Domain Adversarial Training: A Game Perspective
International Conference on Learning Representations (ICLR)(2022)
摘要
The dominant line of work in domain adaptation has focused on learning invariant representations using domain-adversarial training. In this paper, we interpret this approach from a game theoretical perspective. Defining optimal solutions in domain-adversarial training as a local Nash equilibrium, we show that gradient descent in domain-adversarial training can violate the asymptotic convergence guarantees of the optimizer, oftentimes hindering the transfer performance. Our analysis leads us to replace gradient descent with high-order ODE solvers (i.e., Runge-Kutta), for which we derive asymptotic convergence guarantees. This family of optimizers is significantly more stable and allows more aggressive learning rates, leading to high performance gains when used as a drop-in replacement over standard optimizers. Our experiments show that in conjunction with state-of-the-art domain-adversarial methods, we achieve up to 3.5% improvement with less than of half training iterations. Our optimizers are easy to implement, free of additional parameters, and can be plugged into any domain-adversarial framework.
更多查看译文
关键词
Domain Adversarial Training,Domain Adaptation,Neural Networks Optimization,Game Theory
AI 理解论文
溯源树
样例
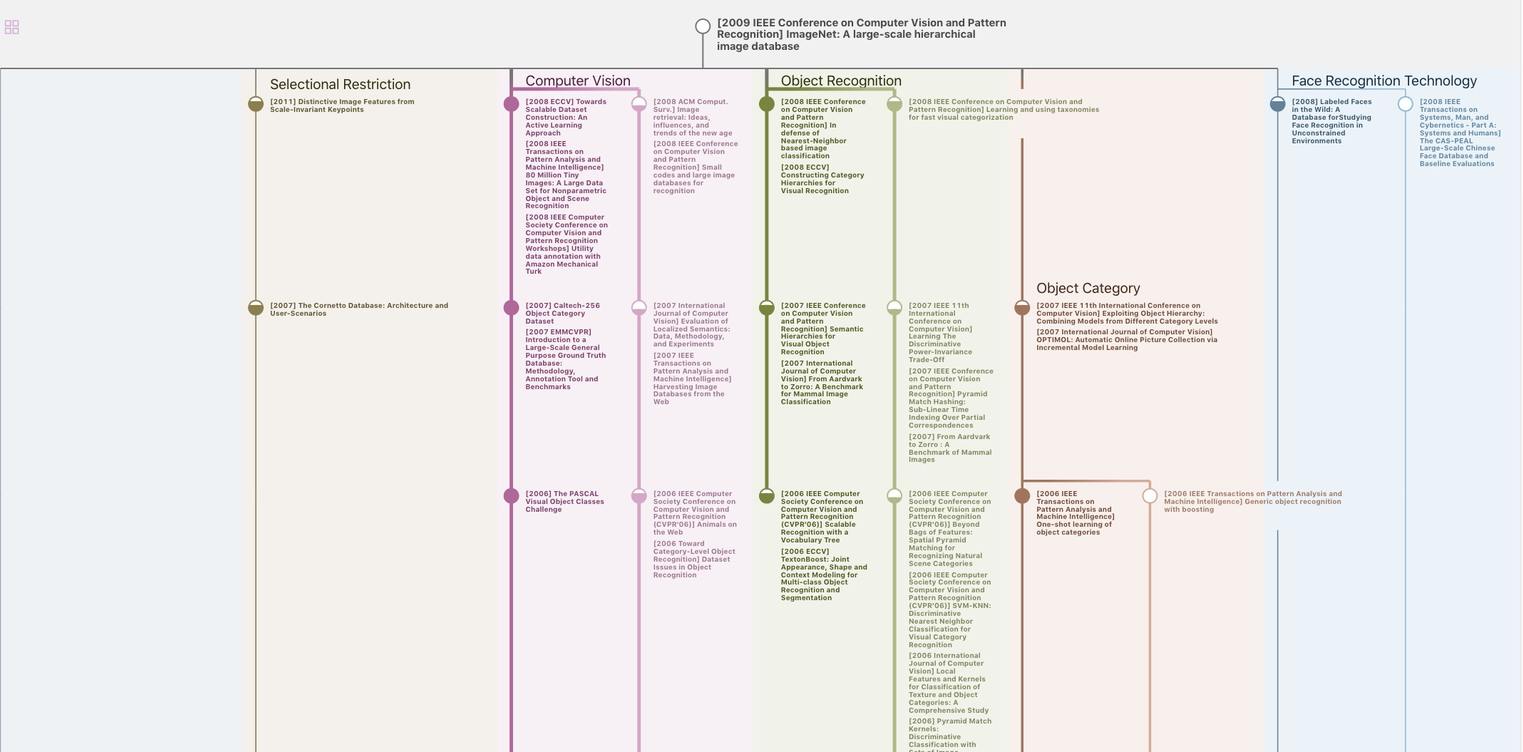
生成溯源树,研究论文发展脉络
Chat Paper
正在生成论文摘要