Nonlinear Optical Joint Transform Correlator for Low Latency Convolution Operations
arxiv(2022)
摘要
Convolutions are one of the most relevant operations in artificial intelligence (AI) systems. High computational complexity scaling poses significant challenges, especially in fast-responding network-edge AI applications. Fortunately, the convolution theorem can be executed on-the-fly in the optical domain via a joint transform correlator (JTC) offering to fundamentally reduce the computational complexity. Nonetheless, the iterative two-step process of a classical JTC renders them unpractical. Here we introduce a novel implementation of an optical convolution-processor capable of near-zero latency by utilizing all-optical nonlinearity inside a JTC, thus minimizing electronic signal or conversion delay. Fundamentally we show how this nonlinear auto-correlator enables reducing the high $O(n^4)$ scaling complexity of processing two-dimensional data to only $O(n^2)$. Moreover, this optical JTC processes millions of channels in time-parallel, ideal for large-matrix machine learning tasks. Exemplary utilizing the nonlinear process of four-wave mixing, we show light processing performing a full convolution that is temporally limited only by geometric features of the lens and the nonlinear material's response time. We further discuss that the all-optical nonlinearity exhibits gain in excess of $>10^{3}$ when enhanced by slow-light effects such as epsilon-near-zero. Such novel implementation for a machine learning accelerator featuring low-latency and non-iterative massive data parallelism enabled by fundamental reduced complexity scaling bears significant promise for network-edge, and cloud AI systems.
更多查看译文
关键词
low latency convolution
AI 理解论文
溯源树
样例
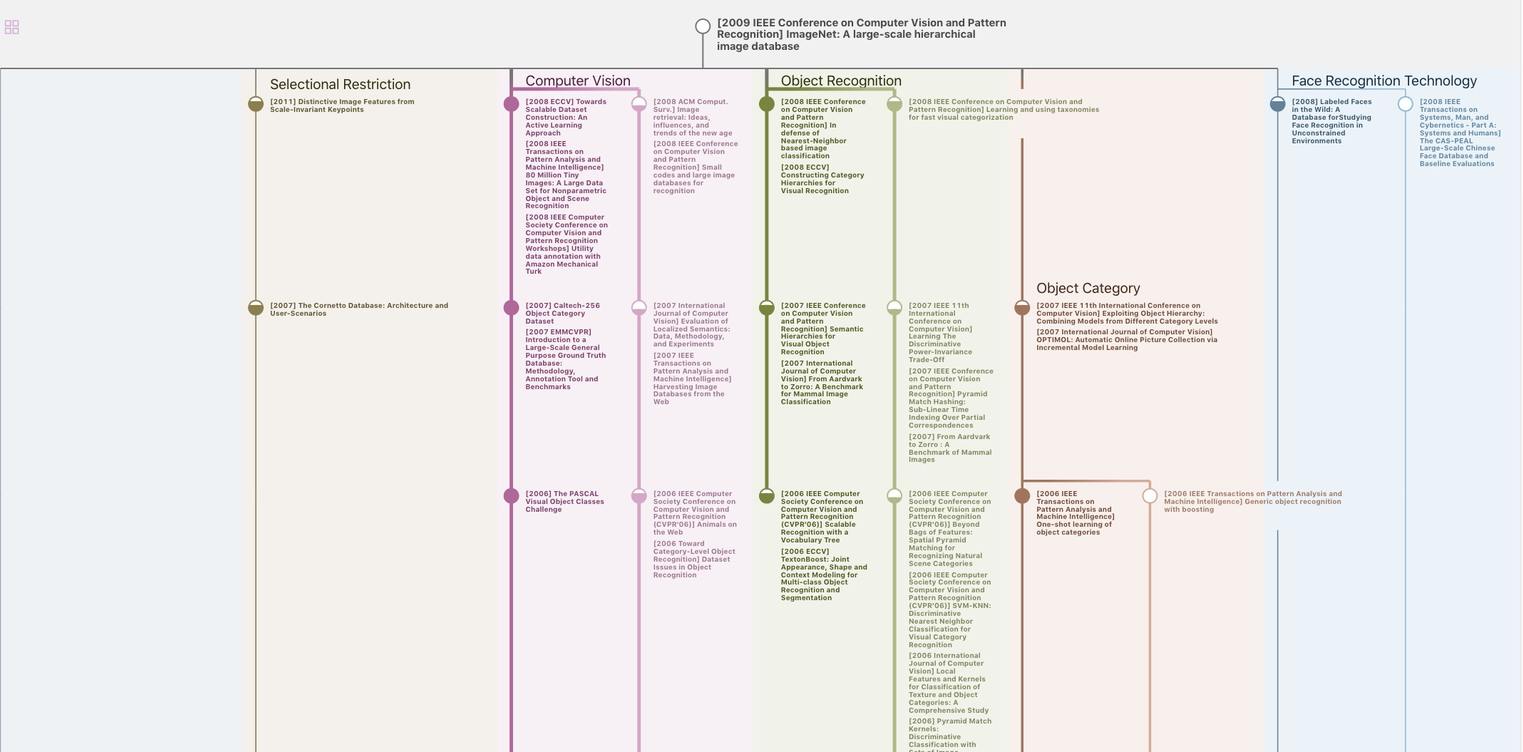
生成溯源树,研究论文发展脉络
Chat Paper
正在生成论文摘要