AI-Based Resource Allocation in End-to-End Network Slicing Under Demand and CSI Uncertainties
IEEE TRANSACTIONS ON NETWORK AND SERVICE MANAGEMENT(2023)
摘要
Network slicing (NwS) is one of the main technologies in the fifth-generation of mobile communication and beyond (5G+). One of the important challenges in the NwS is information uncertainty which mainly involves demand and channel state information (CSI). Demand uncertainty is divided into three types: number of users requests, amount of bandwidth, and requested virtual network functions workloads. Moreover, the CSI uncertainty is modeled by three methods: worst-case, probabilistic, and hybrid. In this paper, our goal is to maximize the utility of the infrastructure provider by exploiting deep reinforcement learning (DRL) algorithms in end-to-end NwS resource allocation under demand and CSI uncertainties. Enhanced mobile broadband (eMBB) requires high data rates. The uncertainties we argued above have a direct negative impact on the data rate and our objective function. Therefore, we focus primarily on eMBB. Additionally, we also consider ultra-reliable low latency communications (uRLLC) and massive machine-type communication (mMTC). The proposed formulation is a non-convex mixed-integer non-linear programming problem. To perform resource allocation in problems that involve uncertainty, we need a history of previous information. To this end, we use a recurrent deterministic policy gradient (RDPG) algorithm, a recurrent and memory-based approach in DRL. Then, we compare the RDPG method in different scenarios with soft actor-critic (SAC), deep deterministic policy gradient (DDPG), distributed, and greedy algorithms. The simulation results show that the SAC method is better than the DDPG, distributed, and greedy methods, respectively. Moreover, the RDPG method out performs the SAC approach on average by 70%.
更多查看译文
关键词
End-to-end network slicing,resource allocation,software-defined networking (SDN),network function virtualization (NFV),demand uncertainty,channel state information (CSI) uncertainty,recurrent deterministic policy gradient (RDPG)
AI 理解论文
溯源树
样例
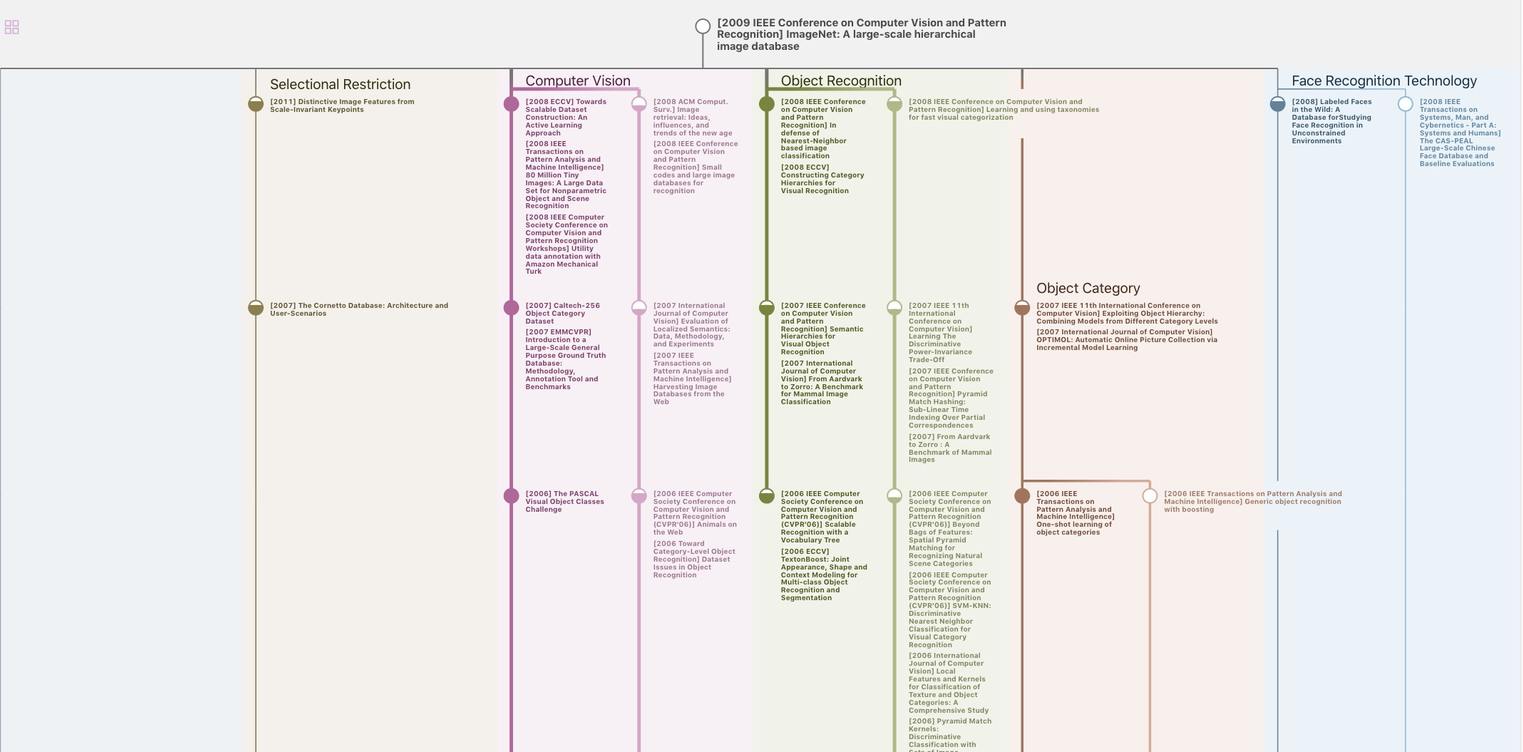
生成溯源树,研究论文发展脉络
Chat Paper
正在生成论文摘要