Audio-Based Deep Learning Frameworks for Detecting COVID-19.
European Signal Processing Conference (EUSIPCO)(2022)
摘要
This paper evaluates a wide range of audio-based deep learning frameworks applied to the breathing, cough, and speech sounds for detecting COVID-19. In general, the audio recording inputs are transformed into low-level spectrogram features, then they are fed into pre-trained deep learning models to extract high-level embedding features. Next, the dimension of these high-level embedding features are reduced before finetuning using Light Gradient Boosting Machine (LightGBM) as a back-end classification. Our experiments on the Second DiCOVA Challenge achieved the highest Area Under the Curve (AUC), F1 score, sensitivity score, and specificity score of 89.03%, 64.41%, 63.33%, and 95.13%, respectively. Based on these scores, our method outperforms the state-of-the-art systems, and improves the challenge baseline by 4.33%, 6.00% and 8.33% in terms of AUC, F1 score and sensitivity score, respectively.
更多查看译文
关键词
deep learning frameworks,audio-based
AI 理解论文
溯源树
样例
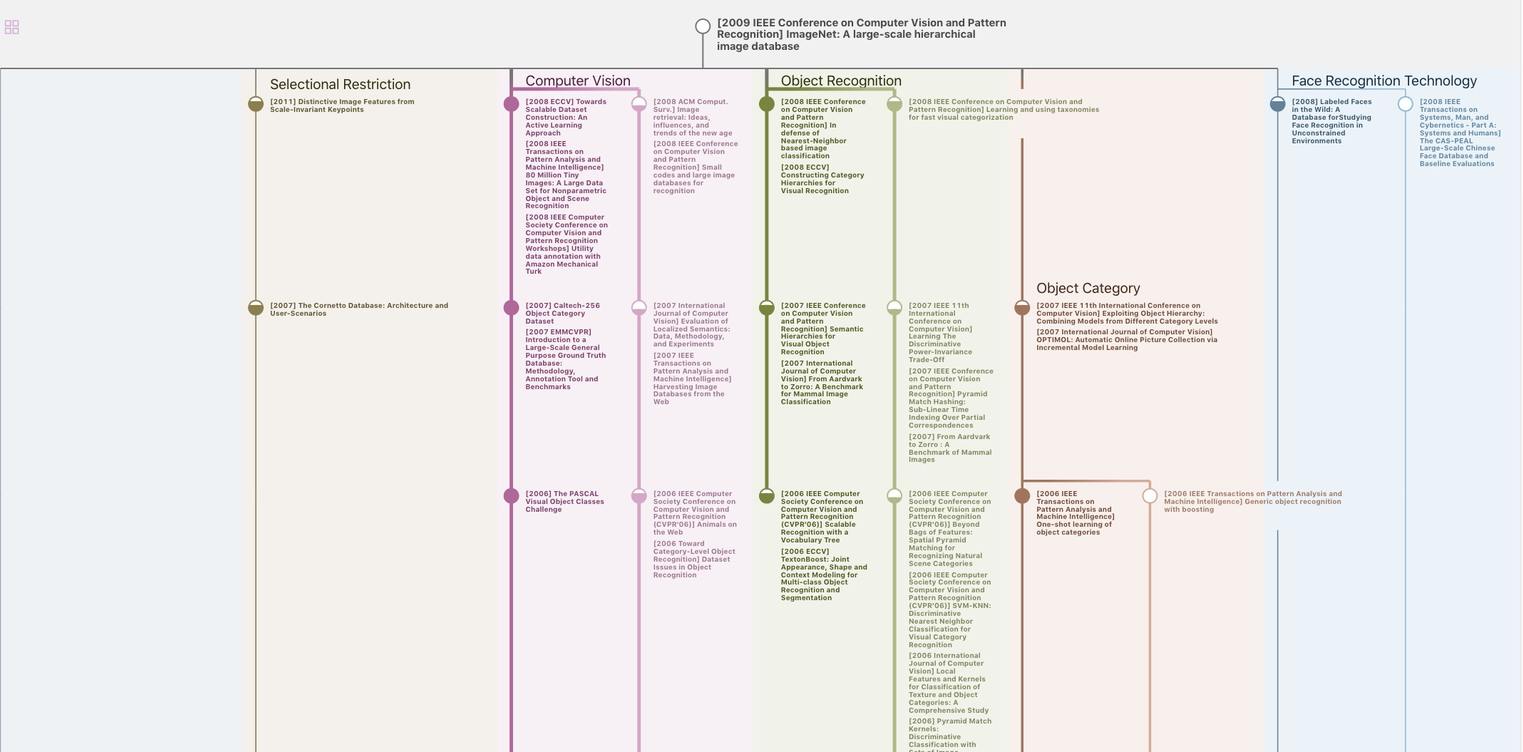
生成溯源树,研究论文发展脉络
Chat Paper
正在生成论文摘要