Low-light Image Enhancement by Retinex Based Algorithm Unrolling and Adjustment
arxiv(2022)
摘要
Motivated by their recent advances, deep learning techniques have been widely applied to low-light image enhancement (LIE) problem. Among which, Retinex theory based ones, mostly following a decomposition-adjustment pipeline, have taken an important place due to its physical interpretation and promising performance. However, current investigations on Retinex based deep learning are still not sufficient, ignoring many useful experiences from traditional methods. Besides, the adjustment step is either performed with simple image processing techniques, or by complicated networks, both of which are unsatisfactory in practice. To address these issues, we propose a new deep learning framework for the LIE problem. The proposed framework contains a decomposition network inspired by algorithm unrolling, and adjustment networks considering both global brightness and local brightness sensitivity. By virtue of algorithm unrolling, both implicit priors learned from data and explicit priors borrowed from traditional methods can be embedded in the network, facilitate to better decomposition. Meanwhile, the consideration of global and local brightness can guide designing simple yet effective network modules for adjustment. Besides, to avoid manually parameter tuning, we also propose a self-supervised fine-tuning strategy, which can always guarantee a promising performance. Experiments on a series of typical LIE datasets demonstrated the effectiveness of the proposed method, both quantitatively and visually, as compared with existing methods.
更多查看译文
关键词
Algorithm unrolling, deep learning, low-light image enhancement (LIE), Retinex theory
AI 理解论文
溯源树
样例
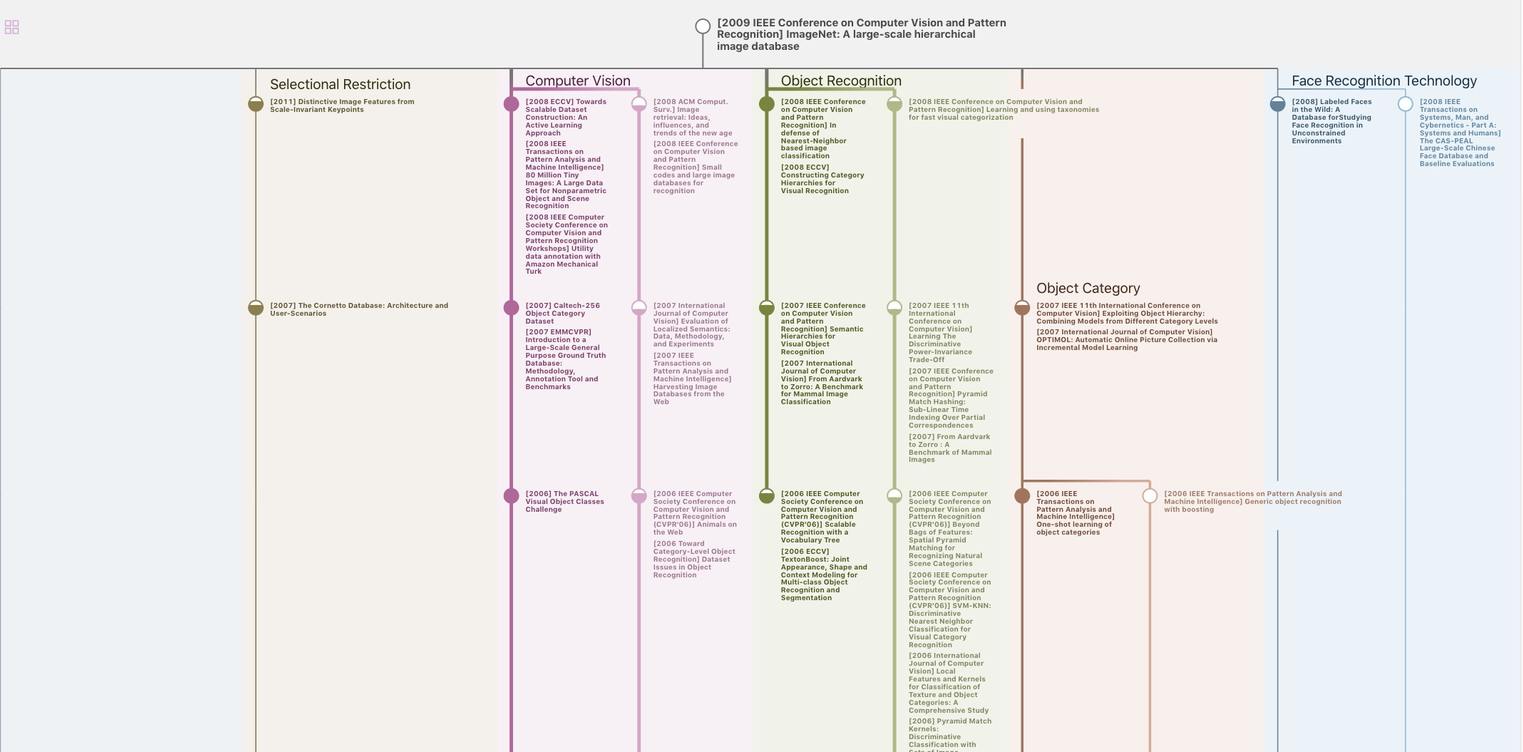
生成溯源树,研究论文发展脉络
Chat Paper
正在生成论文摘要