Measuring dissimilarity with diffeomorphism invariance.
International Conference on Machine Learning(2022)
摘要
Measures of similarity (or dissimilarity) are a key ingredient to many machine learning algorithms. We introduce DID, a pairwise dissimilarity measure applicable to a wide range of data spaces, which leverages the data’s internal structure to be invariant to diffeomorphisms. We prove that DID enjoys properties which make it relevant for theoretical study and practical use. By representing each datum as a function, DID is defined as the solution to an optimization problem in a Reproducing Kernel Hilbert Space and can be expressed in closed-form. In practice, it can be efficiently approximated via Nystr{ö}m sampling. Empirical experiments support the merits of DID.
更多查看译文
关键词
diffeomorphism invariance,dissimilarity
AI 理解论文
溯源树
样例
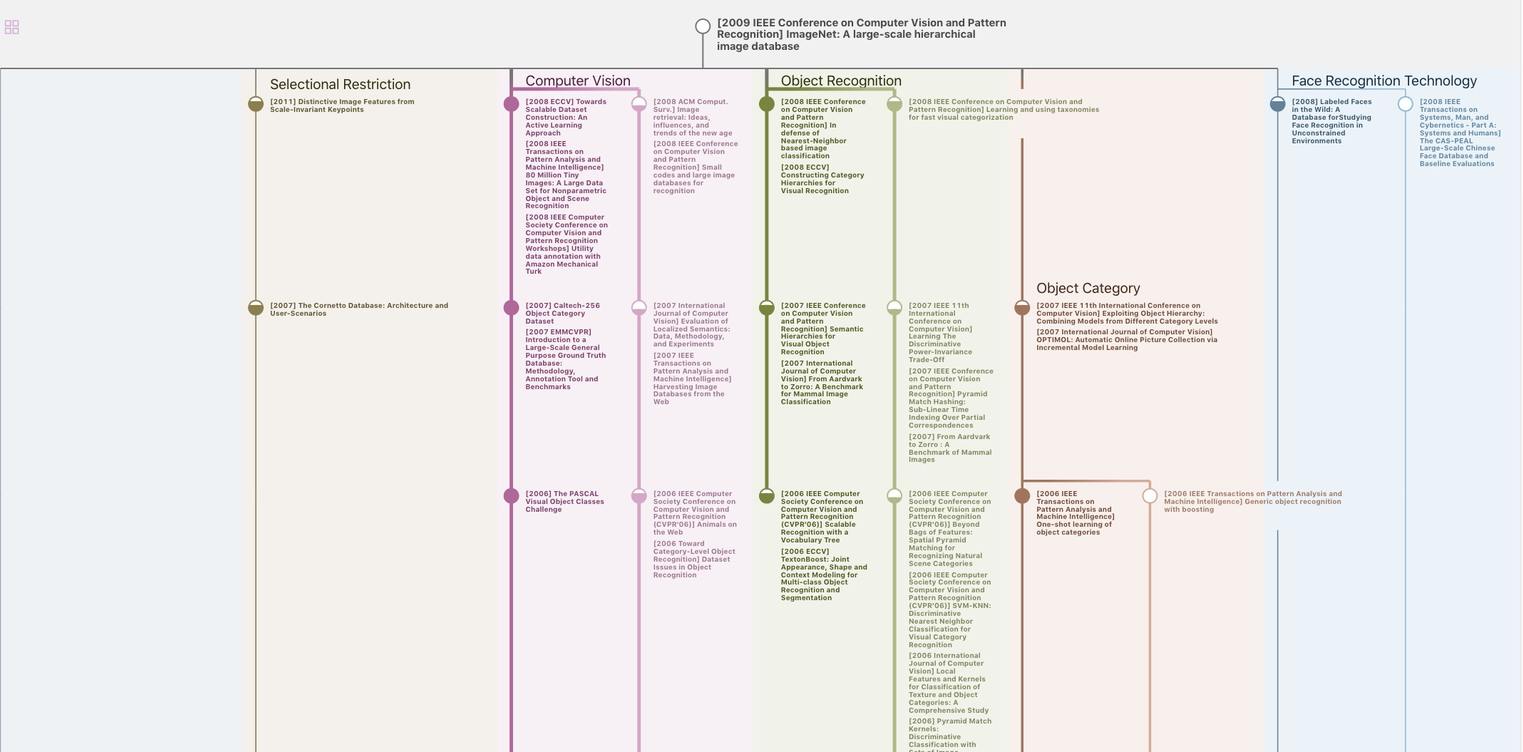
生成溯源树,研究论文发展脉络
Chat Paper
正在生成论文摘要