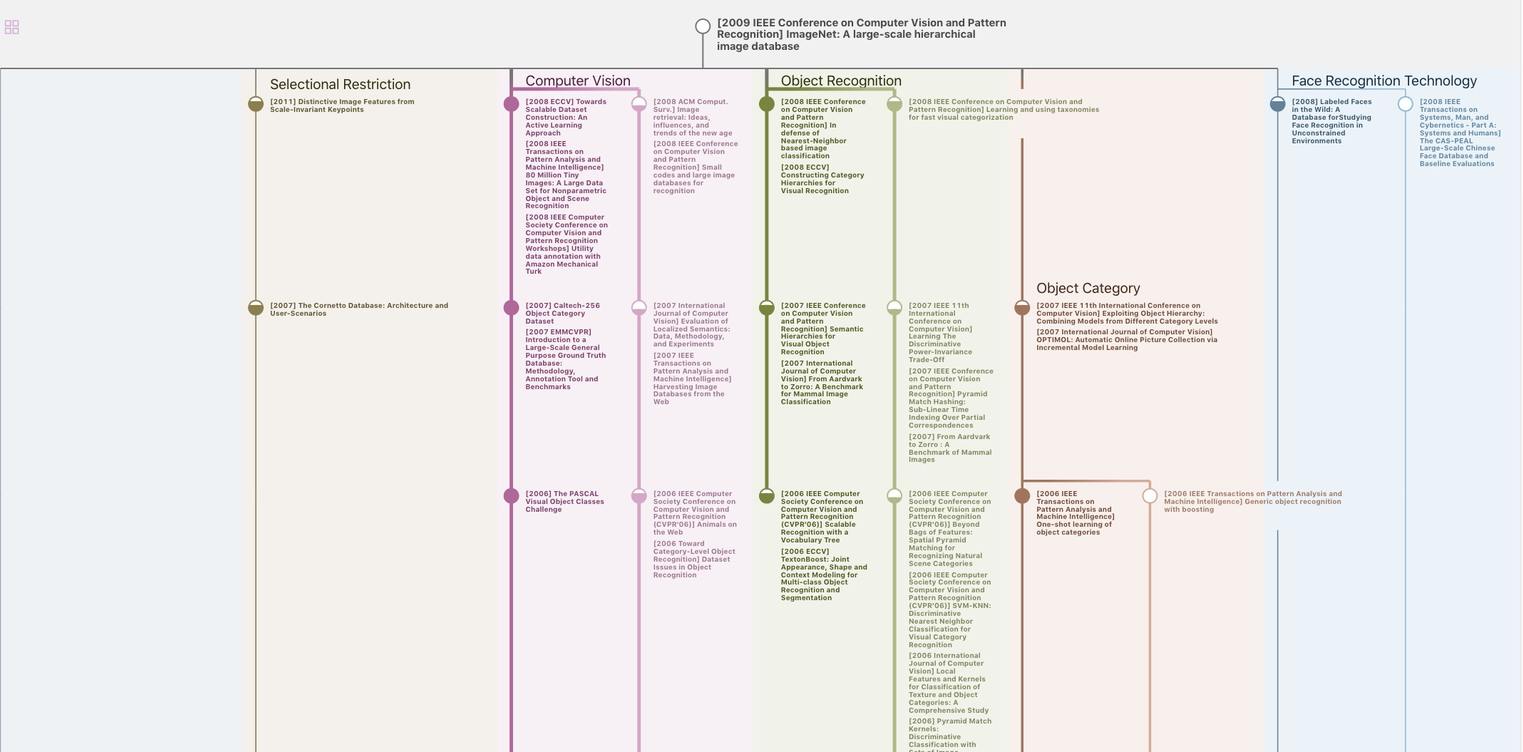
生成溯源树,研究论文发展脉络
Chat Paper
正在生成论文摘要